Multiphysiological Shallow Neural Network-Based Mental Stress Detection System for Wearable Environment.
ISCAS(2022)
摘要
Health problems related to stress are increasing globally and significantly affect the mental health and quality of life of human beings. Continuous suffering from stress may lead to serious psychological and physical health problems. But still, no effective and reliable stress detection methods are available. In this paper, a novel wearable device is presented to measure electroencephalogram (EEG) and electrocardiogram (ECG) simultaneously in a non-invasive approach. This system includes an analog front end (AFE) integrated with a machine learning-based digital backend (DBE) processor for mental stress prediction using only 3 electrodes. A PCB prototype is developed using the commercial off-the-shelf components. The developed prototype shows excellent noise performance of $0.1\mu V_{rms}$ and predicts the mental stress with a classification accuracy of 92.7%. The proposed system is lightweight and easily wearable (behind the ear). The data is acquired from 25 participants for different stress scenarios including the Arithmetic Test and Stroop Color Word Test. Different EEG and ECG based features combinations are used for the classification of stress conditions using a shallow neural network (SNN) classifier.
更多查看译文
AI 理解论文
溯源树
样例
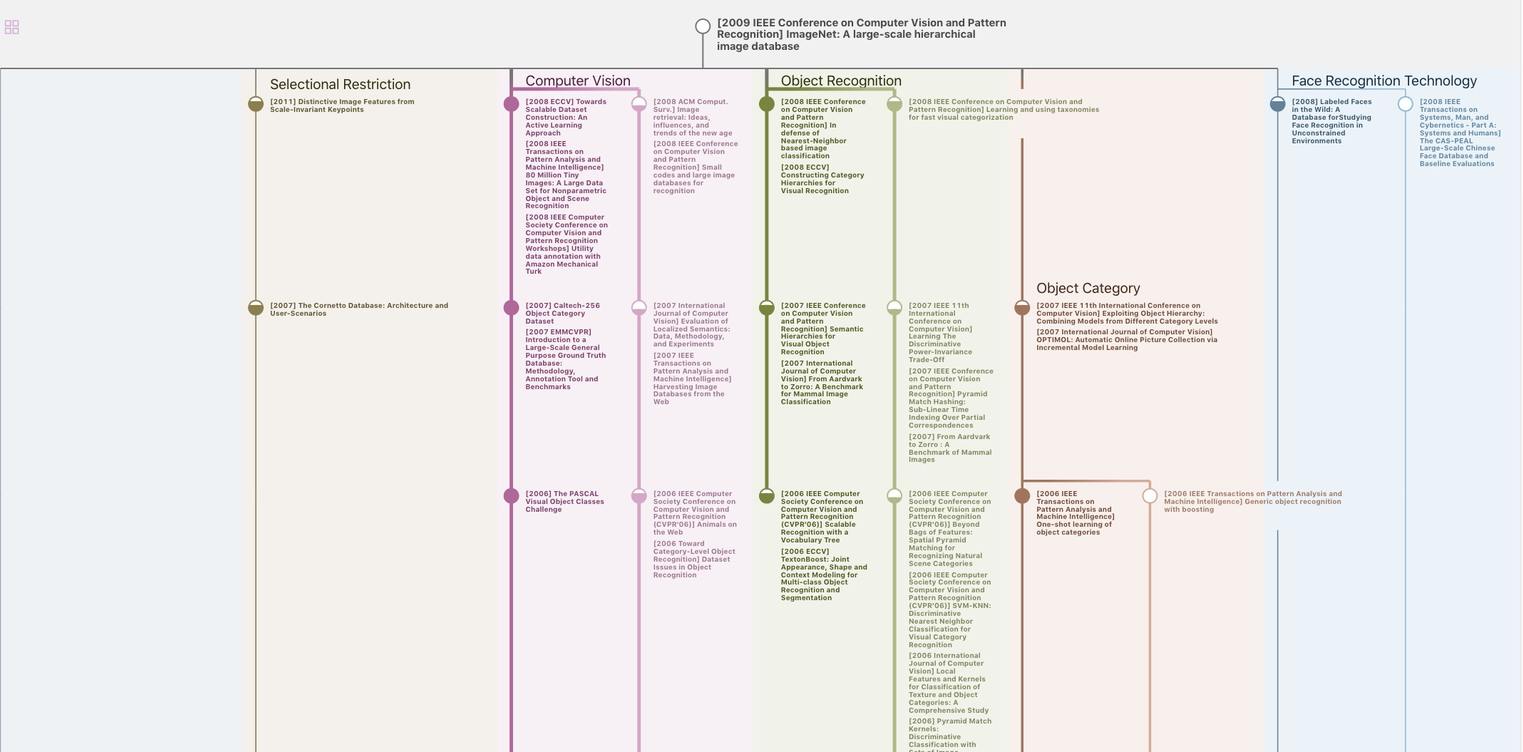
生成溯源树,研究论文发展脉络
Chat Paper
正在生成论文摘要