De-Noising of Sparse Signals Using Mixture Model Shrinkage Function.
IEEE Access(2023)
摘要
In this work a new thresholding function referred to as ’mixture model shrinkage’ (MMS) based on the minimization of a convex cost function is proposed. Normally, thresholding functions underestimate larger signal amplitudes during the de-noising process. The proposed model is a more flexible shrinkage function as it solves the underestimation problem to a greater extent and thus efficiently de-noises the signal without affecting signal amplitudes. The Expectation minimization (EM) algorithm is used to find the model parameters along with the majorization-minimization (MM) algorithm that minimize the monotonic cost function. The proposed model is then applied for de-noising group sparse signals and Shepp Logan phantom images. Our experimental study shows that MMS outclasses current thresholding functions and overlapping group shrinkage algorithm without results suffering from underestimation. Furthermore, the proposed model has the smallest Root Mean Square Error (RMSE) for de-noising group sparse signals.
更多查看译文
关键词
Convex optimization,EM algorithm,maximum a posteriori estimator (MAP),MM algorithm,PSNR,RMSE
AI 理解论文
溯源树
样例
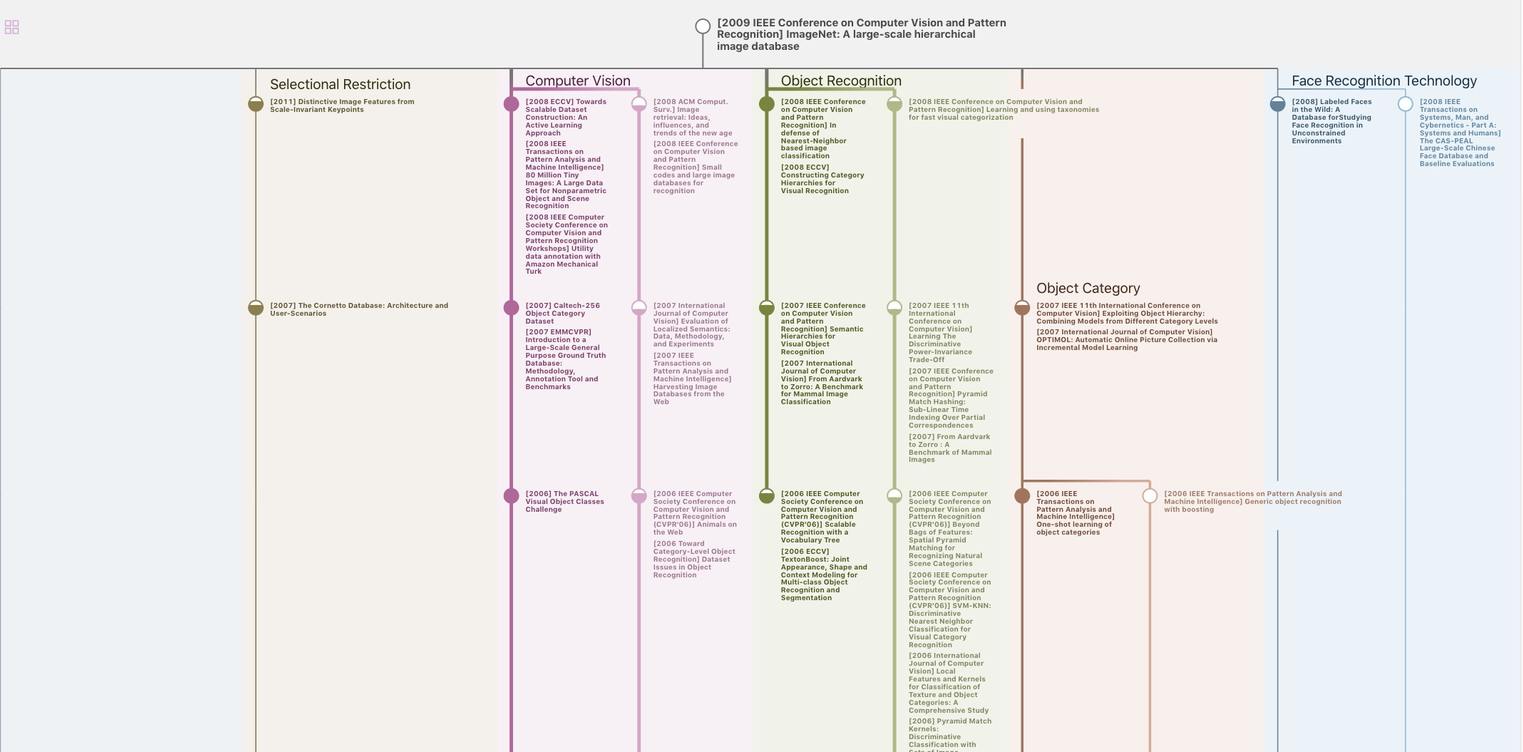
生成溯源树,研究论文发展脉络
Chat Paper
正在生成论文摘要