Complex Table Structure Recognition in the Wild Using Transformer and Identity Matrix-Based Augmentation.
ICFHR(2022)
摘要
Tables are a widely used and efficient data structure. Although people can intuitively understand table contents, it remains challenging for machines, especially the tables taken in the wild. Previous methods mainly focus on scanned or PDF tables, but ignore investigating camera-based tables. This paper treats table structure recognition (TSR) as an image-to-sequence recognition task and adopts an end-to-end trainable model for complex TSR in the wild. Specifically, the model consists of a CNN-based encoder and two Transformer-based decoding branches, which can simultaneously predict the logical and physical structures of a table. Currently available camera-based table datasets are scarce, but deep learning methods heavily rely on large-scale datasets. To alleviate data insufficiency and boost model's performance, we propose a new and effective table data augmentation method, called TabSplitter. Due to the complex structure caused by cells spanning multiple rows or columns, directly cropping will lead to damage and change the properties of these cells. To solve this problem, we proposed a matrix representation, named Identity Matrix (IM), to describe the table structure. Based on IM, we crop the tables and correct the cells whose attributes have changed, thus enhancing data diversity. Furthermore, the proposed IM facilitates the pre-processing of data and post-processing of predictions. Experimental results on several datasets demonstrate the effectiveness of the model and the TabSplitter for TSR, especially for complex tables in the wild.
更多查看译文
关键词
table,recognition,matrix-based
AI 理解论文
溯源树
样例
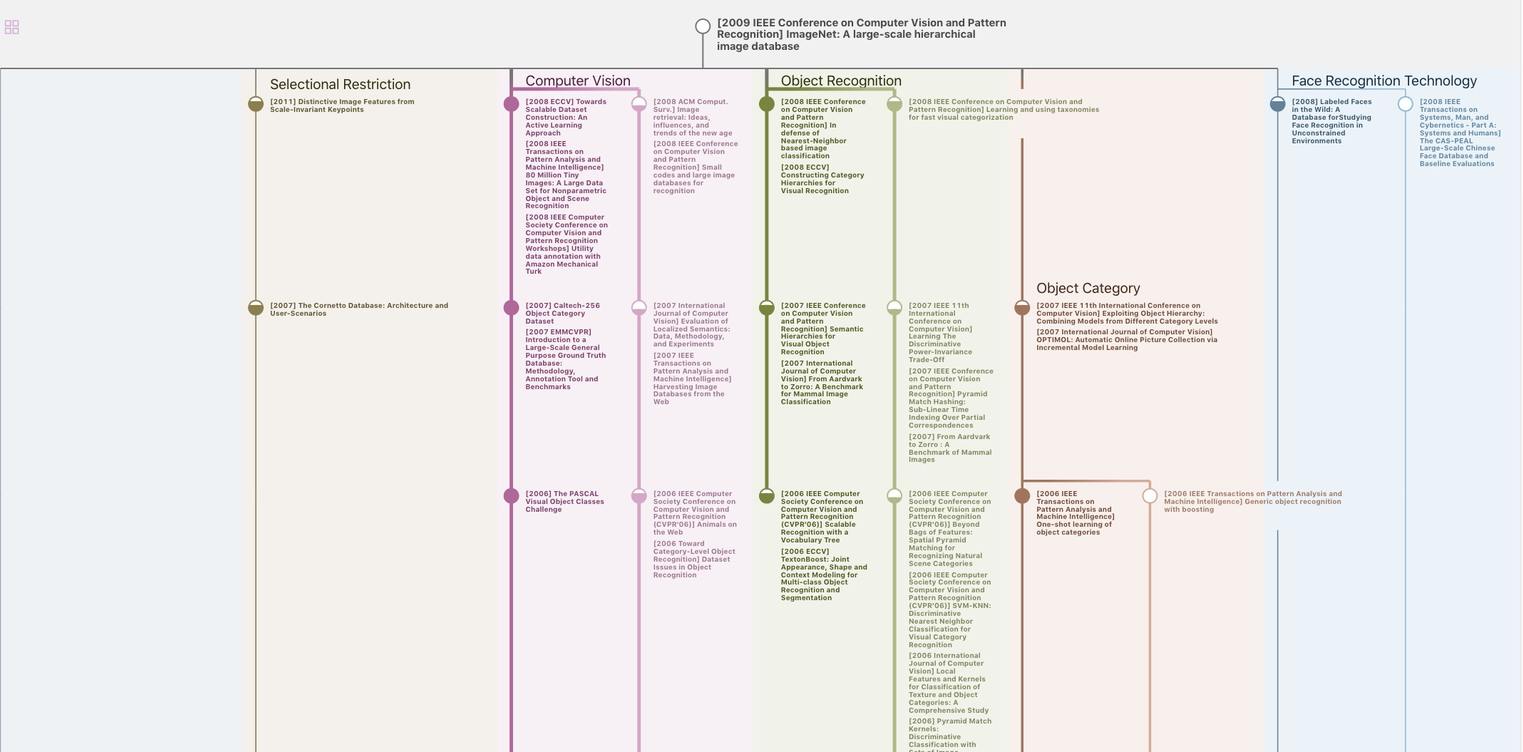
生成溯源树,研究论文发展脉络
Chat Paper
正在生成论文摘要