AMGmal: Adaptive Mask-Guided Adversarial Attack Against Malware Detection with Minimal Perturbation.
Computers & security(2023)
Abstract
Modern cyber security systems increasingly rely on deep neural networks (DNNs) to tackle the surge in security threats. However, it is well-known that DNNs are vulnerable to adversarial examples. The state-of-the-art adversarial malware attacks focus on fooling the detector without losing functionality, but ignore the magnitude of perturbation, which can lead to attack failure in real-world scenarios. This paper proposes a novel technique of generating adversarial malware examples, AMGmal, i.e., Adaptive Mask-Guided adversarial attack against malware detection with minimal perturbation. By prioritizing the modification of critical bytes at slack areas by saliency detection and mask guidance technique, we find an optimal balance between the evasion rate, perturbation magnitude and running time through three strategies, namely Dilation, Erosion and Heuristic. The proposed strategies can be extended to other attack methods as a generic post-processing step to minimize the perturbation. We evaluate the performance of AMGmal on the Malimg and SOREL-20M datasets and five DNN-based malware detectors. Experimental results show that the perturbation magnitude is reduced to one-third of base attack, from 3.34% to 0.90% and 2.32% to 0.73%. Compared to existing methods, AMGmal has the highest success rate of 68.09% and 64.75% when the amount of perturbation is similar, and vice versa. Furthermore, AMGmal is still effective even with defenses in place.
MoreTranslated text
Key words
Malware detection,Deep neural network,Malware visualization,Adversarial attack,Saliency detection
AI Read Science
Must-Reading Tree
Example
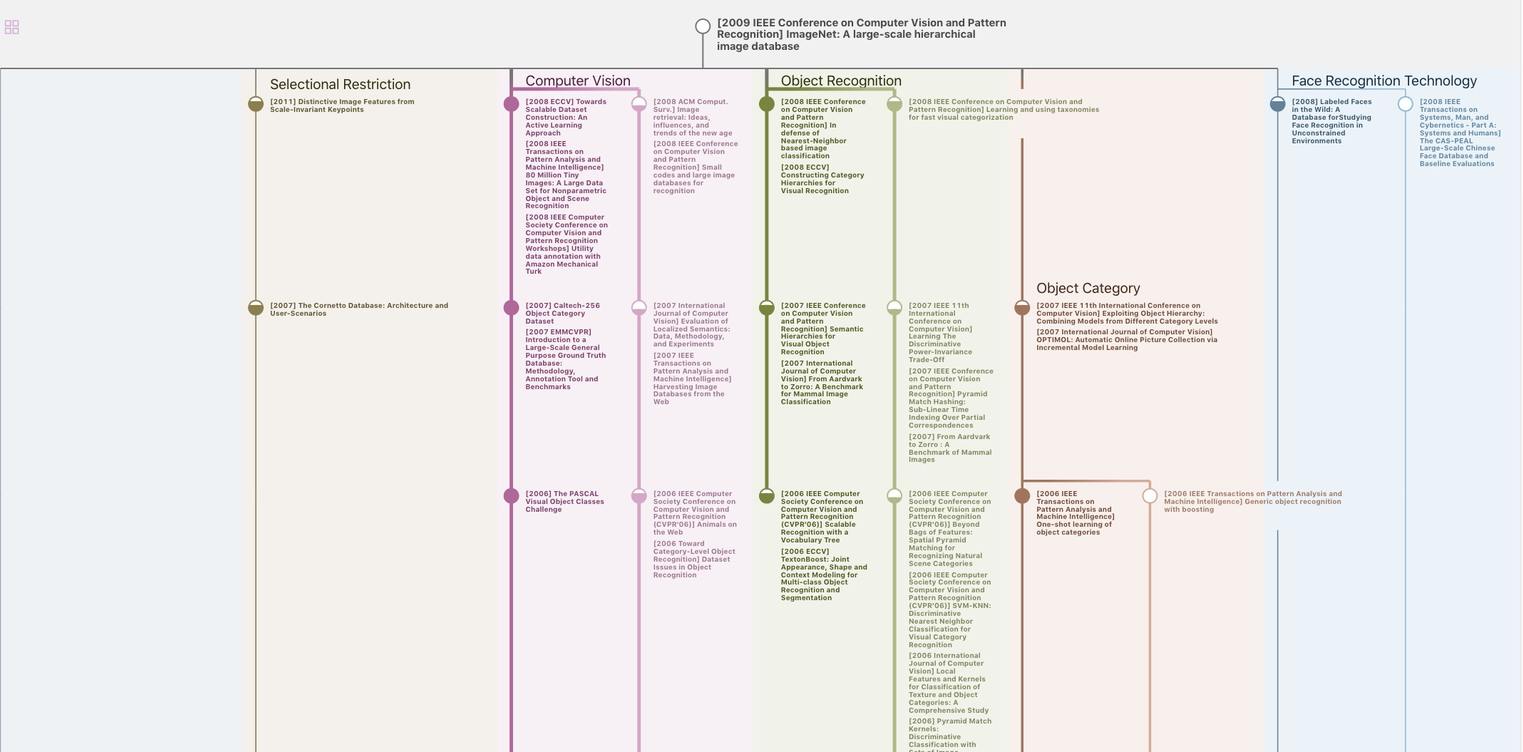
Generate MRT to find the research sequence of this paper
Chat Paper
Summary is being generated by the instructions you defined