Automated Generation of Human-readable Natural Arabic Text from RDF Data
ACM Transactions on Asian and Low-Resource Language Information Processing(2023)
摘要
With the advances in Natural Language Processing (NLP), the industry has been moving towards human-directed artificial intelligence (AI) solutions. Recently, chatbots and automated news generation have captured a lot of attention. The goal is to automatically generate readable text from tabular data or web data commonly represented in Resource Description Framework (RDF) format. The problem can then be formulated as Data-to-text (D2T) generation from structured non-linguistic data into human-readable natural language. Despite the significant work done for the English language, no efforts are being directed towards low-resource languages like the Arabic language. This work promotes the development of the first RDF data-to-text (D2T) generation system for the Arabic language while trying to address the low-resource limitation. We develop several models for the Arabic D2T task using transfer learning from large language models (LLM) such as AraBERT, AraGPT2, and mT5. These models include a baseline Bi-LSTM Sequence-to-Sequence (Seq2Seq) model, as well as encoder-decoder transformers like BERT2BERT, BERT2GPT, and T5. We then provide a detailed comparative study highlighting the strengths and limitations of these methods setting the stage for further advancement in the field. We also introduce a new Arabic dataset (AraWebNLG) that can be used for new model development in the field. To ensure a comprehensive evaluation, general-purpose automated metrics (BLEU and Perplexity scores) are used as well as task-specific human evaluation metrics related to the accuracy of the content selection and fluency of the generated text. The results highlight the importance of pre-training on a large corpus of Arabic data and show that transfer learning from AraBERT gives the best performance. Text-to-text pre-training using mT5 achieves second best performance results even with multilingual weights.
更多查看译文
关键词
Low-resource languages,data-to-text,RDF,language models,neural networks,datasets
AI 理解论文
溯源树
样例
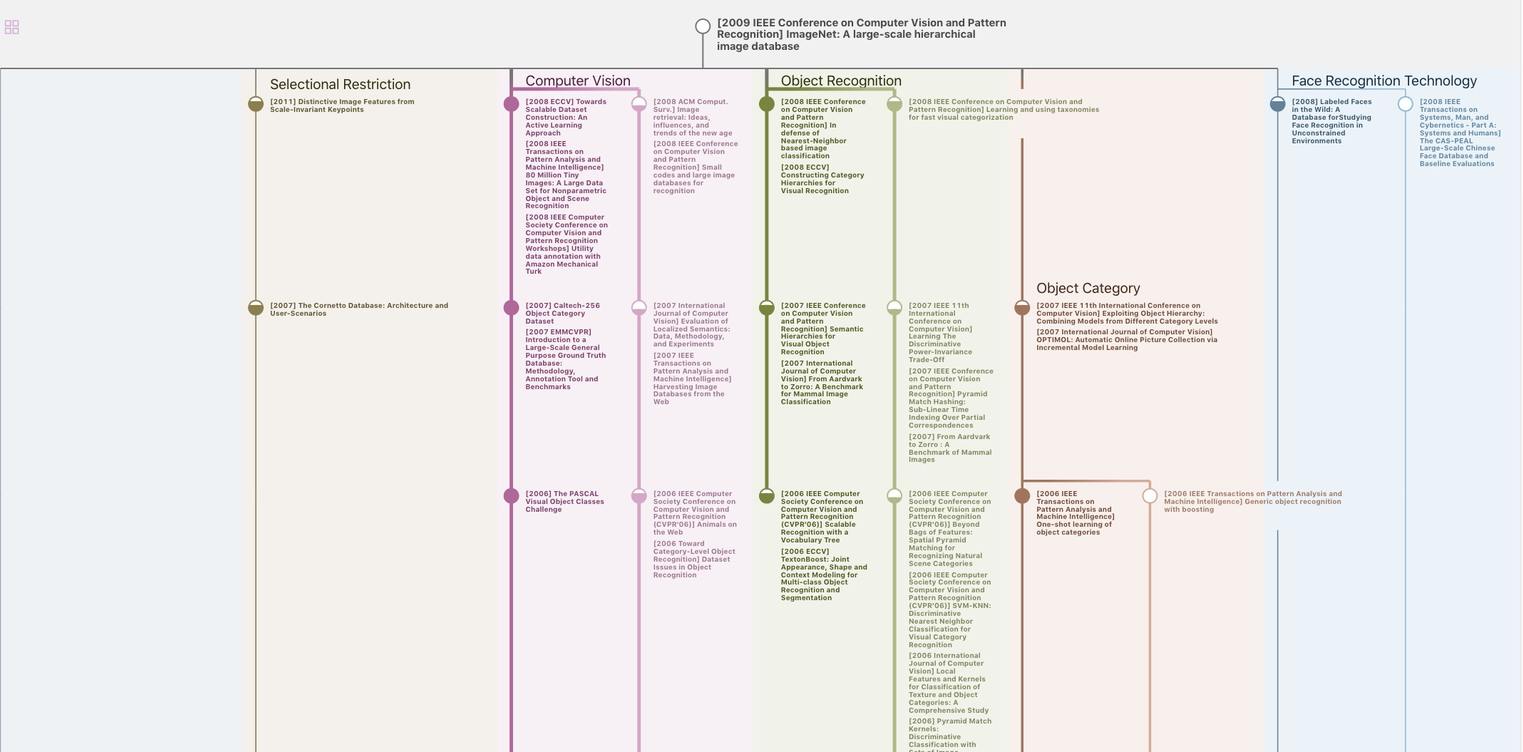
生成溯源树,研究论文发展脉络
Chat Paper
正在生成论文摘要