Unsupervised Domain Adaptation of Neural PLDA Using Segment Pairs for Speaker Verification
2022 IEEE Spoken Language Technology Workshop (SLT)(2023)
摘要
Probabilistic linear discriminant analysis (PLDA) is a popular tool in speaker recognition. PLDA parameters are estimated from labeled data, and domain shift causes performance degradation. Since obtaining a labeled dataset for target domain is costly, we propose an unsupervised domain adaptation of PLDA which does not require clustering or alignment. Our proposed method relies on discriminative training of PLDA using pseudo target/non-target labels. Segment pairs extracted from the same utterance that is likely to contain a single speaker are labeled as target, and pairs from randomly sampled utterances that are likely to belong to different speakers are labeled as non-target. We applied this approach to Neural PLDA. Our approach significantly improved the performance of the out-of-domain PLDA on the target domain with a relatively small amount of unlabeled data, performing on par with a baseline adaptation approach on SRE18 full-full scenario while outperforming it on full-10s and 10s-10s cases.
更多查看译文
关键词
speaker verification,PLDA,unsupervised learning,domain adaptation
AI 理解论文
溯源树
样例
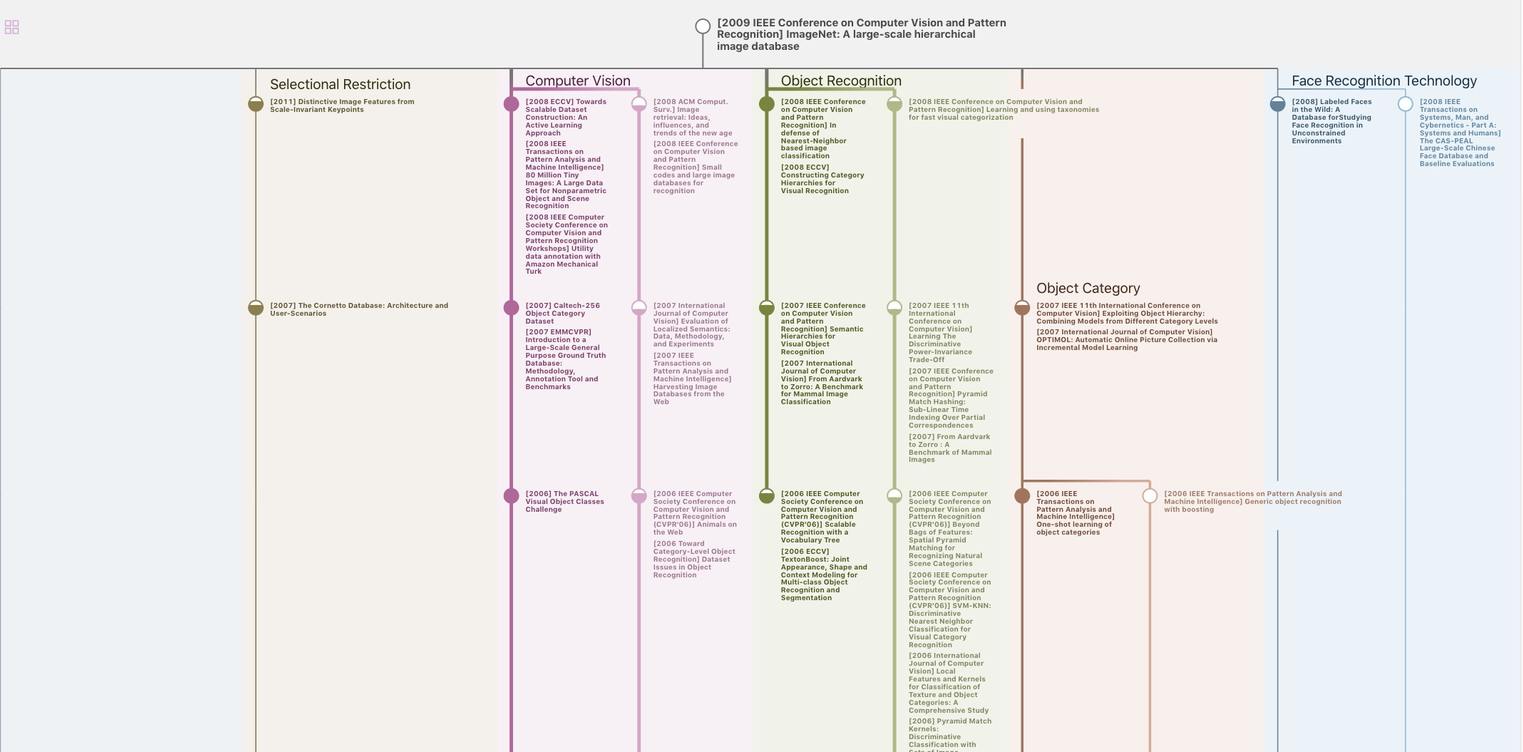
生成溯源树,研究论文发展脉络
Chat Paper
正在生成论文摘要