Machine Learning for Energy Load Prediction and its Interpretation
2022 IEEE 11th International Conference on Intelligent Systems (IS)(2022)
摘要
This paper refers to real-life data on energy consumption from three companies, connected to a medium voltage grid. The companies use single-, two-, or three-zone tariffs and the general characteristic of their activity is comparable. The input dataset contains hourly electricity consumption recorded from January 2020 to December 2020. The independent variables used for the study include weather, time-dependent factors, and aggregated energy factors. We perform the prediction of consumption using three methods: naive, multiple regression, and extreme gradient boosting regression. The performance of the methods is compared based on the MSE, RMSE, MAE, and MAPE metrics. The study shows that the scores achieved for the extreme gradient boosting method are higher than for the multiple regression. To gain the interpretability of the energy load forecasting and feature analysis the Shapley additive explanations method is used for the best model. As a result, a ranking of the most important factors is created. It shows the importance of aggregated energy factors and weather conditions while placing daylight and season at the bottom of the ranking.
更多查看译文
关键词
machine learning,regression model,Shapley additive explanations,electricity load forecasting,gradient boosting,feature evaluation
AI 理解论文
溯源树
样例
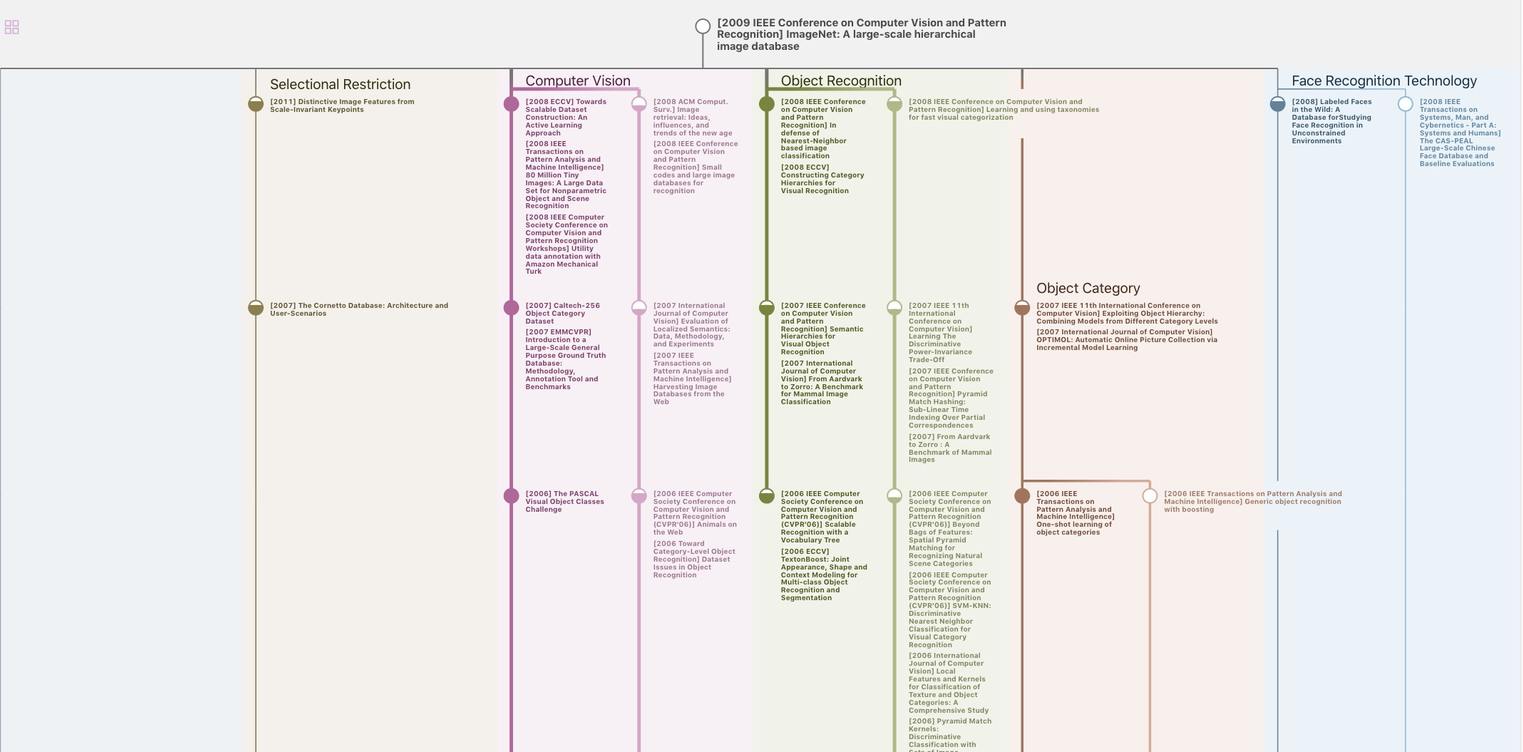
生成溯源树,研究论文发展脉络
Chat Paper
正在生成论文摘要