Adversarial Attack Detection Based on Example Semantics and Model Activation Features.
DSIT(2022)
摘要
With the widespread application of deep learning technology, its security issue is also gradually paid attention to. To improve the security and reliability of deep learning technology in practical applications, we focus on the vulnerability of deep neural networks against adversarial attacks and address the problems of existing adversarial example detection algorithms that rely on pre-known attack types, and low detection efficiency, and high detection costs. In this paper, we propose an adversarial attack detection method based on sample semantics and model activation features to provide an effective solution sample semantics for the problems of attack dependence and uninterpretable detection results of detection methods. Firstly, normal examples are input into the deep model to obtain the semantic features and model activation features. Secondly, binary classification datasets are constructed separately to train the binary classifier and then divides by the binary classification detector for we construct the adversarial samples in two parts based on relevance features and model activation features to achieve the detection of adversarial samples. Finally, in the experiments, the detection rate against different attacks under different datasets reached higher than 93.00%. At the same time, the detection algorithm in this paper still maintains high performance in the case of the attacker's known detection algorithm, the average detection rate decreases by no more than 5%.
更多查看译文
关键词
adversarial attack detection,model activation features,example semantics
AI 理解论文
溯源树
样例
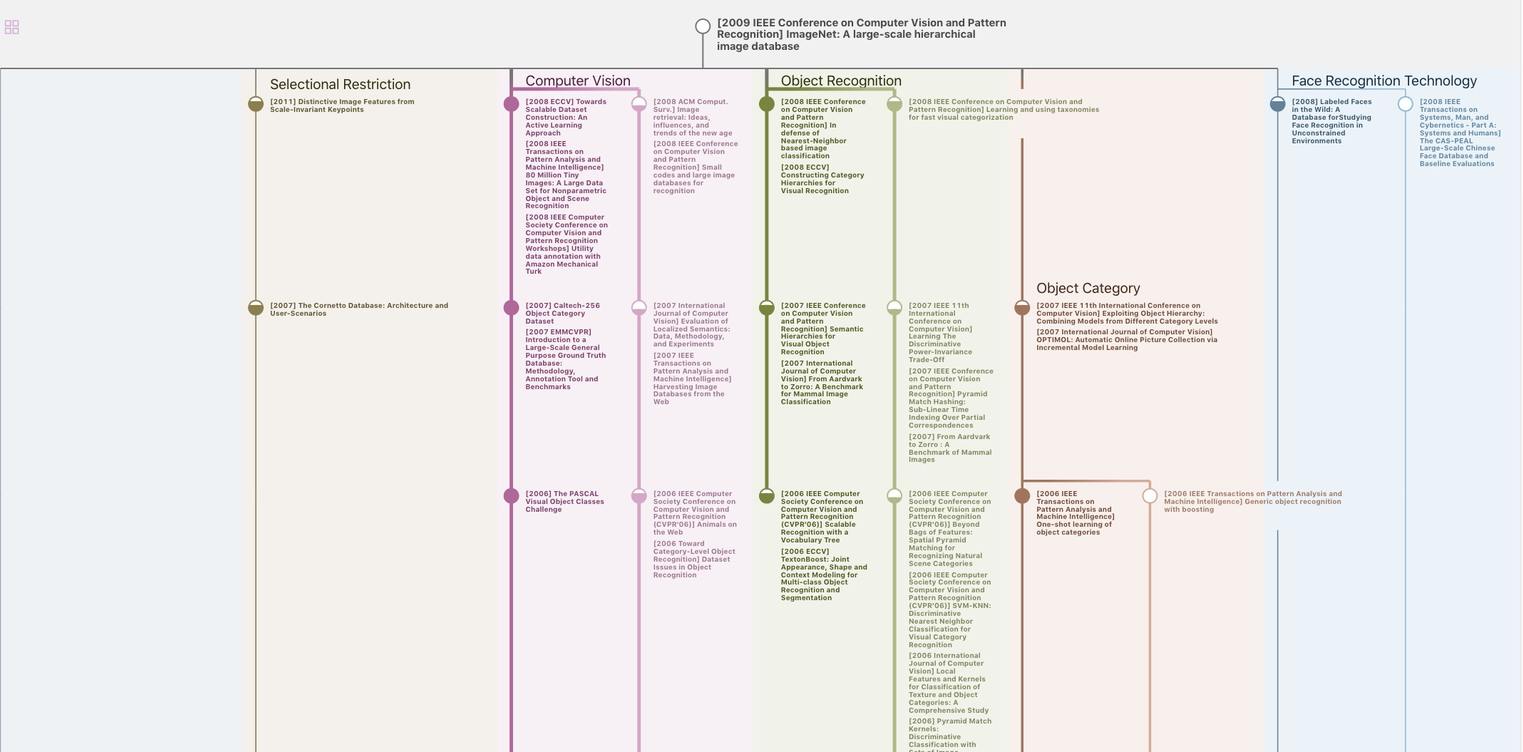
生成溯源树,研究论文发展脉络
Chat Paper
正在生成论文摘要