Randomized Learning-Based Classification of Sound Quality Using Spectrogram Image and Time-Series Data: A Practical Perspective
Engineering applications of artificial intelligence(2023)
摘要
Audio classification is an important research topic in the deep learning field, and classification accuracy has developed exponentially. However, as the model performance increases, it becomes difficult to apply it to actual industrial data owing to limitations such as the requirement of a large amount of data or increased training time. In this study, we developed a method for a small dataset with ambiguous classification boundaries, similar to most industrial data. We used randomized learning methods to reduce the training time and the risk of overfitting that required low computing time and few hyperparameters. Vehicle interior noise data were used as industrial data. The data were classified into luxury, powerful, and sporty, according to the human emotional reactions. Data was recorded from approximately 300 vehicles in the same environment and preprocessed into two types: spectrogram images and time-series data. Through deep fuzzy c-means clustering, it was confirmed that the classification boundary of the vehicle interior noise data was ambiguous. We selected the random vector functional link (RVFL), deep RVFL, and random kernel transformation (ROCKET) as randomized learning methods and compared the results with convolutional neural network and long short term memory. RVFL and deep RVFL showed similar classification accuracy to deep learning but significantly reduced training time by approximately 99%. In the case of ROCKET, the training time was reduced by approximately 91% and the classification performance was improved by approximately 3%. In addition, all randomized learning methods have few hyperparameters, which significantly reduces the classification model design time.
更多查看译文
关键词
Randomized learning,Random vector functional link,Random convolutional kernel transform,Time-series classification,Spectrogram classification
AI 理解论文
溯源树
样例
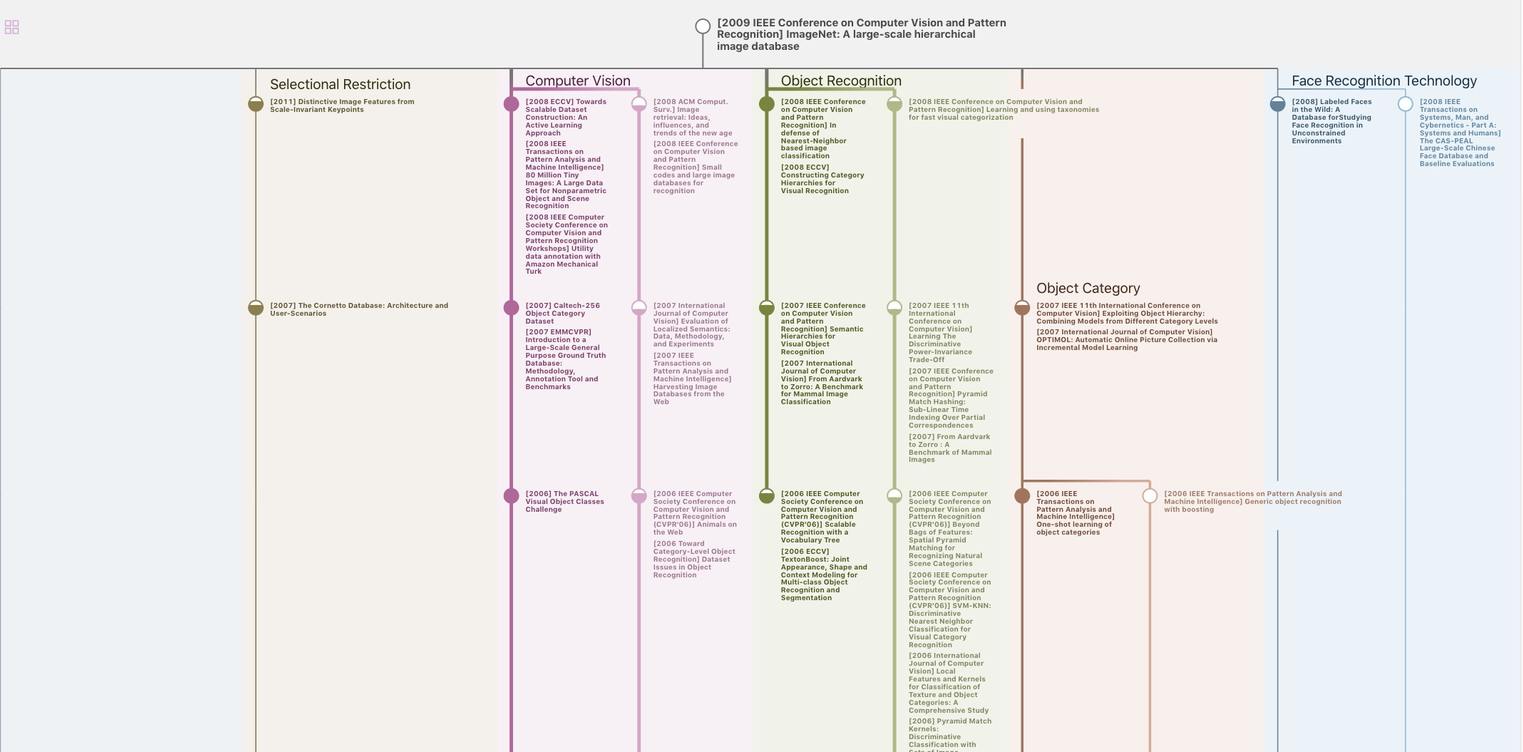
生成溯源树,研究论文发展脉络
Chat Paper
正在生成论文摘要