Think before you shrink: Alternatives to default shrinkage methods can improve prediction accuracy, calibration and coverage
arxiv(2023)
摘要
While shrinkage is essential in high-dimensional settings, its use for low-dimensional regression-based prediction has been debated. It reduces variance, often leading to improved prediction accuracy. However, it also inevitably introduces bias, which may harm two other measures of predictive performance: calibration and coverage of confidence intervals. Much of the criticism stems from the usage of standard shrinkage methods, such as lasso and ridge with a single, cross-validated penalty. Our aim is to show that readily available alternatives can strongly improve predictive performance, in terms of accuracy, calibration or coverage. For linear regression, we use small sample splits of a large, fairly typical epidemiological data set to illustrate this. We show that usage of differential ridge penalties for covariate groups may enhance prediction accuracy, while calibration and coverage benefit from additional shrinkage of the penalties. In the logistic setting, we apply an external simulation to demonstrate that local shrinkage improves calibration with respect to global shrinkage, while providing better prediction accuracy than other solutions, like Firth's correction. The benefits of the alternative shrinkage methods are easily accessible via example implementations using \texttt{mgcv} and \texttt{r-stan}, including the estimation of multiple penalties. A synthetic copy of the large data set is shared for reproducibility.
更多查看译文
关键词
default shrinkage methods,prediction accuracy,calibration
AI 理解论文
溯源树
样例
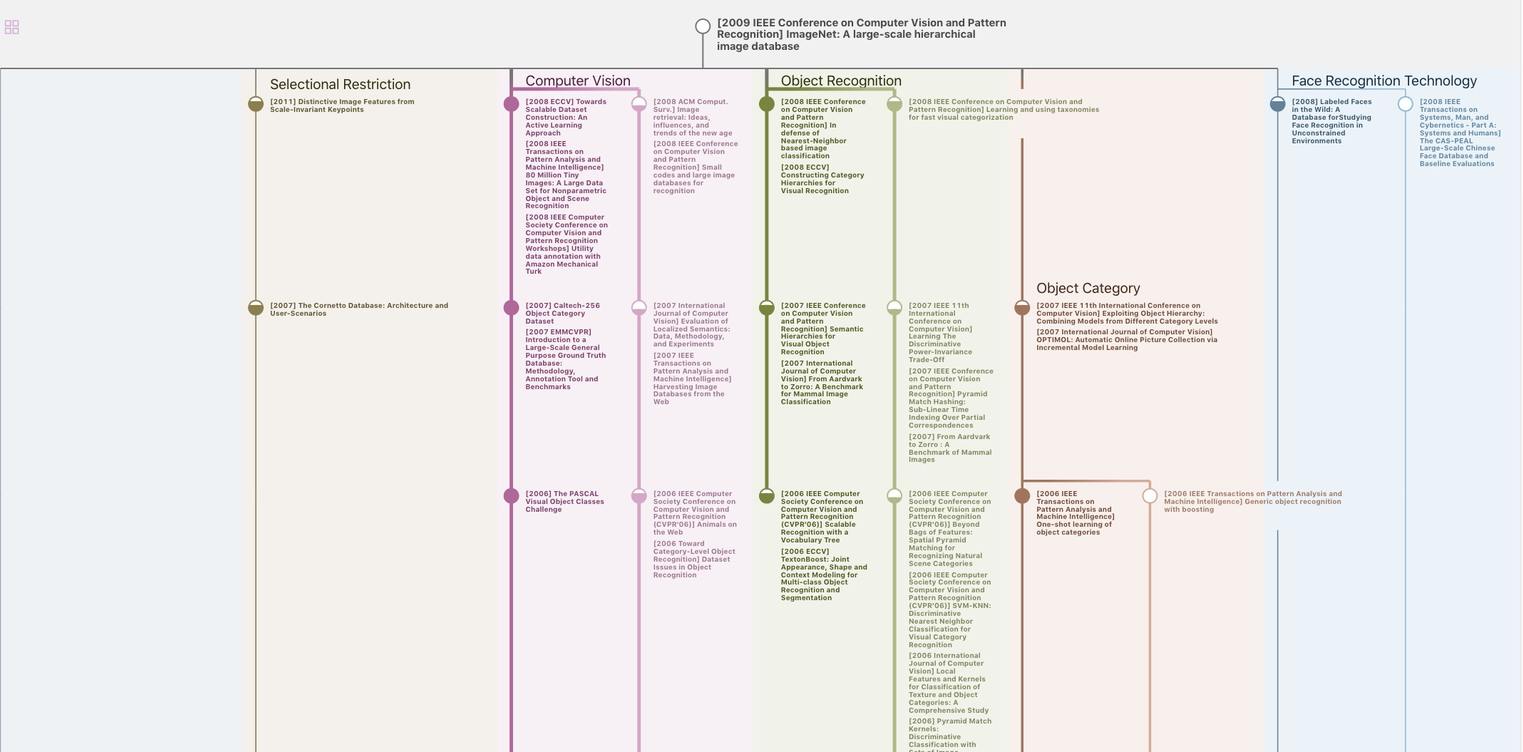
生成溯源树,研究论文发展脉络
Chat Paper
正在生成论文摘要