Artificial intelligence for automated detection of large mammals creates path to upscale drone surveys
Scientific reports(2023)
摘要
Imagery from drones is becoming common in wildlife research and management, but processing data efficiently remains a challenge. We developed a methodology for training a convolutional neural network model on large-scale mosaic imagery to detect and count caribou ( Rangifer tarandus ), compare model performance with an experienced observer and a group of naïve observers, and discuss the use of aerial imagery and automated methods for large mammal surveys. Combining images taken at 75 m and 120 m above ground level, a faster region-based convolutional neural network (Faster-RCNN) model was trained in using annotated imagery with the labels: “ adult caribou ”, “ calf caribou ”, and “ ghost caribou ” (animals moving between images, producing blurring individuals during the photogrammetry processing). Accuracy, precision, and recall of the model were 80%, 90%, and 88%, respectively. Detections between the model and experienced observer were highly correlated (Pearson: 0.96–0.99, P value < 0.05). The model was generally more effective in detecting adults, calves, and ghosts than naïve observers at both altitudes. We also discuss the need to improve consistency of observers’ annotations if manual review will be used to train models accurately. Generalization of automated methods for large mammal detections will be necessary for large-scale studies with diverse platforms, airspace restrictions, and sensor capabilities.
更多查看译文
关键词
large mammals,artificial intelligence,detection
AI 理解论文
溯源树
样例
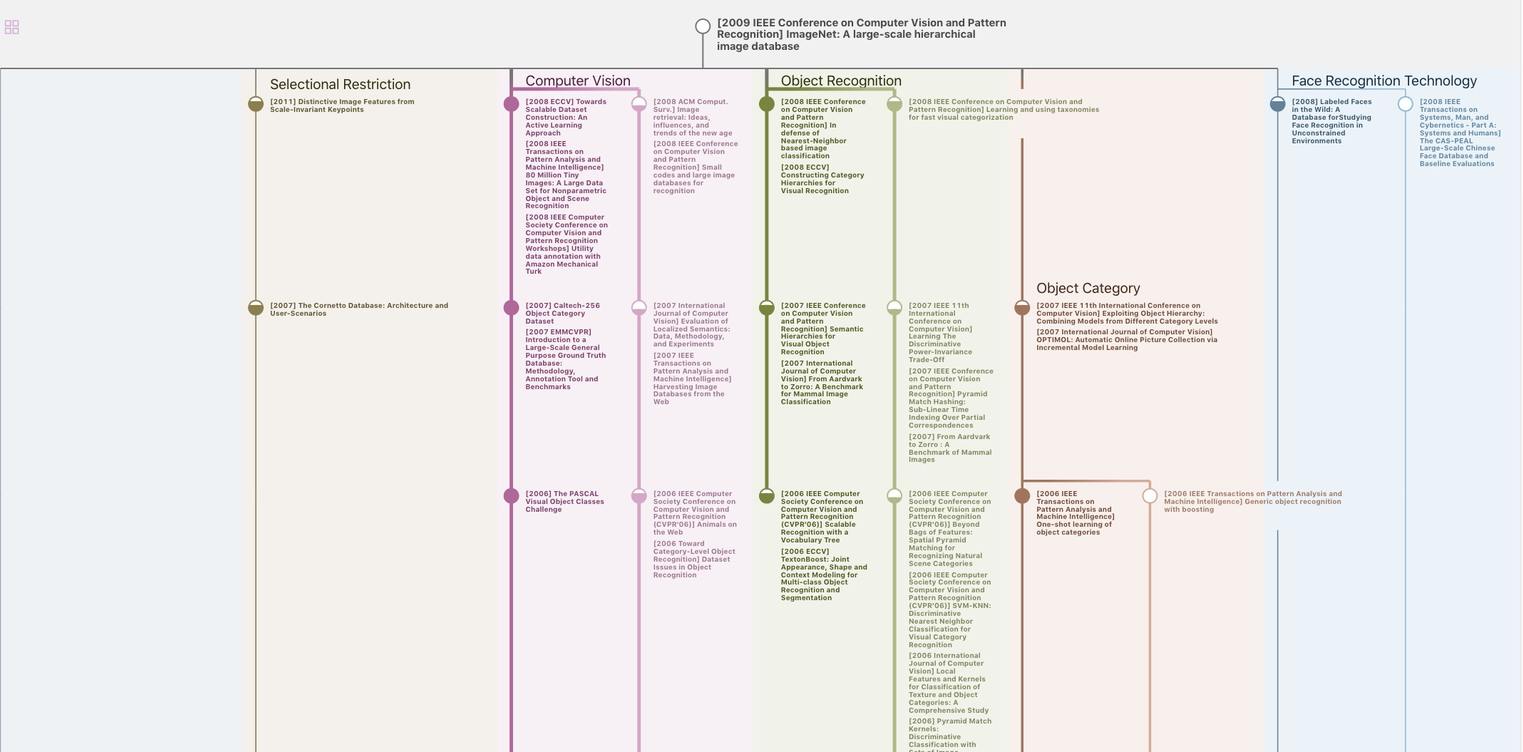
生成溯源树,研究论文发展脉络
Chat Paper
正在生成论文摘要