A 11.6μ W Computing-on-Memory-Boundary Keyword Spotting Processor with Joint MFCC-CNN Ternary Quantization
2022 IEEE International Symposium on Circuits and Systems (ISCAS)(2022)
摘要
This paper presents an ultra-low-power keyword spotting processor using an algorithm-architecture co-design approach. Joint MFCC-CNN ternary weight quantization is proposed to reduce power consumption. The Mel filter and the DCT module are merged into one matrix multiplication. The merged coefficients and the weights of the rest NN classifier are ternary-quantized, causing less than 3% accuracy loss but 39 × energy efficiency improvement. Moreover, a Computing-on-Memory-Boundary macro is adopted to store the quantized coefficients and weights, and perform matrix multiplications. Compared to the existing computing-in-memory technology, the proposed technique can reduce power consumption due to the higher utilization ratio. To verify the proposed techniques, a keyword spotting processor prototype is designed with 28nm CMOS technology. Simulation results show that the prototype achieves power consumption of 11.6μ W under a power supply of 0.72V and a clock frequency of 250KHz.
更多查看译文
关键词
Keyword Spotting,Computing on Memory Boundary,Joint Quantization,Ultra-low Power,Ternary Weight Neural-Networks
AI 理解论文
溯源树
样例
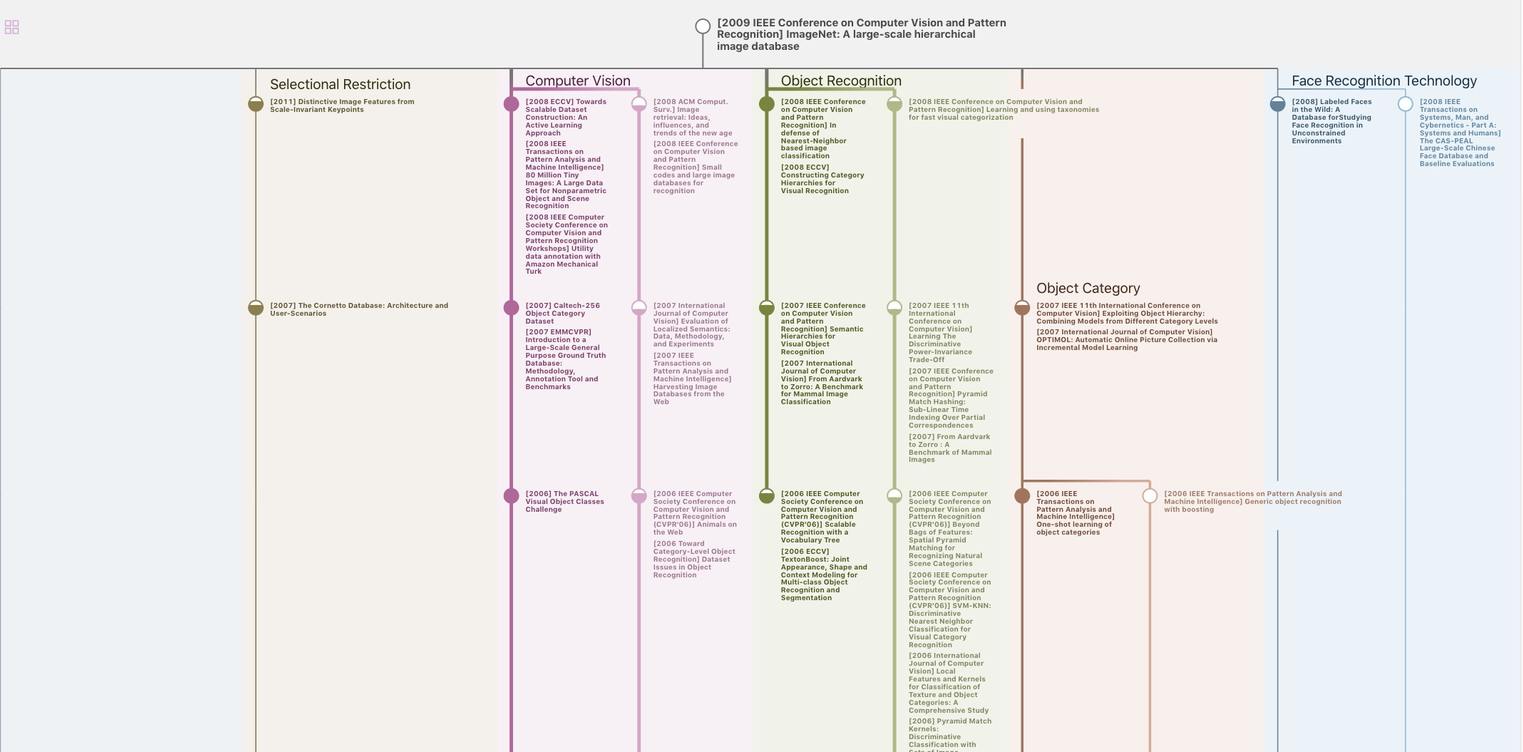
生成溯源树,研究论文发展脉络
Chat Paper
正在生成论文摘要