Performance Assessment of Automatic Sleep Stage Classification Using Only Partial PSG Sensors.
BioCAS(2022)
摘要
As sleep disorders are becoming overwhelmingly prevalent in industrialized countries, a simple, readily accessible sleep stage classification technology is strikingly at need. Conventional sleep stage classification relies on sensors such as electroencephalogram (EEG), electrooculogram (EOG), and electromyogram (EMG). However, such sensors are costly and exceedingly inconvenient to utilize. By employing deep neural network technology for sleep stage classification with polysomnography (PSG), we studied how much loss in accuracy incurred when eliminating specific signals in PSG. We compared the performance of Transformer with those of fully-connected deep neural network and recurrent neural network models. Especially, we aim to eliminate EEG sensors as they are the most cumbersome to use. This experiment demonstrates the possibility and limitations of simple sleep stage classification that does not rely on EEG signals.
更多查看译文
关键词
Sleep stage classification,Polysomnography
AI 理解论文
溯源树
样例
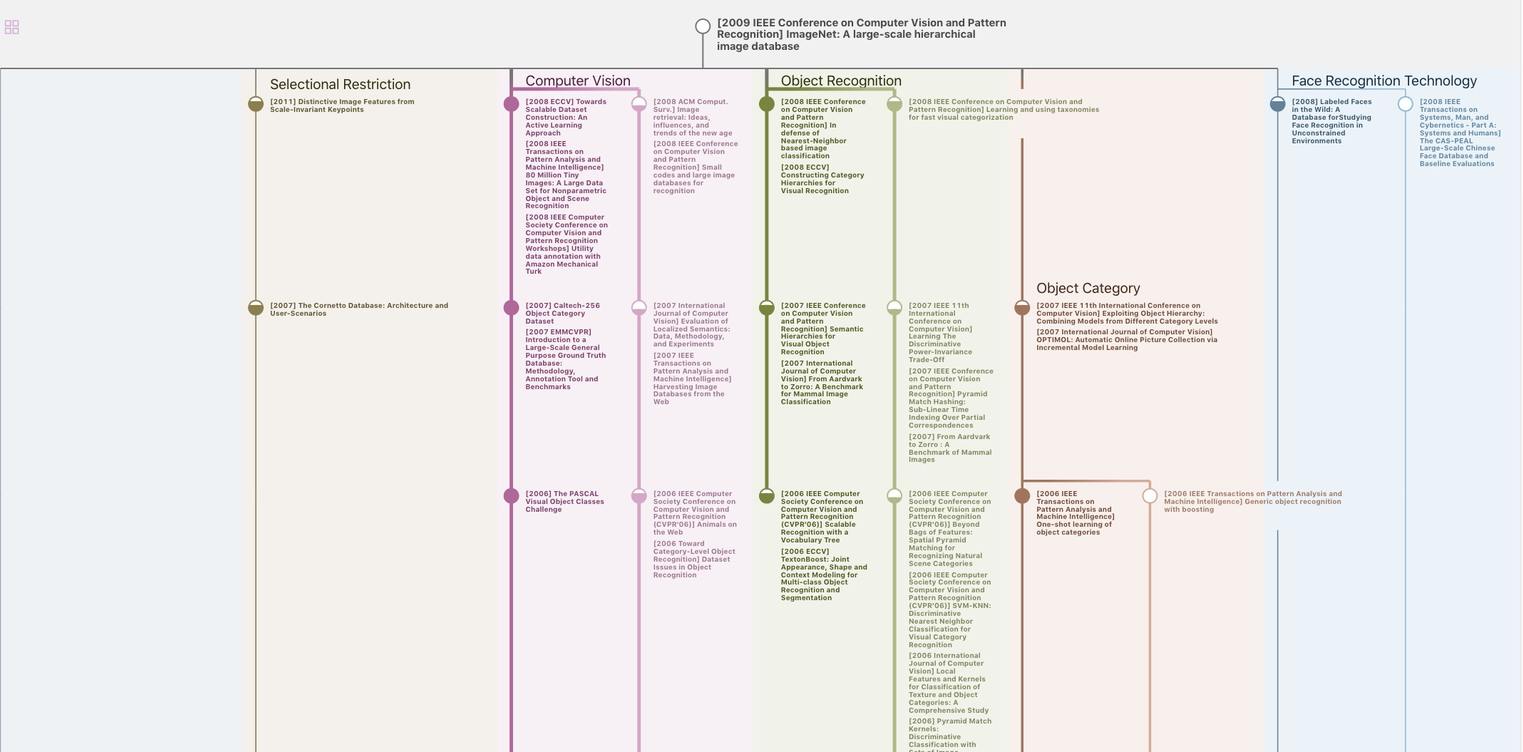
生成溯源树,研究论文发展脉络
Chat Paper
正在生成论文摘要