Workload Prediction for Adaptive Approximate Query Processing
2022 IEEE International Conference on Big Data (Big Data)(2022)
摘要
Approximate Query Processing (AQP) enables a trade-off between accuracy and performance to deliver users real-time responses. State-of-the-art AQP relies on forming compact data summaries and approximating the queries using these summaries. By understanding the sequencing of queries in the given workload, engines tune the construction of synopses and buffer them in a warehouse to reduce the cost of subsequent query execution costs. We present Adaptive Approximate Query Processing (AAQP), which predicts future workload and generates the best set of synopses to execute a given query, i.e., those that minimize the predicted workload’s execution time. We equip AAQP with Recurrent Neural Networks (RNN) that are trained with end-user sessions extracted from the historical workload. We conduct several experiments on real-world workloads to show that AAQP can effectively predict the future workload based on the recent queries and adapt the construction of synopses to minimize the workload execution time, almost nearing optimal performance.
更多查看译文
关键词
SQL Prediction,Approximate Query Processing,Neural Networks
AI 理解论文
溯源树
样例
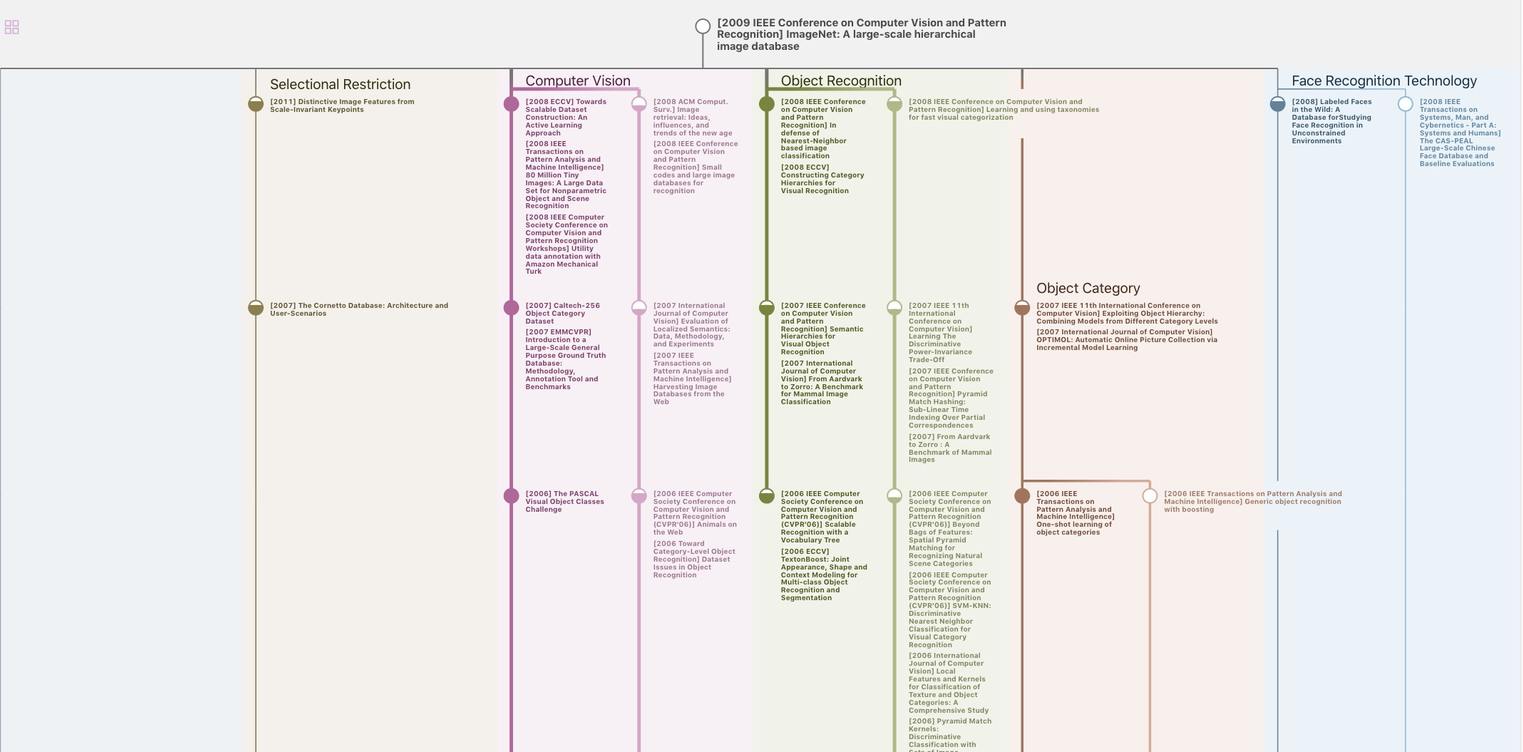
生成溯源树,研究论文发展脉络
Chat Paper
正在生成论文摘要