Green Simulation Based Policy Optimization with Partial Historical Trajectory Reuse
2022 Winter Simulation Conference (WSC)(2022)
摘要
Built on our previous study on green simulation assisted policy gradient (GS-PG), in this paper, we consider infinite-horizon Markov decision processes and create a new importance sampling based policy gradient optimization approach to support dynamic decision making. The existing GS-PG method was designed to learn from complete episodes or process trajectories, which limits its applicability to low-data situations and flexible online process control. To overcome this limitation, the proposed approach utilizes a mixture likelihood ratio (MLR) based policy gradient and intelligently select and reuse the most related historical transition samples to improve the policy gradient estimation and accelerate the learning of optimal policy. Our empirical study demonstrates that it can improve optimization convergence and enhance the performance of state-of-the-art policy optimization approaches such as actor-critic method and proximal policy optimizations.
更多查看译文
AI 理解论文
溯源树
样例
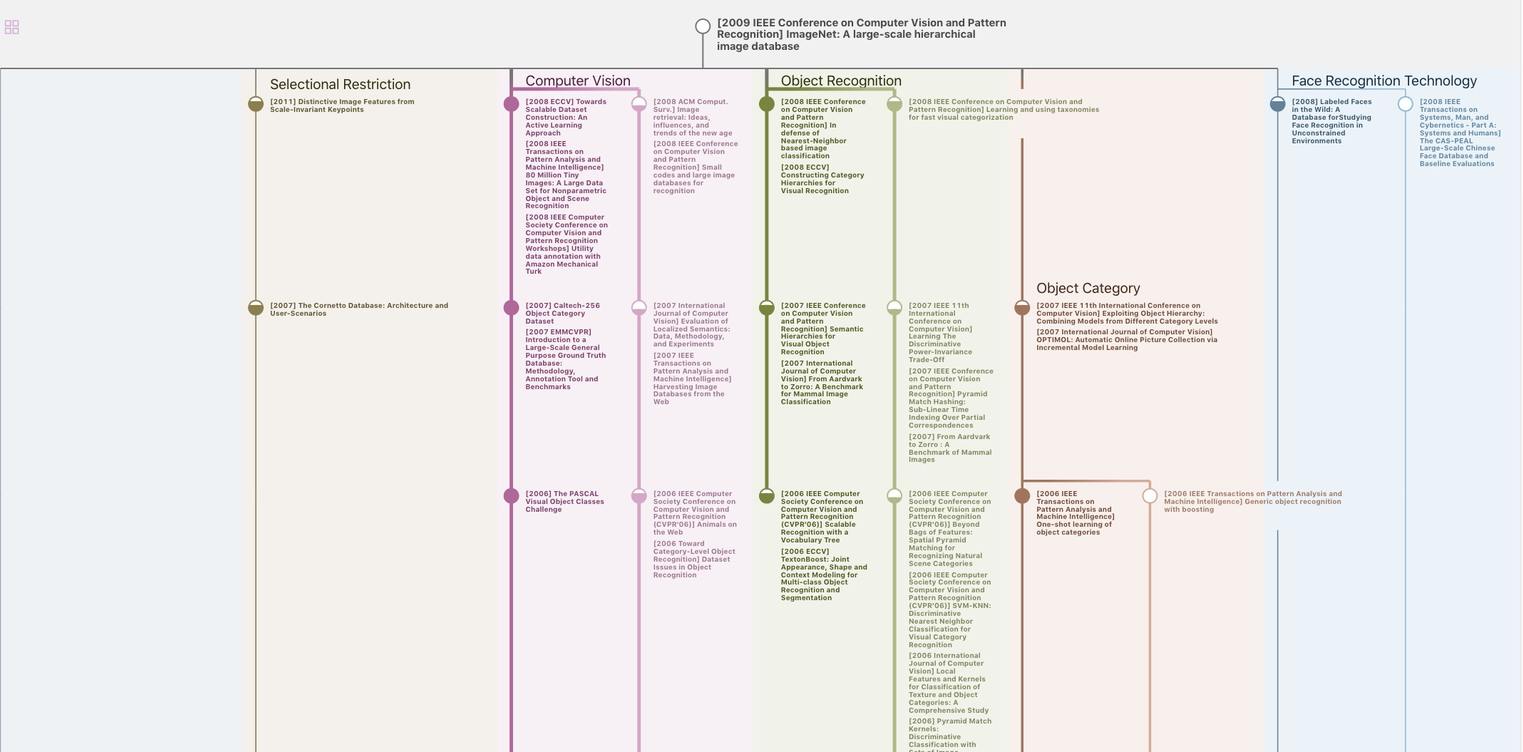
生成溯源树,研究论文发展脉络
Chat Paper
正在生成论文摘要