Cooperative Perception: Mitigating Messages Content Duplication
2022 5th International Conference on Communications, Signal Processing, and their Applications (ICCSPA)(2022)
摘要
Autonomous Vehicles (AVs) and smart vehicles rely on cooperative perception to complement the information missed by their sensors due to their limited range and non-line of sight objects. There are many applications that rely on communication leaving sometimes limited resources for cooperative perceptions. Therefore, optimizing the resources and messages sent over the network becomes crucial. An important factor that undermines the potential of vehicular networks is the number of messages containing information about the same objects. Not only this issue exhausts the limited network bandwidth and may lead to dropping some messages in high-density traffics but also prevent other useful information from being shared. This paper suggests a Game Theory Based Transmission (GTBT) to mitigate redundant message content in a fog-based vehicular network. We show that this approach is real-time and achieves 10.5% fewer duplicate messages compared to the Max Score Based Transmission (MSBT) baseline while it does not require any additional communication costs. Moreover, we contrast the pros and cons of our approach against two other baselines: random (Rand) and geo-filtering (Geo) transmission-based approaches, showing that GTBT is able to minimize the lost information (5.3% for GTBT vs 15.2% and 18.3% for Geo and Rand respectively) and is competing with Geo approach in the number of unique messages sent and their value. Lastly, the GTBT is able to provide a gain of 1.7 as calculated by our suggested new metric as a measure of the ability to compensate for the missed information, vs (- 0.5) for the Geo approach. To the best of our knowledge, this is the first study that employs game theory for selecting the content of the message for the cooperative perception problem.
更多查看译文
关键词
Game Theory,Message Content Selection,V2X
AI 理解论文
溯源树
样例
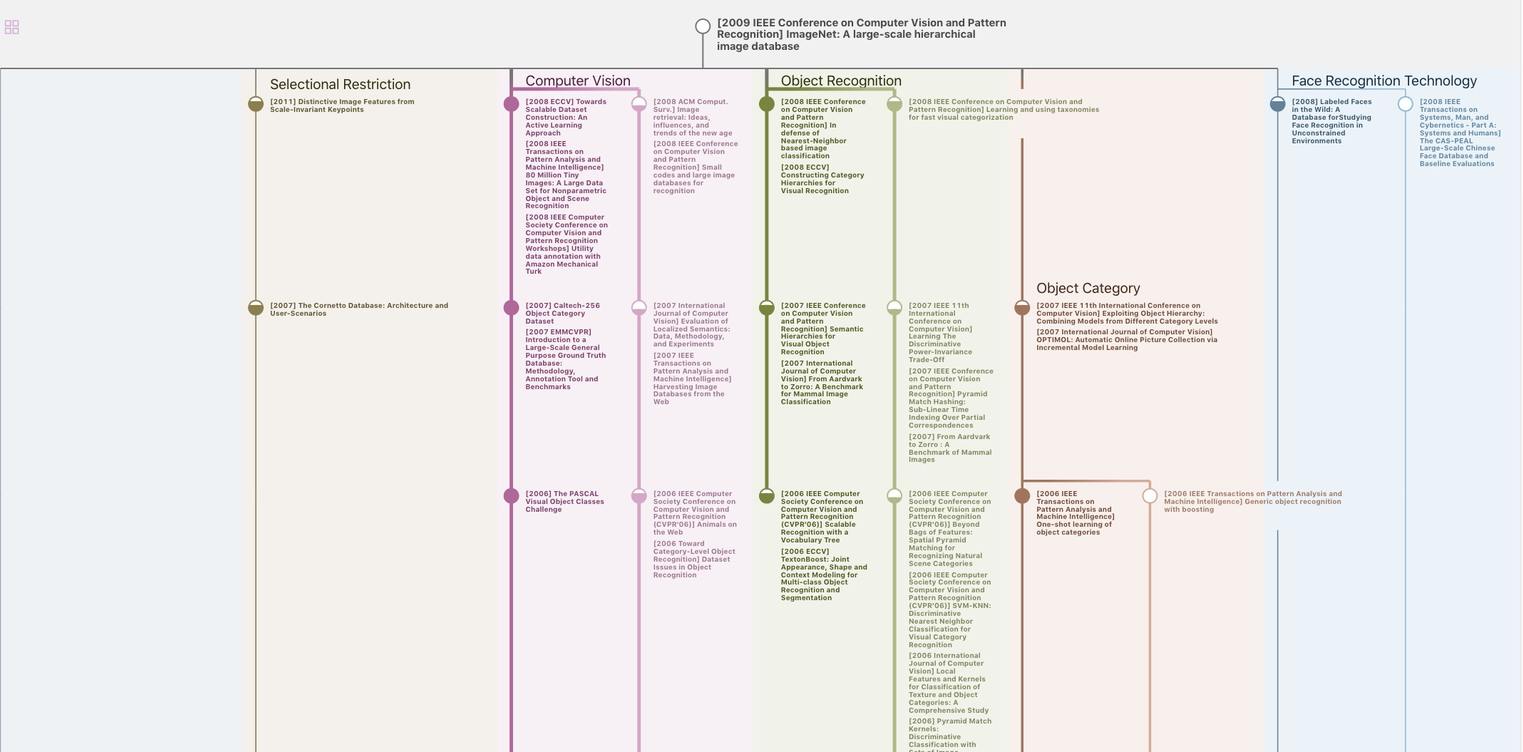
生成溯源树,研究论文发展脉络
Chat Paper
正在生成论文摘要