Closed-loop automatic gradient design for liquid chromatography using Bayesian optimization.
Analytica chimica acta(2023)
摘要
Contemporary complex samples require sophisticated methods for full analysis. This work describes the development of a Bayesian optimization algorithm for automated and unsupervised development of gradient programs. The algorithm was tailored to LC using a Gaussian process model with a novel covariance kernel. To facilitate unsupervised learning, the algorithm was designed to interface directly with the chromatographic system. Single-objective and multi-objective Bayesian optimization strategies were investigated for the separation of two complex (n>18, and n>80) dye mixtures. Both approaches found satisfactory optima in under 35 measurements. The multi-objective strategy was found to be powerful and flexible in terms of exploring the Pareto front. The performance difference between the single-objective and multi-objective strategy was further investigated using a retention modeling example. One additional advantage of the multi-objective approach was that it allows for a trade-off to be made between multiple objectives without prior knowledge. In general, the Bayesian optimization strategy was found to be particularly suitable, but not limited to, cases where retention modelling is not possible, although its scalability might be limited in terms of the number of parameters that can be simultaneously optimized.
更多查看译文
关键词
liquid chromatography,automatic gradient design,optimization,closed-loop
AI 理解论文
溯源树
样例
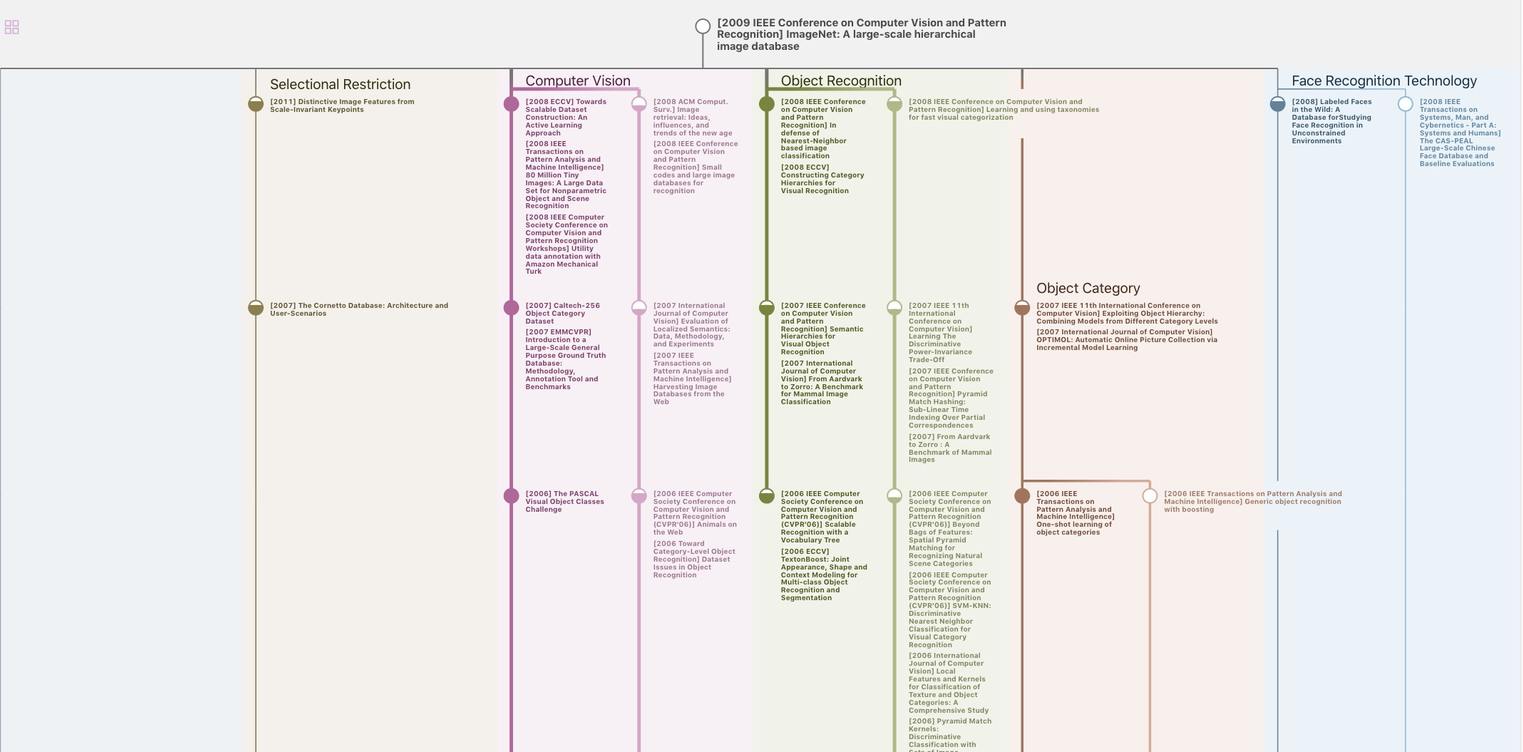
生成溯源树,研究论文发展脉络
Chat Paper
正在生成论文摘要