Learning the Robust and Structured Control of Unknown Linear Systems.
ACC(2022)
摘要
In many control systems in practice, the control input is subject to some specific structure and affected by noise. Designing control laws to stabilize these systems is challenging, especially when the system model is uncertain. This paper will solve this problem for linear systems with unknown system state matrix. First, a model-based framework is formulated to embed the structural control constraint and input noise into the linear quadratic regulator (LQR) setting to find the stabilizing control. Then, this model-based LQR setting is transformed into a databased learning framework, robust structured reinforcement learning (RSRL), to cope with the unknown system state matrix. As a result, the data-based control can stabilize the unknown system with input noise, while obeying the structural constraint. Theoretical results will be validated on a multi-agent network with 6 agents.
更多查看译文
关键词
Reinforcement Learning,Structured Learning,Distributed Control,Stability Guarantee,Robust Learning,Linear Quadratic Regulator
AI 理解论文
溯源树
样例
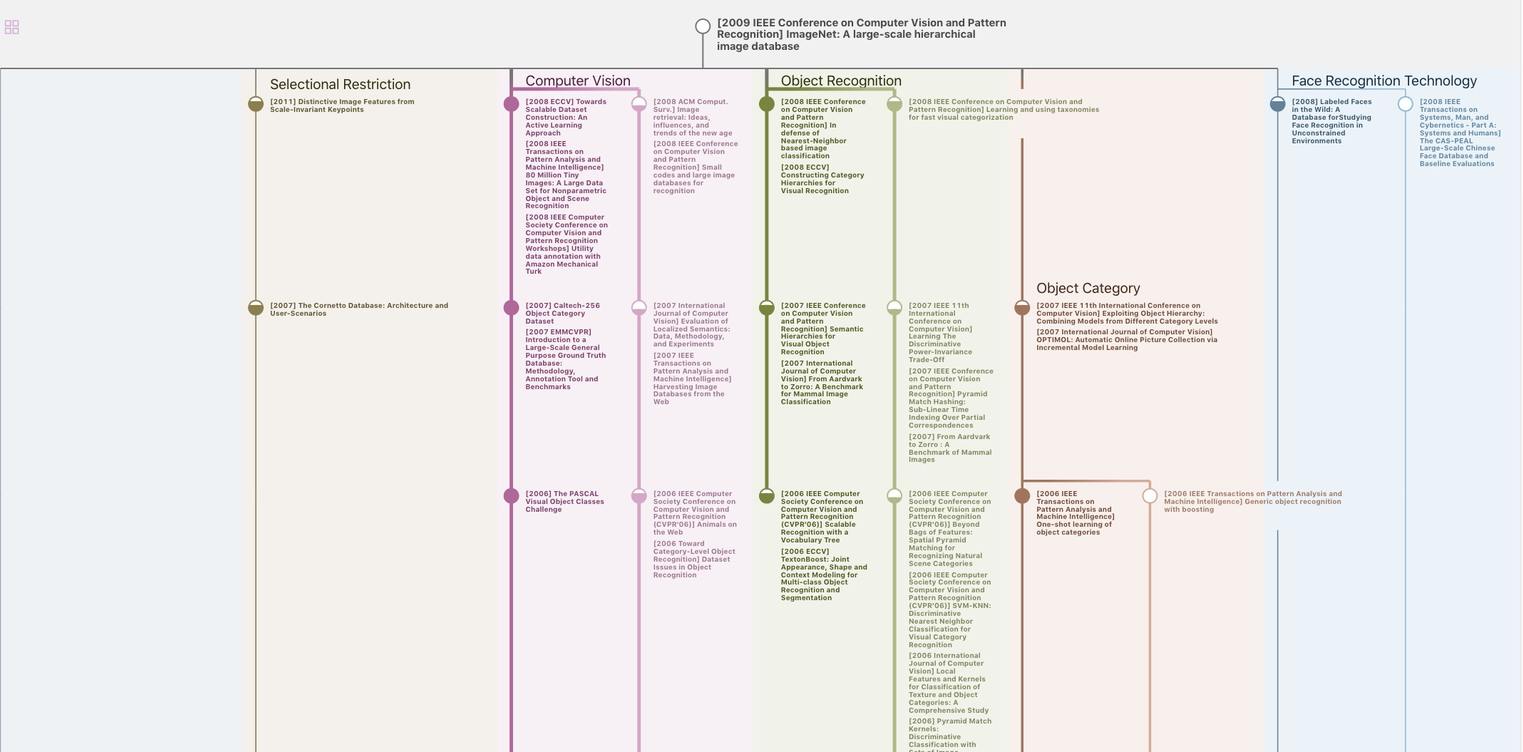
生成溯源树,研究论文发展脉络
Chat Paper
正在生成论文摘要