Learning Effective Strategies for Moving Target Defense with Switching Costs
arxiv(2023)
摘要
Moving Target Defense (MTD) has emerged as a key technique in various security applications as it takes away the attacker's ability to perform reconnaissance for exploiting a system's vulnerabilities. However, most of the existing research in the field assumes unrealistic access to information about the attacker's motivations and/or actions when developing MTD strategies. Many of the existing approaches also assume complete knowledge regarding the vulnerabilities of a system and how each of these vulnerabilities can be exploited by an attacker. In this work, we aim to create algorithms that generate effective Moving Target Defense strategies that do not rely on prior knowledge about the attackers. Our work assumes that the only way the defender receives information about its own reward is via interaction with the attacker in a repeated game setting. Depending on the amount of information that can be obtained from the interactions, we devise two different algorithms using multi-armed bandit formulation to identify efficient strategies. We then evaluate our algorithms using data mined from the National Vulnerability Database to showcase that they match the performance of the state-of-the-art techniques, despite using a lot less amount of information.
更多查看译文
关键词
target defense,effective strategies
AI 理解论文
溯源树
样例
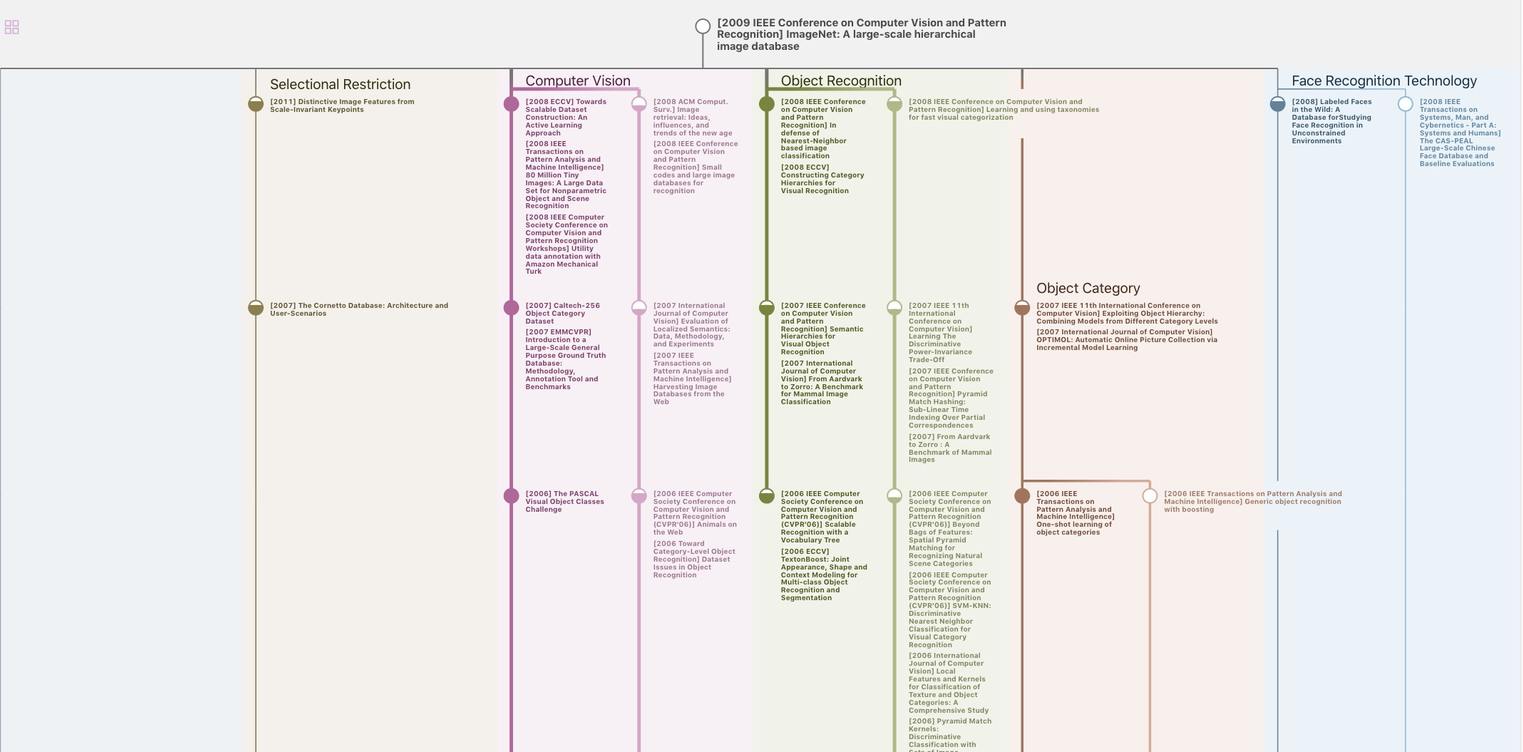
生成溯源树,研究论文发展脉络
Chat Paper
正在生成论文摘要