Species-aware DNA language modeling
biorxiv(2023)
摘要
Motivation: Predicting gene expression from DNA is an open field of research. As in many areas, labeled data is dwarfed by unlabelled data, i.e. species with a sequenced genome but no gene expression assay data. Pretraining on unlabelled data using masked language modeling has proven highly successful in overcoming data constraints in natural language and proteomics. However, in genomics, this approach has so far been applied only to single genomes, neither leveraging conservation of regulatory sequences across species nor the vast amount of available genomes. Results: Here we train a masked language model on more than 800 species spanning over 500 million years of evolution. We show that explicitly modeling species is instrumental in capturing conserved yet evolving regulatory elements and in controlling for oligomer biases. We extract embeddings for 3' untranslated regions of Saccharomyces cerevisiae and Schizosaccharomyces pombe and use them to achieve prediction of mRNA half-life that is better or on-par with the state-of-the-art, demonstrating the utility of the approach for regulatory genomics. Moreover, we show that the per-base reconstruction probability of our model significantly predicts RNA-binding protein bound sites directly. Altogether, our work establishes a self-supervised framework to leverage large genome collections of evolutionary distant species for regulatory genomics and contributes to alignment-free comparative genomics. Availability and implementation: The source code and trained models are available at: https://github.com/DennisGankin/species-aware-DNA-LM .
### Competing Interest Statement
The authors have declared no competing interest.
更多查看译文
AI 理解论文
溯源树
样例
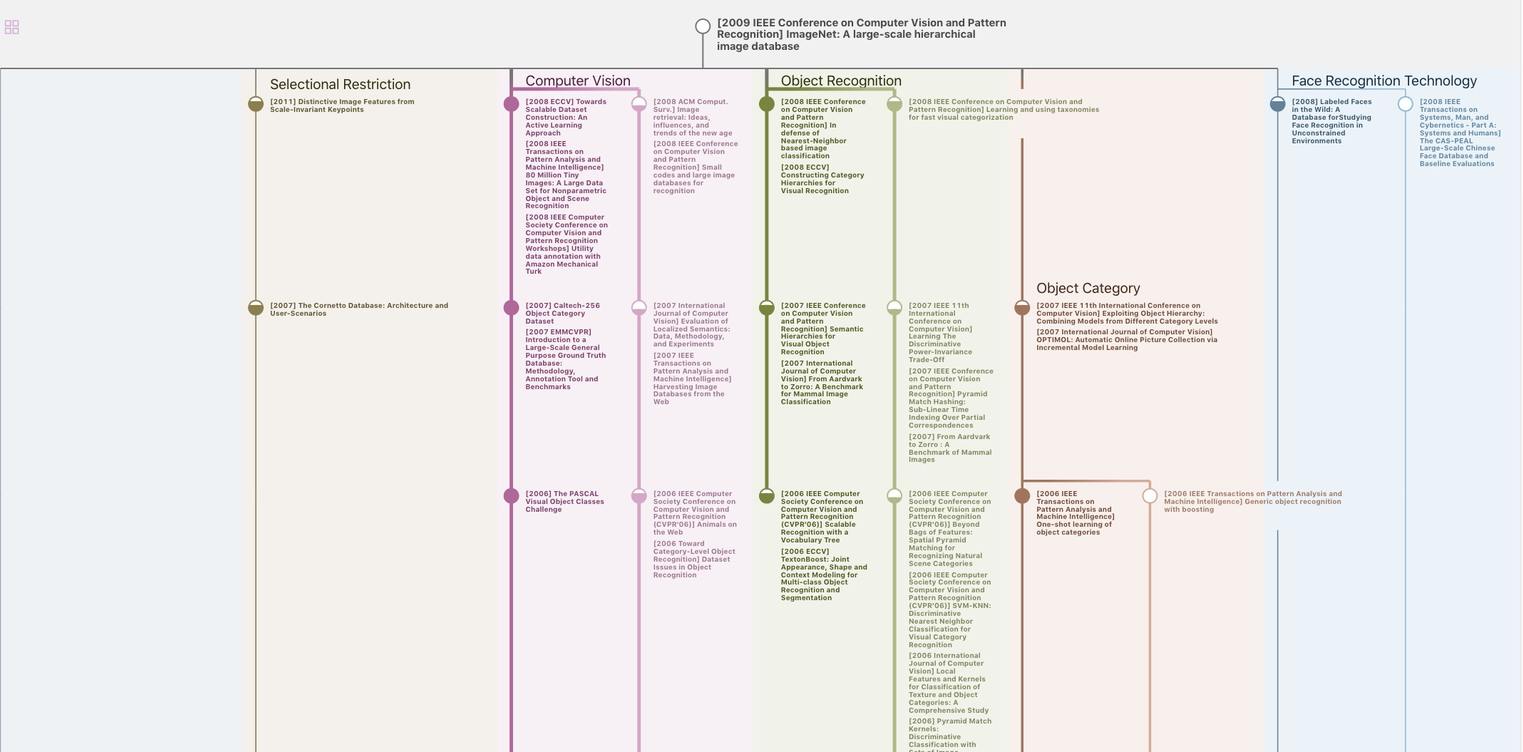
生成溯源树,研究论文发展脉络
Chat Paper
正在生成论文摘要