Prediction of Visual Impairment in Epiretinal Membrane and Feature Analysis: A Deep Learning Approach Using Optical Coherence Tomography
ASIA-PACIFIC JOURNAL OF OPHTHALMOLOGY(2023)
摘要
Purpose:The aim was to develop a deep learning model for predicting the extent of visual impairment in epiretinal membrane (ERM) using optical coherence tomography (OCT) images, and to analyze the associated features. Methods:Six hundred macular OCT images from eyes with ERM and no visually significant media opacity or other retinal diseases were obtained. Those with best-corrected visual acuity <= 20/50 were classified as "profound visual impairment," while those with best-corrected visual acuity >20/50 were classified as "less visual impairment." Ninety percent of images were used as the training data set and 10% were used for testing. Two convolutional neural network models (ResNet-50 and ResNet-18) were adopted for training. The t-distributed stochastic neighbor-embedding approach was used to compare their performances. The Grad-CAM technique was used in the heat map generative phase for feature analysis. Results:During the model development, the training accuracy was 100% in both convolutional neural network models, while the testing accuracy was 70% and 80% for ResNet-18 and ResNet-50, respectively. The t-distributed stochastic neighbor-embedding approach found that the deeper structure (ResNet-50) had better discrimination on OCT characteristics for visual impairment than the shallower structure (ResNet-18). The heat maps indicated that the key features for visual impairment were located mostly in the inner retinal layers of the fovea and parafoveal regions. Conclusions:Deep learning algorithms could assess the extent of visual impairment from OCT images in patients with ERM. Changes in inner retinal layers were found to have a greater impact on visual acuity than the outer retinal changes.
更多查看译文
关键词
artificial intelligence,deep learning,epiretinal membrane,optical coherence tomography
AI 理解论文
溯源树
样例
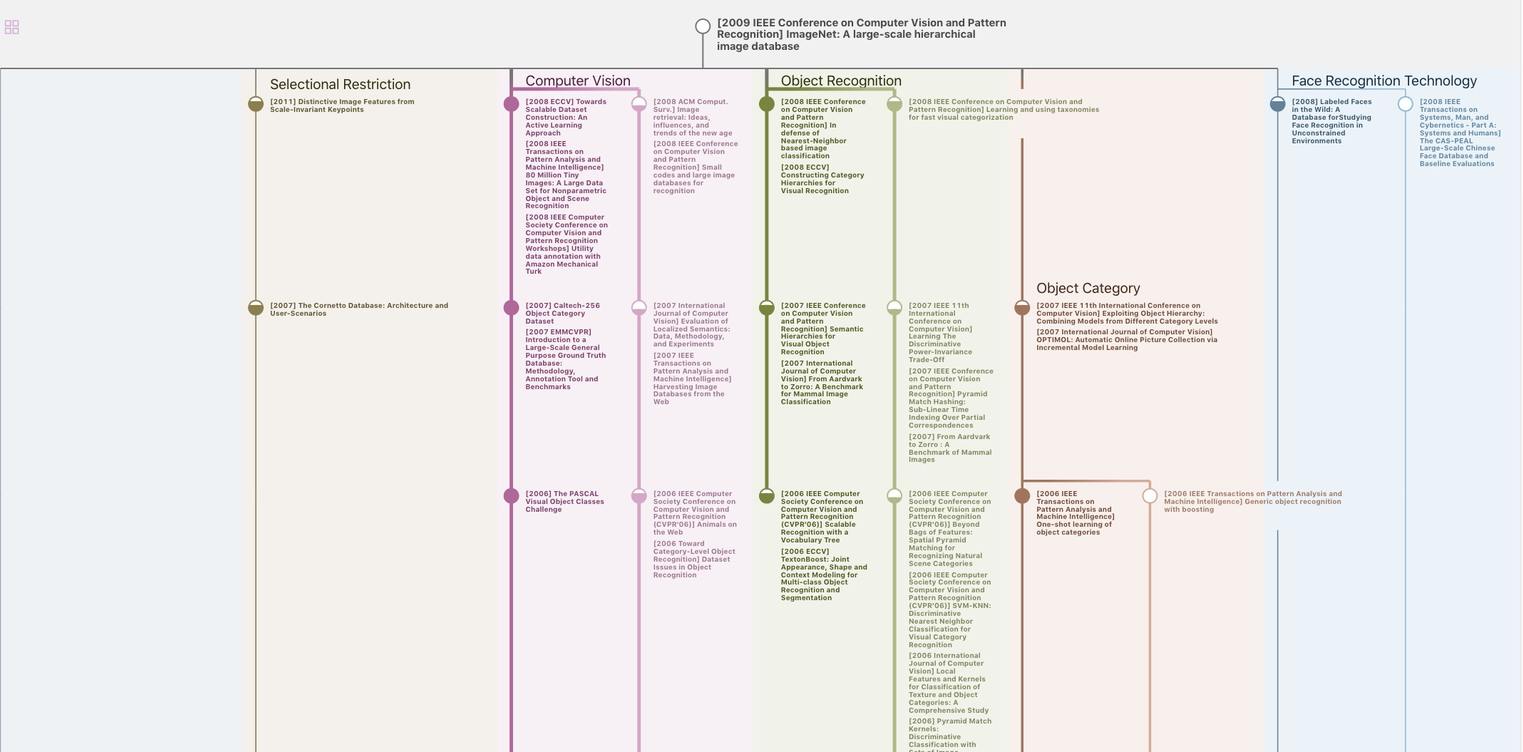
生成溯源树,研究论文发展脉络
Chat Paper
正在生成论文摘要