Efficient Neural Network DPD Architecture for Hybrid Beamforming Mmimo
Electronics(2023)
摘要
This paper presents several different Neural Network based DPD architectures for hybrid beamforming (HBF) mMIMO applications. They are formulated, tested and compared based on their ability to compensate nonlinear distortion of power amplifiers in a single user (SU) and multiuser (MU) Fully-Connected (FC) HBF mMIMO transmitters. The proof-of-concept is provided with a 64 × 64 FC HBF mMIMO system, with 2 RF chains. The complexity of DPD solution is reduced by using a single Real-Valued Time-Delay Neural Network with two hidden layers (RVTDNN2L) instead of using as many different DPD blocks as there are RF chains in the HBF mMIMO transmitter and it is shown that the proposed architecture better compensates nonlinear distortion compared to the traditional memory polynomial DPD. Two RVTDNN2L DPD architectures are developed and tested for linearization of MU FC HBF mMIMO systems, and it is also shown that the proposed RVTDNN2L DPD architecture efficiently linearizes MU FC HBF mMIMO transmitters in terms of Normalized Mean-Squared Error (NMSE) and Error Vector Magnitude (EVM).
更多查看译文
关键词
neural networks,digital predistortion (DPD),massive MIMO,hybrid beamforming (HBF),power amplifiers (PAs)
AI 理解论文
溯源树
样例
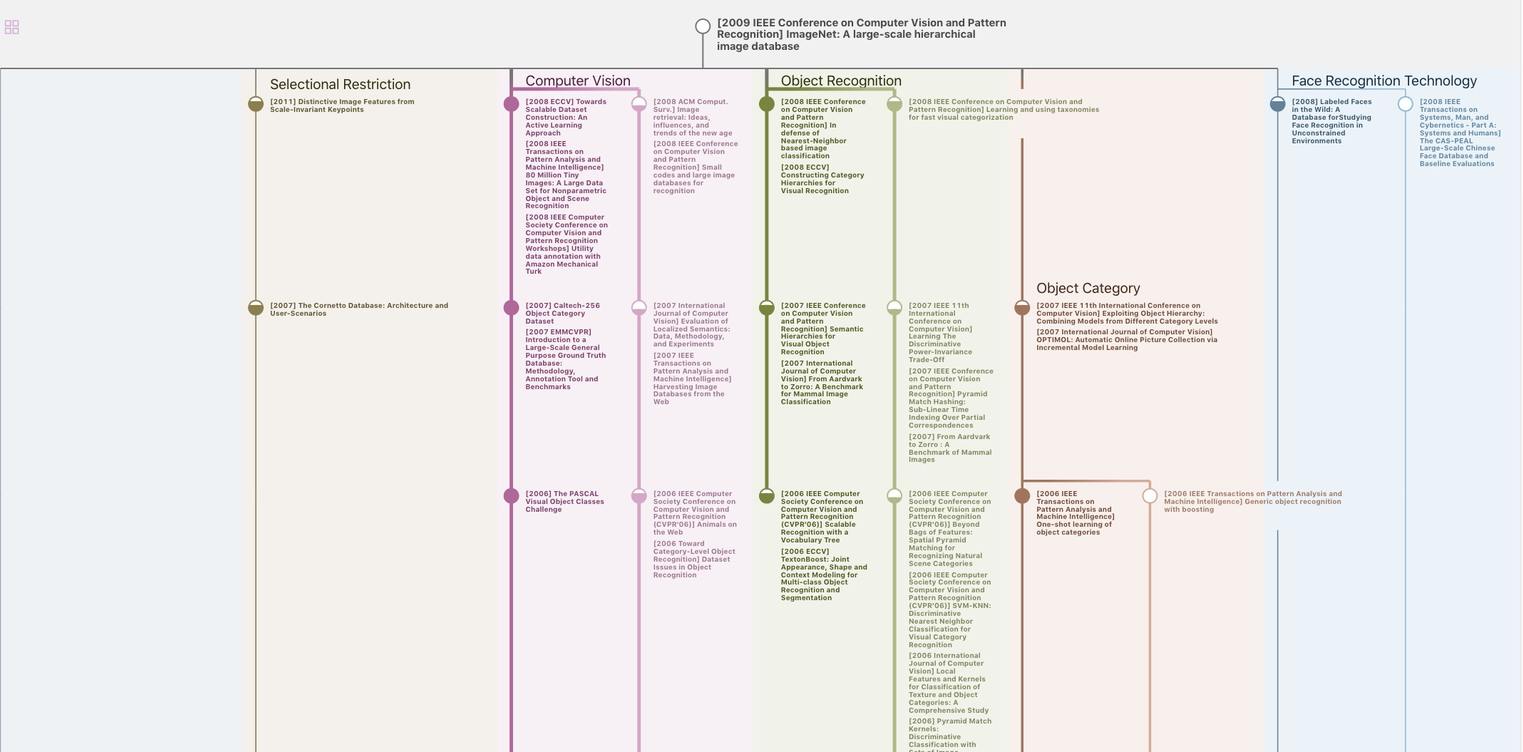
生成溯源树,研究论文发展脉络
Chat Paper
正在生成论文摘要