FedHQL: Federated Heterogeneous Q-Learning
arxiv(2023)
摘要
Federated Reinforcement Learning (FedRL) encourages distributed agents to learn collectively from each other's experience to improve their performance without exchanging their raw trajectories. The existing work on FedRL assumes that all participating agents are homogeneous, which requires all agents to share the same policy parameterization (e.g., network architectures and training configurations). However, in real-world applications, agents are often in disagreement about the architecture and the parameters, possibly also because of disparate computational budgets. Because homogeneity is not given in practice, we introduce the problem setting of Federated Reinforcement Learning with Heterogeneous And bLack-box agEnts (FedRL-HALE). We present the unique challenges this new setting poses and propose the Federated Heterogeneous Q-Learning (FedHQL) algorithm that principally addresses these challenges. We empirically demonstrate the efficacy of FedHQL in boosting the sample efficiency of heterogeneous agents with distinct policy parameterization using standard RL tasks.
更多查看译文
关键词
fedhql,q-learning
AI 理解论文
溯源树
样例
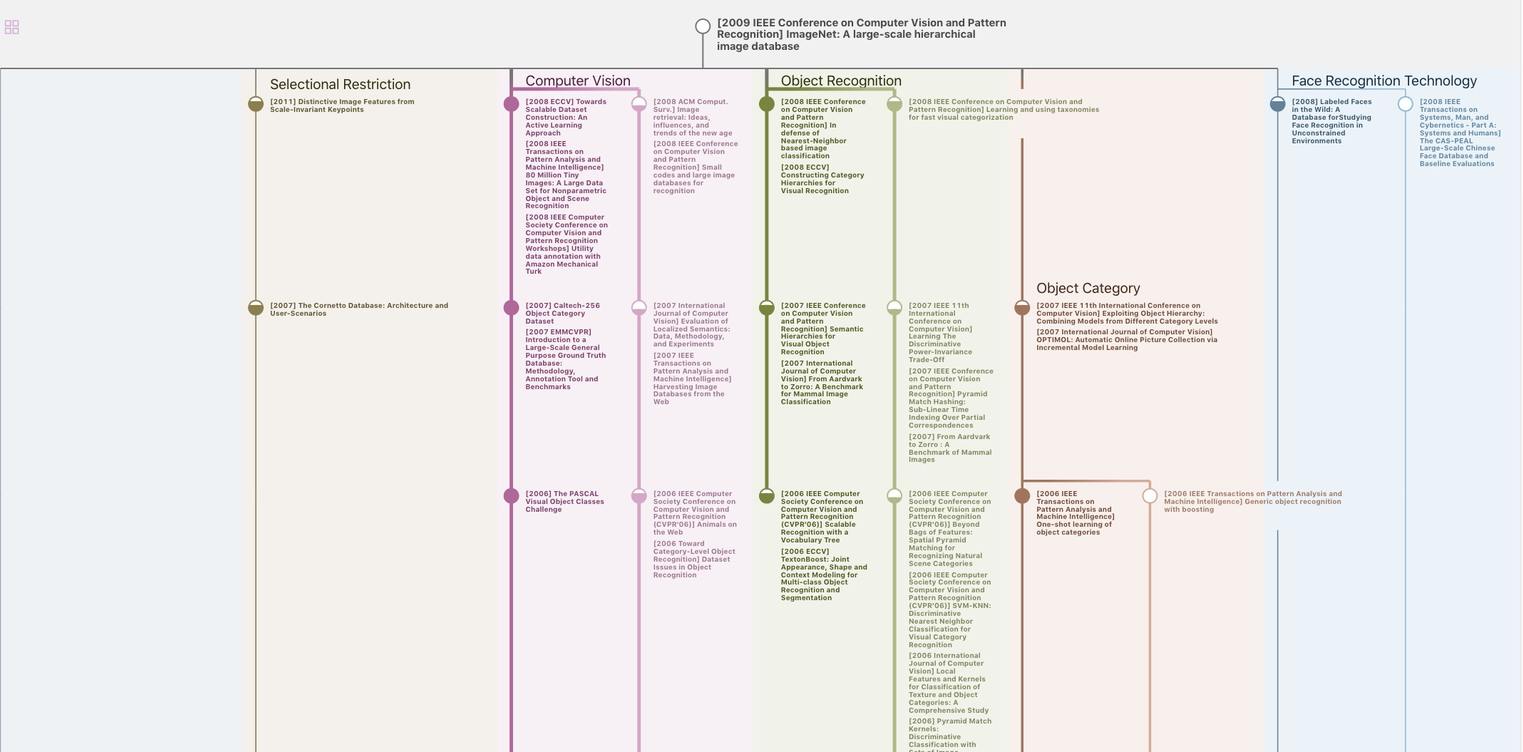
生成溯源树,研究论文发展脉络
Chat Paper
正在生成论文摘要