Interpretable artificial neural networks for retrospective QbD of pharmaceutical tablet manufacturing based on a pilot-scale developmental dataset
International Journal of Pharmaceutics(2023)
摘要
As the pharmaceutical industry increasingly adopts the Pharma 4.0. concept, there is a growing need to effec-tively predict the product quality based on manufacturing or in-process data. Although artificial neural networks (ANNs) have emerged as powerful tools in data-rich environments, their implementation in pharmaceutical manufacturing is hindered by their black-box nature. In this work, ANNs were developed and interpreted to demonstrate their applicability to increase process understanding by retrospective analysis of developmental or manufacturing data. The in vitro dissolution and hardness of extended-release, directly compressed tablets were predicted from manufacturing and spectroscopic data of pilot-scale development. The ANNs using material at-tributes and operational parameters provided better results than using NIR or Raman spectra as predictors. ANNs were interpreted by sensitivity analysis, helping to identify the root cause of the batch-to-batch variability, e.g., the variability in particle size, grade, or substitution of the hydroxypropyl methylcellulose excipient. An ANN-based control strategy was also successfully utilized to mitigate the batch-to-batch variability by flexibly oper-ating the tableting process. The presented methodology can be adapted to arbitrary data-rich manufacturing steps from active substance synthesis to formulation to predict the quality from manufacturing or development data and gain process understanding and consistent product quality.
更多查看译文
关键词
Interpretable artificial neural network,Explainable artificial intelligence,Retrospective quality by design,Pharma 4,0,Real-time release testing,Dissolution prediction
AI 理解论文
溯源树
样例
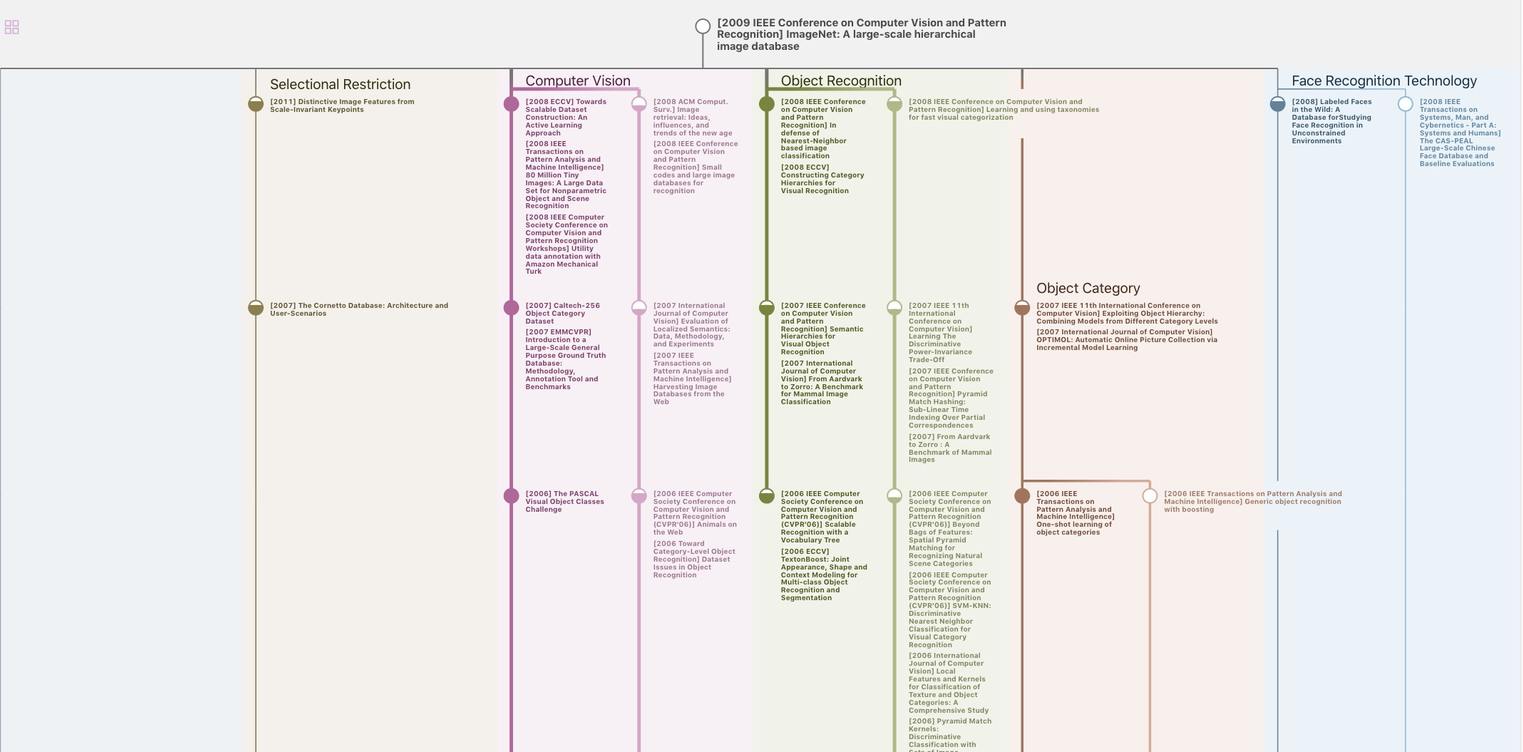
生成溯源树,研究论文发展脉络
Chat Paper
正在生成论文摘要