A Two-branch Symmetric Domain Adaptation Neural Network Based on Ulam Stability Theory
Information Sciences(2023)
摘要
Unsupervised domain adaptation is an important branch of transfer learning, which mainly solves the generalization problem of models from fully labeled datasets to unlabeled datasets. Unsu-pervised domain adaptation mainly aims to make full use of domain features, suppress inter domain distribution shift, and improve the domain generalization ability of the model. In this paper, we propose a two-branch symmetric domain adaptation neural network. In each inde-pendent domain adaptation branch, we use the method of adversarial learning to realize the embedding and alignment of domain features, and use a new generalized autoencoder model based on Ulam stability theory to enhance the feature extraction ability of the network. A fusion network is adapted to fuse the features extracted from each branch of the feature subspace to further improve the domain adaptability and generalization ability of the model. Experimental results show the effectiveness of the proposed method.
更多查看译文
关键词
Unsupervised domain adaptation,Two -branch architecture,Generalized autoencoder,Ulam stability,Feature fusion
AI 理解论文
溯源树
样例
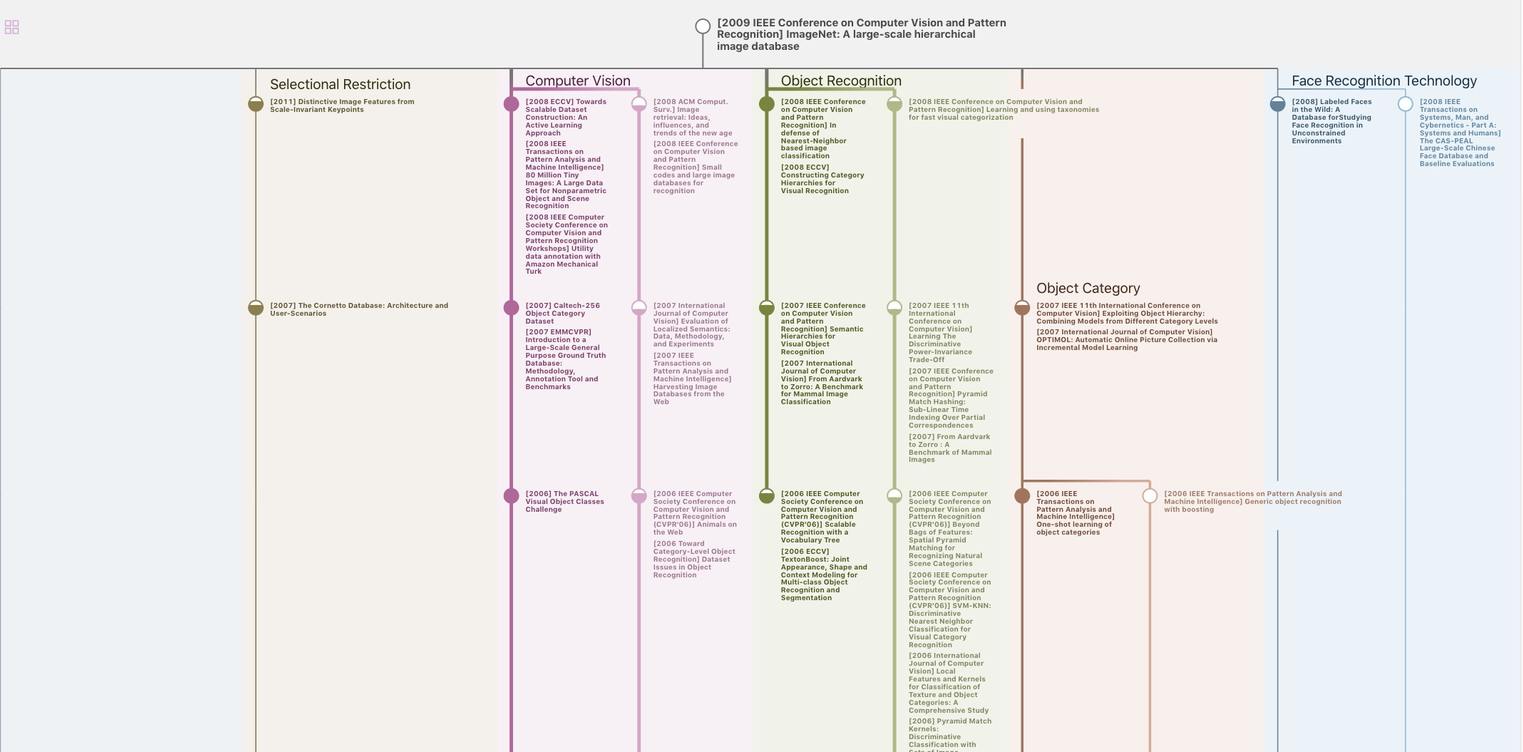
生成溯源树,研究论文发展脉络
Chat Paper
正在生成论文摘要