Various Distance Metrics Evaluation on Neural Spike Classification.
BioCAS(2022)
摘要
State-of-the-art neuroscience applications require the classification of action potential activities (or spikes) recorded by multi-channel electrodes, named spike sorting. A typical spike sorting algorithm involves three parts: detection, feature extraction, and training/classification. Training/classification stages are mainly based on distance-based algorithms like K-means. For the classification in particular, the accuracy depends highly on the distance metric performance. In this paper, we analyze several distance metrics to classify various datasets with different noise levels using the F1-score. This includes Manhattan, Euclidean and, Mahalanobis distance metrics. As a result, the Mahalanobis distance metric outperforms other metrics in noisy conditions by an average of 10% in the presence of the noise.
更多查看译文
关键词
neural spike classification,distance
AI 理解论文
溯源树
样例
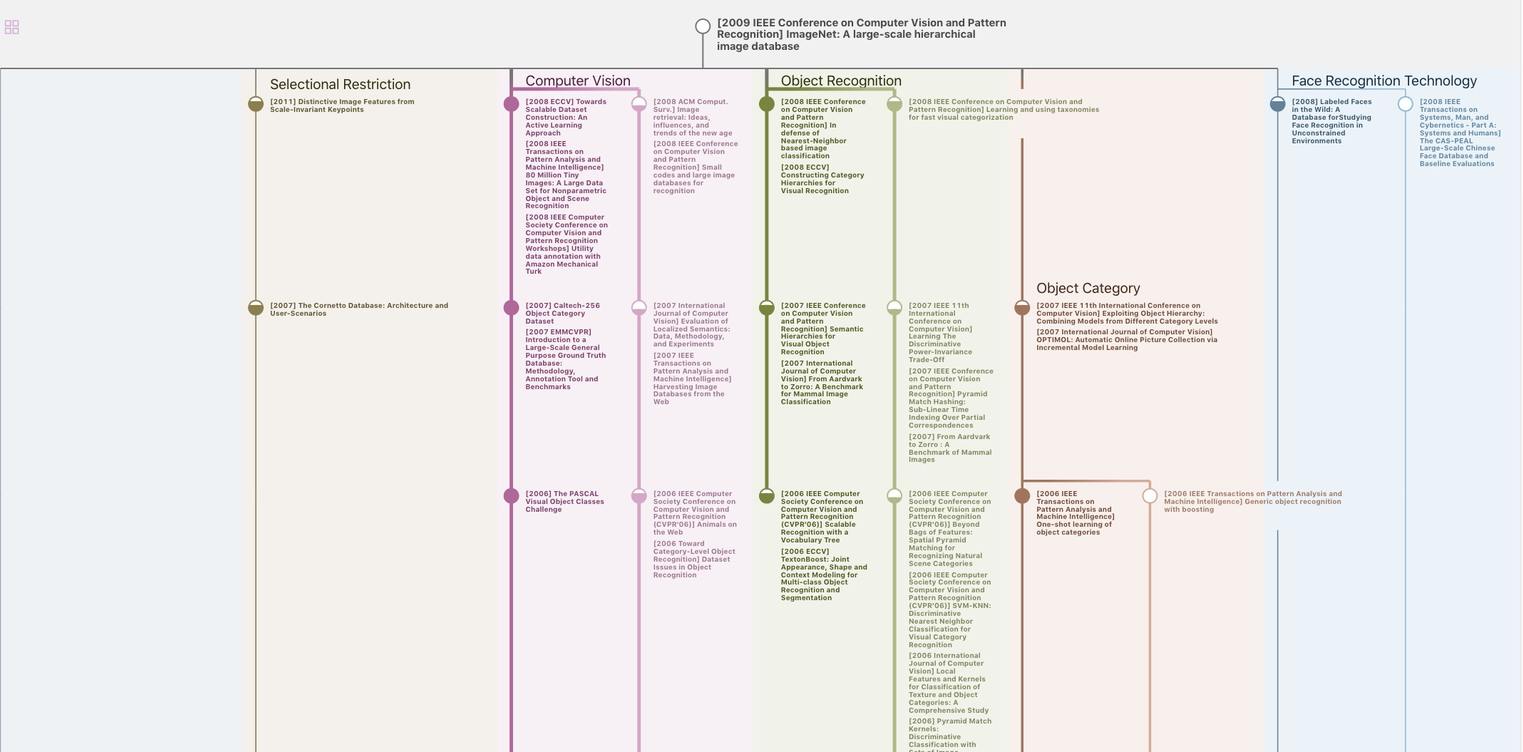
生成溯源树,研究论文发展脉络
Chat Paper
正在生成论文摘要