Progressive Meta-Pooling Learning for Lightweight Image Classification Model
arxiv(2023)
摘要
Practical networks for edge devices adopt shallow depth and small convolutional kernels to save memory and computational cost, which leads to a restricted receptive field. Conventional efficient learning methods focus on lightweight convolution designs, ignoring the role of the receptive field in neural network design. In this paper, we propose the Meta-Pooling framework to make the receptive field learnable for a lightweight network, which consists of parameterized pooling-based operations. Specifically, we introduce a parameterized spatial enhancer, which is composed of pooling operations to provide versatile receptive fields for each layer of a lightweight model. Then, we present a Progressive Meta-Pooling Learning (PMPL) strategy for the parameterized spatial enhancer to acquire a suitable receptive field size. The results on the ImageNet dataset demonstrate that MobileNetV2 using Meta-Pooling achieves top1 accuracy of 74.6\%, which outperforms MobileNetV2 by 2.3\%.
更多查看译文
关键词
lightweight image classification
AI 理解论文
溯源树
样例
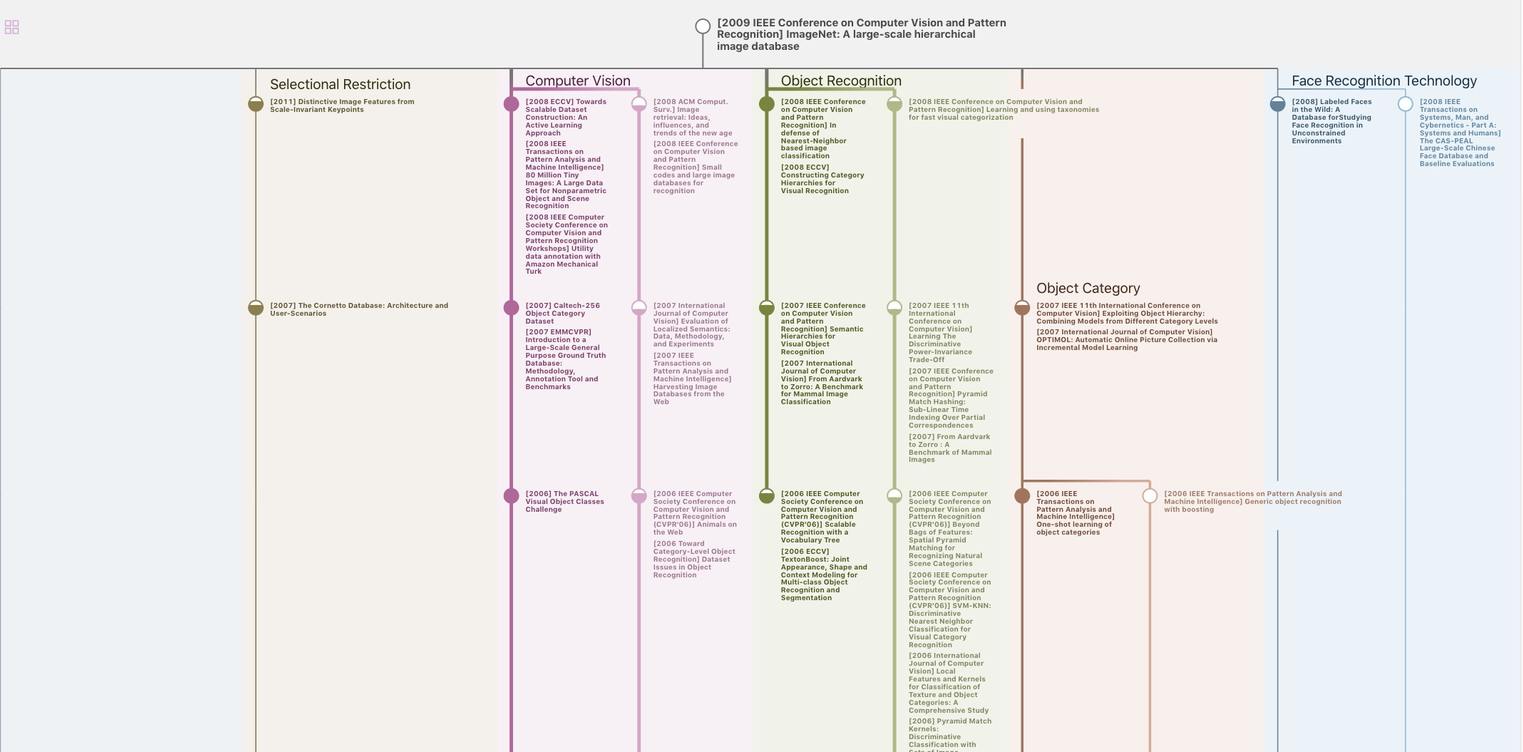
生成溯源树,研究论文发展脉络
Chat Paper
正在生成论文摘要