CT synthesis from MRI with an improved multi-scale learning network
Frontiers in Physics(2023)
摘要
Introduction: Using MRI to synthesize CT and substitute its function in radiation therapy has drawn wide research interests. Currently, deep learning models have become the first choice for MRI—CT synthesis because of their ability to study complex non-linear relations. However, existing studies still lack the ability to learn complex local and global MRI–CT relations in the same time , which influences the intensity and structural performance of synthetic images.Methods: This study proposes a hybrid multi-scale model to explore rich local and global MRI—CT relations, relations, namely, the hybrid multi-scale synthesis network (HMSS-Net). It includes two modules modelling different resolution inputs. In the low-resolution module, the Transformer method is applied to build its bottleneck part to expand the receptive field and explore long-range MRI—CT relations to estimate the coarse distribution of widely spread tissues and large organs. In the high-resolution module, residual and dense connections are applied to explore complex local MRI—CT relations under multiple step sizes. Then, the feature spaces of two modules are combined together and utilized to provide synthetic CT. HMSS-Net also introduces the multi-scale structural similarity index measure loss to provide multi-scale supervision during training.Results: The experimental results on head and neck regions of 78 patients showed that HMSS–Net reduced the average of 7.6/3.13 HU on the mean absolute error and increased the average of 2.1/1.8% on the dice coefficient of bone compared with competing image–to–image synthesis methods.Conclusion: The results imply that HMSS–Net could effectively improve the intensity and structural performances of synthetic CT.
更多查看译文
关键词
MRI–CT synthesis,multi-scale learning,deep learning,transformer,hybrid model,convolution
AI 理解论文
溯源树
样例
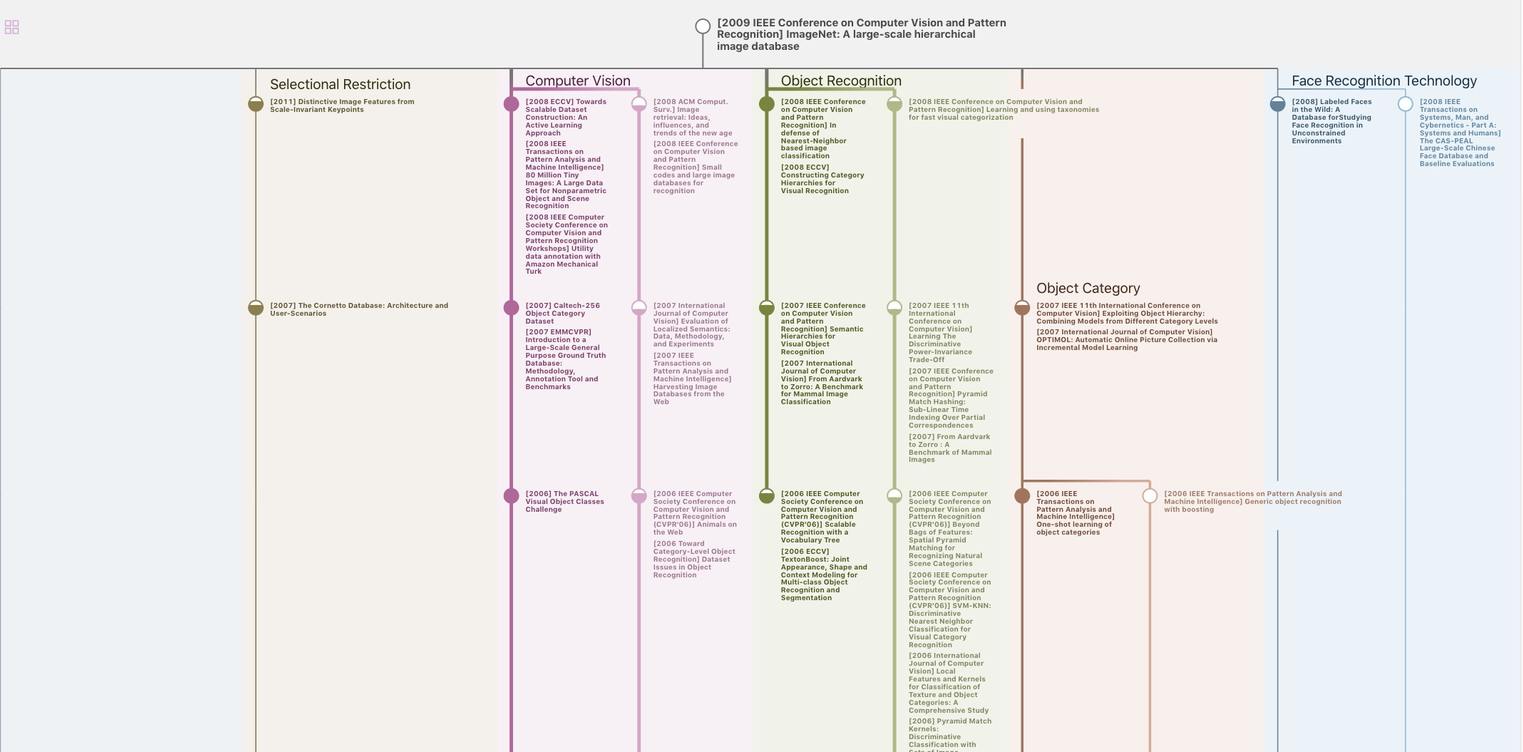
生成溯源树,研究论文发展脉络
Chat Paper
正在生成论文摘要