Multi-task Learning-based Text Classification with Subword-Phrase Extraction
SoICT(2022)
摘要
Text classification using deep learning, which is trained with a tremendous amount of text, has achieved superior performance than traditional methods. In addition to its success, multi-task learning has become a promising approach for text classification; for instance, a multi-task learning approach employs named entity recognition as an auxiliary task for text classification. The existing MTL-based text classification methods depend on auxiliary tasks using supervised labels, which require large human and/or financial efforts to create. To reduce these efforts, this paper proposes a multi-task learning-based text classification framework which reduces the additional efforts on supervised label creation. A basic idea to realize this is that to utilize phrasal expressions consisting of subwords (called subword-phrase). To the best of our knowledge, there has been no text classification approach on top of subword-phrases, because subwords do not always express a coherent set of meanings. The proposed framework is new to add subword-phrase recognition as an auxiliary task, and to utilize subword-phrases for text classification. To realize the low-cost auxiliary recognition task, the framework extracts subword-phrases in an unsupervised manner. The experimental evaluation of the five popular datasets for text classification showcases the effectiveness of the involvement of the subword-phrase recognition as an auxiliary task. It also shows comparative results with the state-of-the-art method.
更多查看译文
AI 理解论文
溯源树
样例
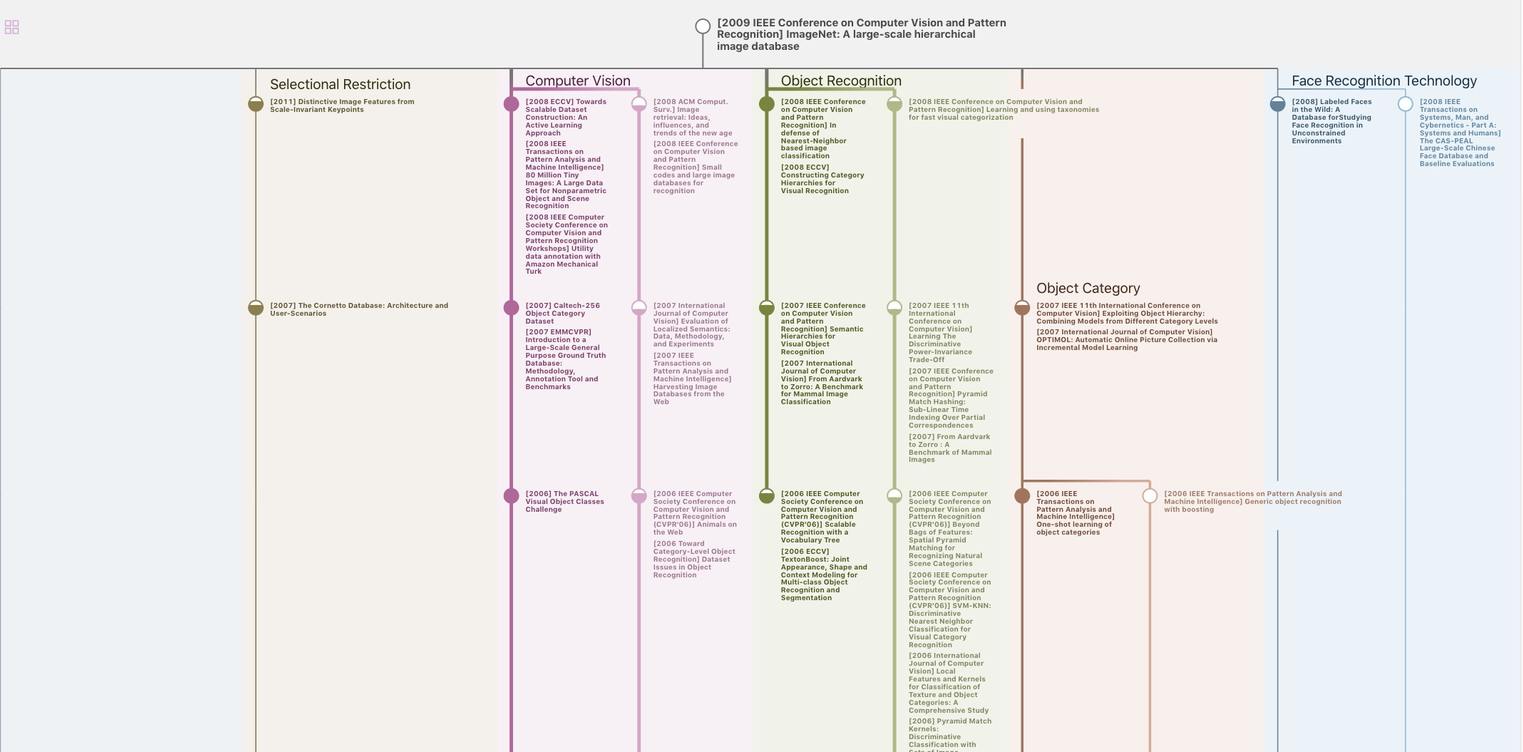
生成溯源树,研究论文发展脉络
Chat Paper
正在生成论文摘要