Investigating Labeler Bias in Face Annotation for Machine Learning
arxiv(2023)
摘要
In a world increasingly reliant on artificial intelligence, it is more important than ever to consider the ethical implications of artificial intelligence on humanity. One key under-explored challenge is labeler bias, which can create inherently biased datasets for training and subsequently lead to inaccurate or unfair decisions in healthcare, employment, education, and law enforcement. Hence, we conducted a study to investigate and measure the existence of labeler bias using images of people from different ethnicities and sexes in a labeling task. Our results show that participants possess stereotypes that influence their decision-making process and that labeler demographics impact assigned labels. We also discuss how labeler bias influences datasets and, subsequently, the models trained on them. Overall, a high degree of transparency must be maintained throughout the entire artificial intelligence training process to identify and correct biases in the data as early as possible.
更多查看译文
关键词
face annotation,labeler bias,machine learning
AI 理解论文
溯源树
样例
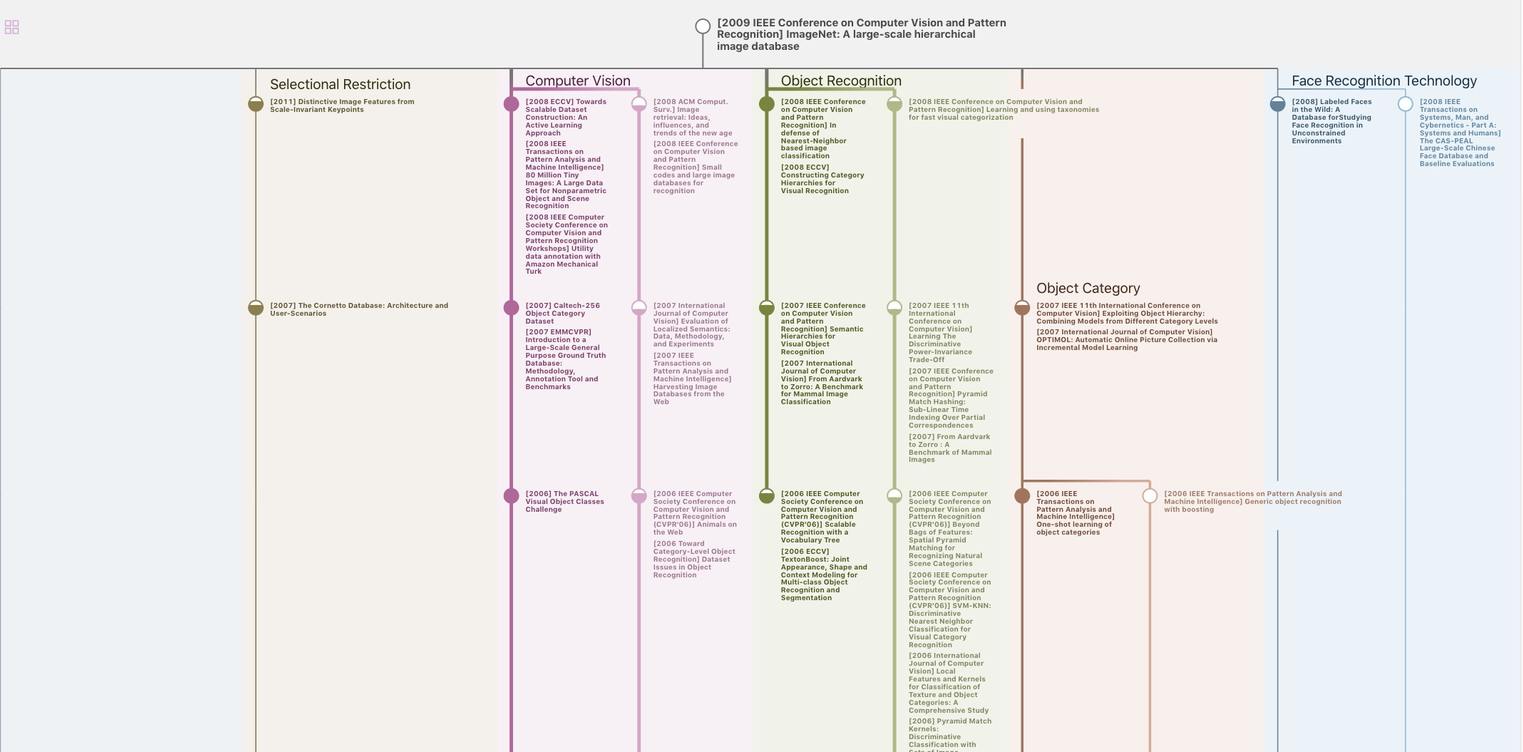
生成溯源树,研究论文发展脉络
Chat Paper
正在生成论文摘要