Label Enhancement with Sample Correlation via Sparse Representation
2022 Tenth International Conference on Advanced Cloud and Big Data (CBD)(2022)
摘要
Label distribution describes the instance by multiple labels with different intensities and accommodates to more-general conditions compared with single-label and multi-label annotations. In the past few years, many label enhancement (LE) algorithms are proposed in order to solve the problem that many training sets cannot use label distribution learning (LDL) algorithms because they only contain logical labels rather than label distribution. To handle this problem, this paper proposes a novel LE algorithm called Label Enhancement with sample correlations via Sparse Representation (LE-SR). Unlike most existing methods, a sparse representation method is employed so as to capture the global relationships of samples and predict implicit label correlations to achieve label enhancement. Experimental results on thirteen real-world datasets show clear advantages of LE-SR over several existing LE algorithms.
更多查看译文
关键词
label enhancement,label distribution learning,sparse Representation
AI 理解论文
溯源树
样例
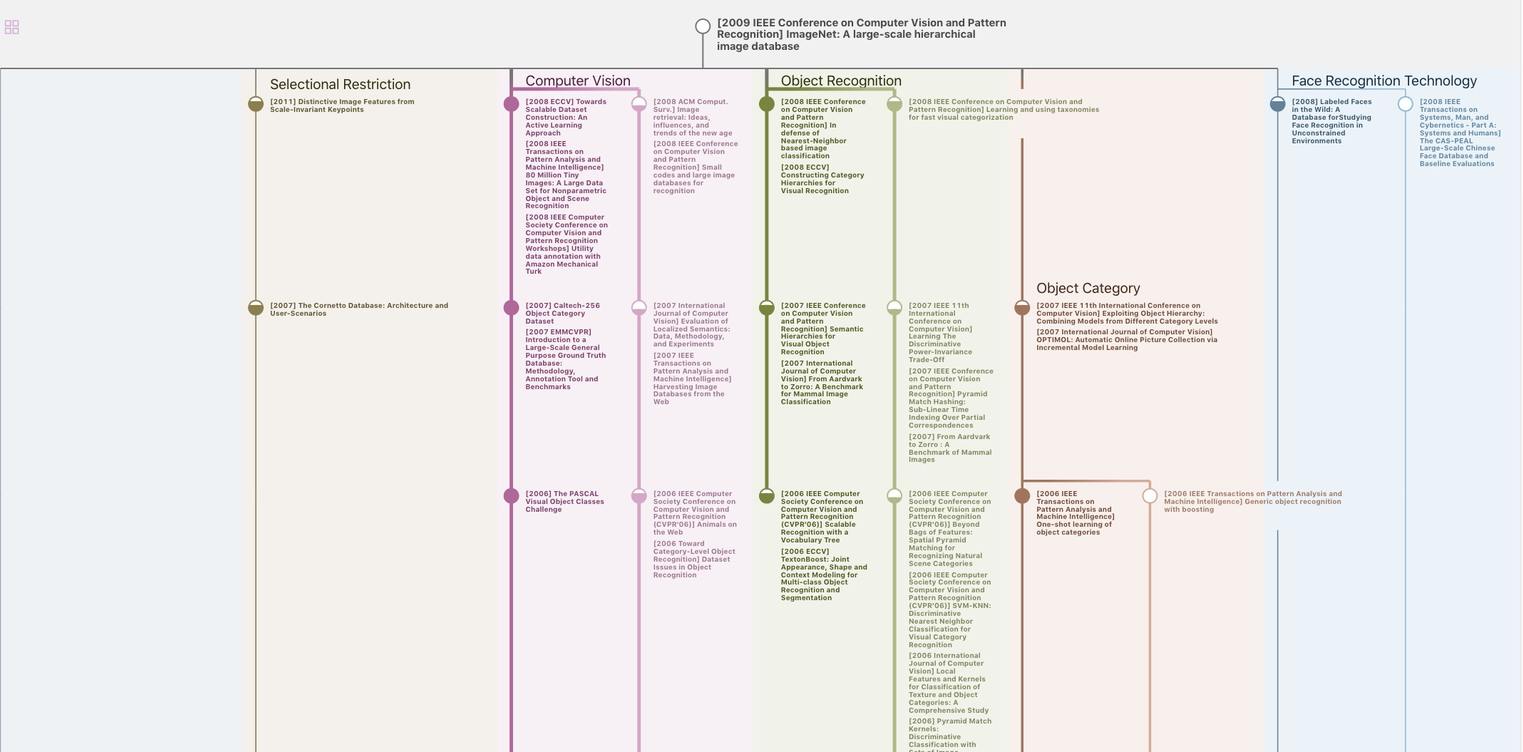
生成溯源树,研究论文发展脉络
Chat Paper
正在生成论文摘要