Learning-based Adaptive-Scenario-Tree Model Predictive Control with Probabilistic Safety Guarantees Using Bayesian Neural Networks
ACC(2022)
摘要
This paper proposes a learning-based adaptive-scenario-tree model predictive control (MPC) approach with probabilistic safety guarantees using Bayesian neural networks (BNNs) for nonlinear systems. First, a data-driven description of the model uncertainty (i.e., plant-model mismatch) is learned using a BNN. Then, the learned description is employed to generate adaptive scenarios online for scenario-based MPC (sMPC). To accurately represent the evolution of uncertainties, we use a moment-matching method to compute the probabilities of the generated time-varying scenarios. Moreover, probabilistic safety guarantees are provided by ensuring that the trajectories of the scenarios contain the real trajectory of the system and all the generated scenarios satisfy the constraints with a high probability. By realizing a less conservative estimation of the model uncertainty, the proposed approach can improve robust control performance with respect to sMPC with a fixed scenario tree. Closed-loop simulations on a cold atmospheric plasma system with prototypical applications in (bio)materials processing demonstrate that the proposed approach results in an improved control performance compared to sMPC with a fixed scenario tree.
更多查看译文
关键词
time-varying scenarios,probabilistic safety guarantees,model uncertainty,fixed scenario tree,Bayesian neural networks,data-driven description,plant-model mismatch,learned description,adaptive scenario-based MPC,learning-based adaptive-scenario-tree model predictive control,robust control,nonlinear systems,moment-matching method
AI 理解论文
溯源树
样例
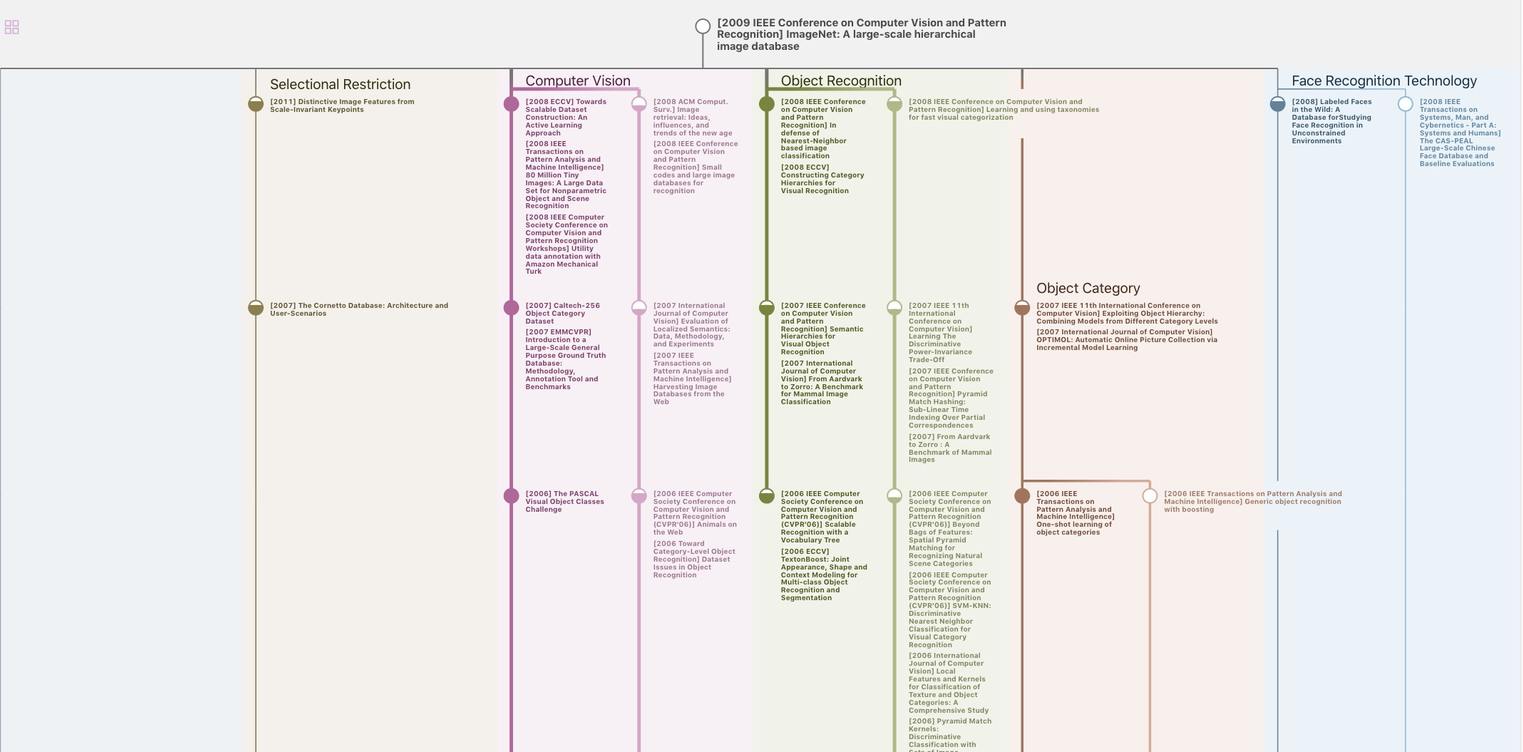
生成溯源树,研究论文发展脉络
Chat Paper
正在生成论文摘要