Comparative assessment of advanced machine learning techniques for simulation of lake water level fluctuations based on different dimensionality reduction methods
Earth Science Informatics(2023)
摘要
Global warming and unprecedented human impacts causing environmental degradation are taking place at an alarming rate. As one of the most valuable and important water resources that provides countless benefits to the ecosystem and residents, lakes are highly exposed to such threats. To better manage these precious resources, one of the most underlying steps in this field is accurate forecasting of the lake water level. In this study, the Reduced Error Pruning Tree (RT) model as one of the successful algorithms of machine learning coupled with three ensemble algorithms including Bagging (Ba), Random Committee (RC), and Random Subspace (RSS) were used to predict the monthly water level changes in Lake Urmia. Also, since the role of data analysis is very important for predicting the future state of the lake water level, two methods including Principal component analysis (PCA) and Sequential feature selection (SFS) were used to find the best input combination for model training. The analysis of the results showed that between the dimensionality reduction approaches, SFS presented better results than PCA. In addition, the Bagging and Random Committee ensemble algorithms typically performed better than the standalone models. Among all models developed in this study, the Ba-RT model based on SFS implemented with 2 months of lag times’ inputs (R = 0.99788, EVS = 0.99576, NSE = 0.99576, MAE = 0.10961, and RMSE = 0.14742) showed the best performance compared to the other models.
更多查看译文
关键词
Machine learning,Water level prediction,Ensemble models,PCA,Bagging REP Tree,Lake Urmia
AI 理解论文
溯源树
样例
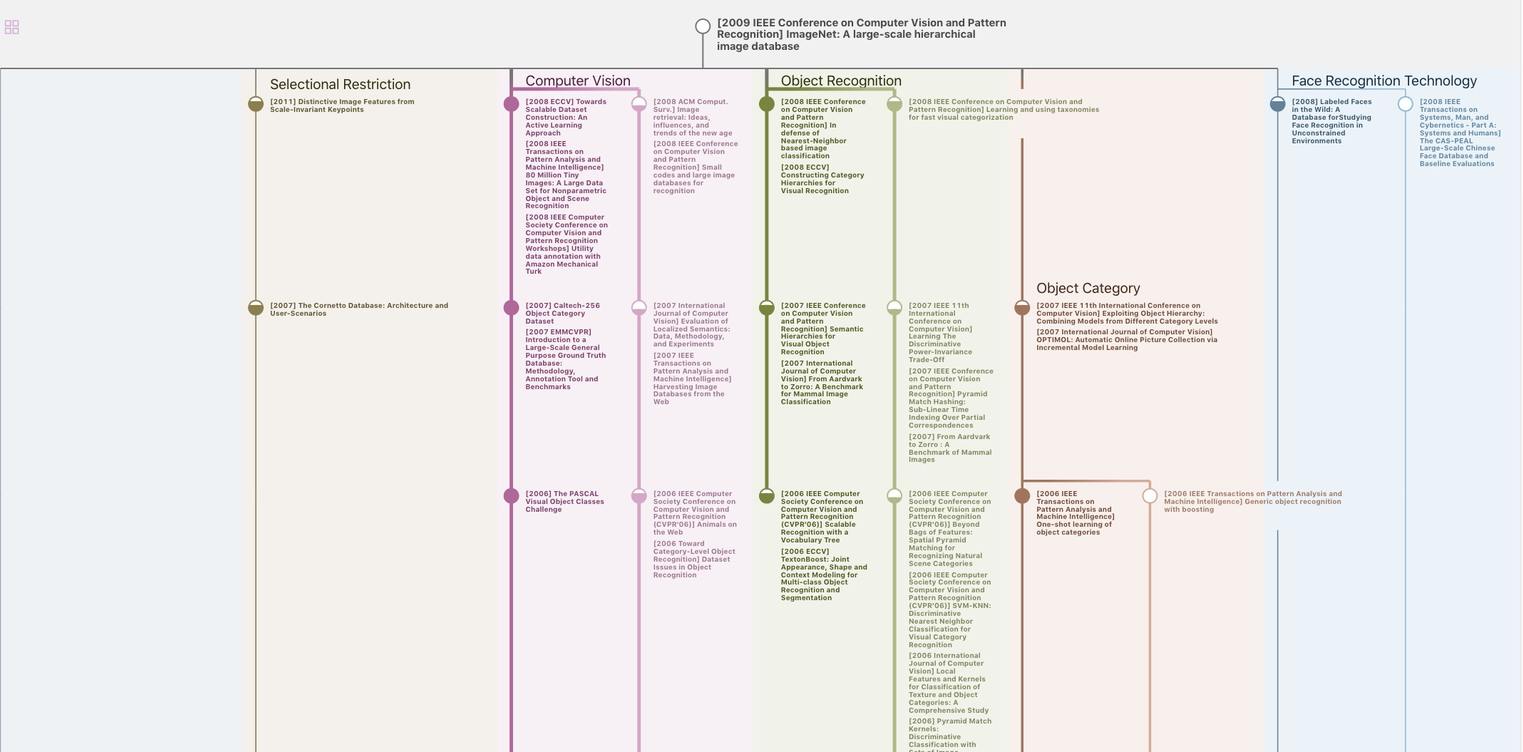
生成溯源树,研究论文发展脉络
Chat Paper
正在生成论文摘要