Deep-learning Methods for Integrated Sensing and Communication in Vehicular Networks
Vehicular communications(2023)
摘要
The integrated sensing and communication (ISAC) technique can significantly improve the overall system performance, especially in saving the bandwidth and transmitting power. In this paper, we focus on a vehicular scene of simultaneously sensing multiple targets and communicating with multiple users. We propose a signal scheme based on orthogonal frequency-division multiplexing (OFDM) and nonorthogonal multiple access (NOMA). In addition, we research the potential of applying deep-learning methods, including deep neural networks (DNNs) and convolutional neural networks (CNNs), to enhance the system's performance. A DNN-based multi-user demodulation scheme and a YOLOv5-SORT target tracking scheme in the ISAC scene are proposed, and the system realization methods are researched. The performance of the proposed system is evaluated by simulations. Under the given scene, we reach a mean-symbol-error-rate of 1×10−5 when the signal-noise-ratio is larger than 23 dB, and we reach the precision ratio, recall ratio, and the F1 value of about 0.98, 0.97, 0.97 at the confidence 0.5, respectively. In addition, the proposed schemes perform better than baselines in ISAC application scenarios.
更多查看译文
关键词
Integrated sensing and communication,Deep learning,Nonorthogonal multiple access,Orthogonal frequency division multiplexing
AI 理解论文
溯源树
样例
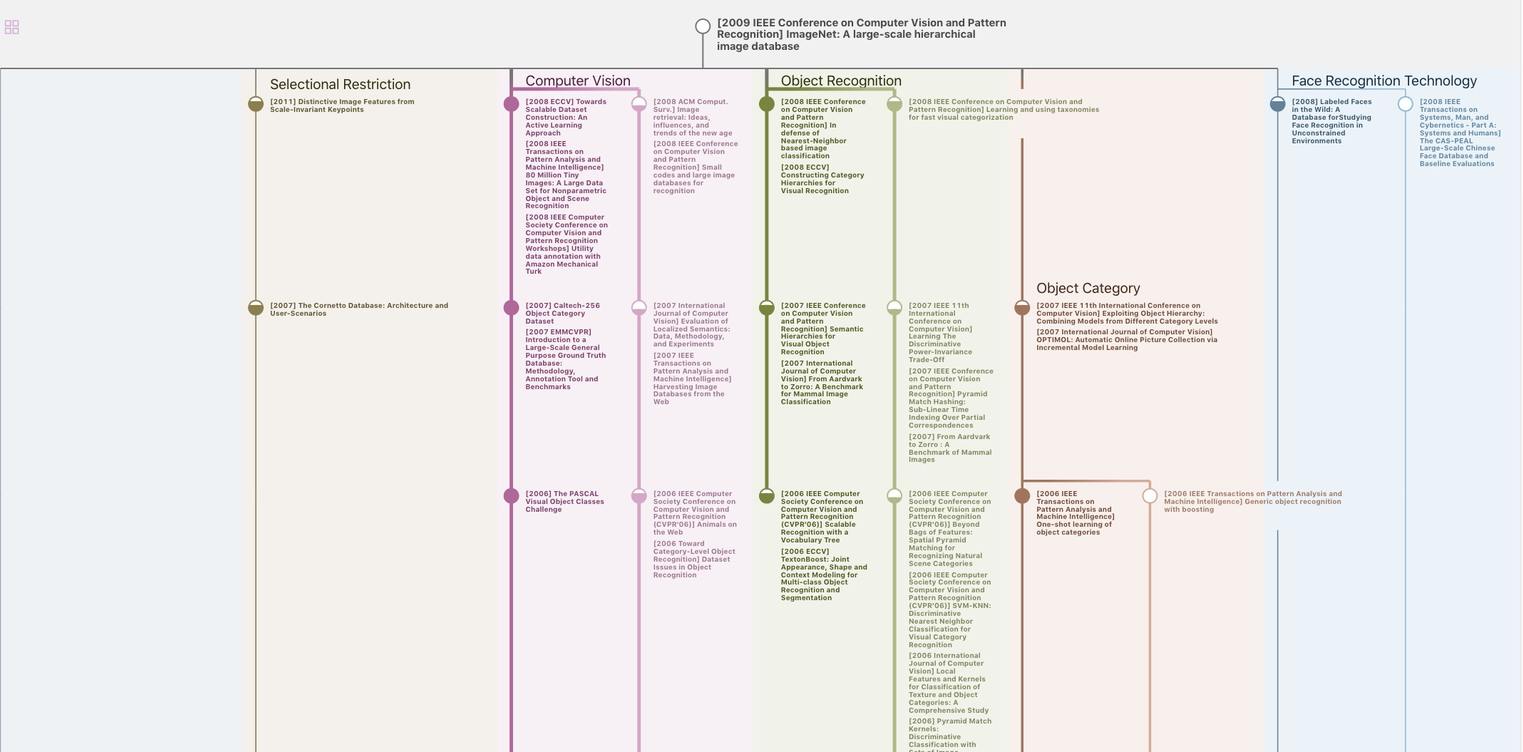
生成溯源树,研究论文发展脉络
Chat Paper
正在生成论文摘要