Combination of Multi-Fidelity Data Sources For Uncertainty Quantification: A Lightweight CNN Approach
arxiv(2023)
摘要
Reynolds Averaged Navier Stokes (RANS) modelling is notorious for introducing the model-form uncertainty due to the Boussinesq turbulent viscosity hypothesis. Recently, the eigenspace perturbation method (EPM) has been developed to estimate the RANS model-form uncertainty. This approach estimates model-form uncertainty through injecting perturbations to the predicted Reynolds stress tensor. However, there is a need for a reliable machine learning method for estimating the perturbed amplitude of the Reynolds stress tensor. Machine learning models are often too complex and data intensive for this application. We propose a lightweight convolutional neural network (CNN) approach to learn a correction function for RANS from paired-samples of RANS and DNS simulation results. The CNN learned RANS correction function successfully facilitates the RANS uncertainty quantification (UQ), and our findings suggest that the lightweight CNN approach is effective in combining RANS and DNS simulation results to enrich the existing perturbation method in estimating the RANS UQ more precisely.
更多查看译文
关键词
uncertainty
AI 理解论文
溯源树
样例
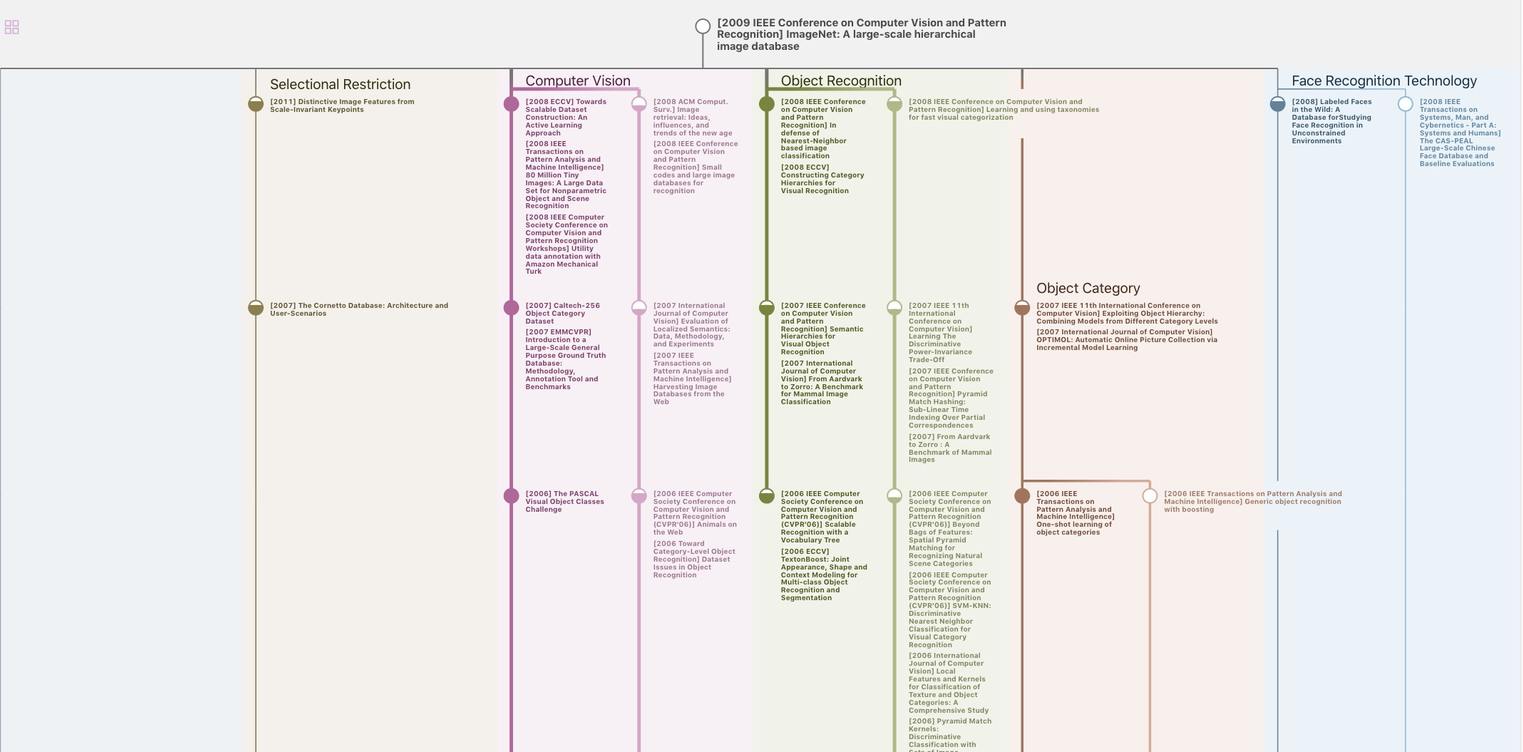
生成溯源树,研究论文发展脉络
Chat Paper
正在生成论文摘要