Systematic Monotonicity and Consistency for Adversarial Natural Language Inference.
AI(2022)
摘要
Natural Language Inference is a fundamental task required for understanding natural language. With the introduction of large Natural Language Inference (NLI) benchmark datasets such as SNLI and MultiNLI, NLI has seen an uptake in models achieving near-human accuracy. Deeper analyses through adversarial methods performed on these models however have cast doubts on their ability to actually understand the inference process. In this work, we attempt to define a principled way to generate adversarial attacks based on monotonic reasoning and consistency to examine their language understanding abilities. We show that the language models trained for general tasks have a poor understanding of monotonic reasoning. For this purpose, we provide methods to generate an adversarial dataset from any NLI dataset based on monotonicity and consistency principles and conduct extensive experiments to support our hypothesis. Our adversarial datasets preserve these crucial aspects of monotonicity, consistency and semantic similarity and are still able to fool a model finetuned on SNLI 79% of the time while preserving semantic similarity to a much greater extent than previous methods.
更多查看译文
关键词
natural language,consistency
AI 理解论文
溯源树
样例
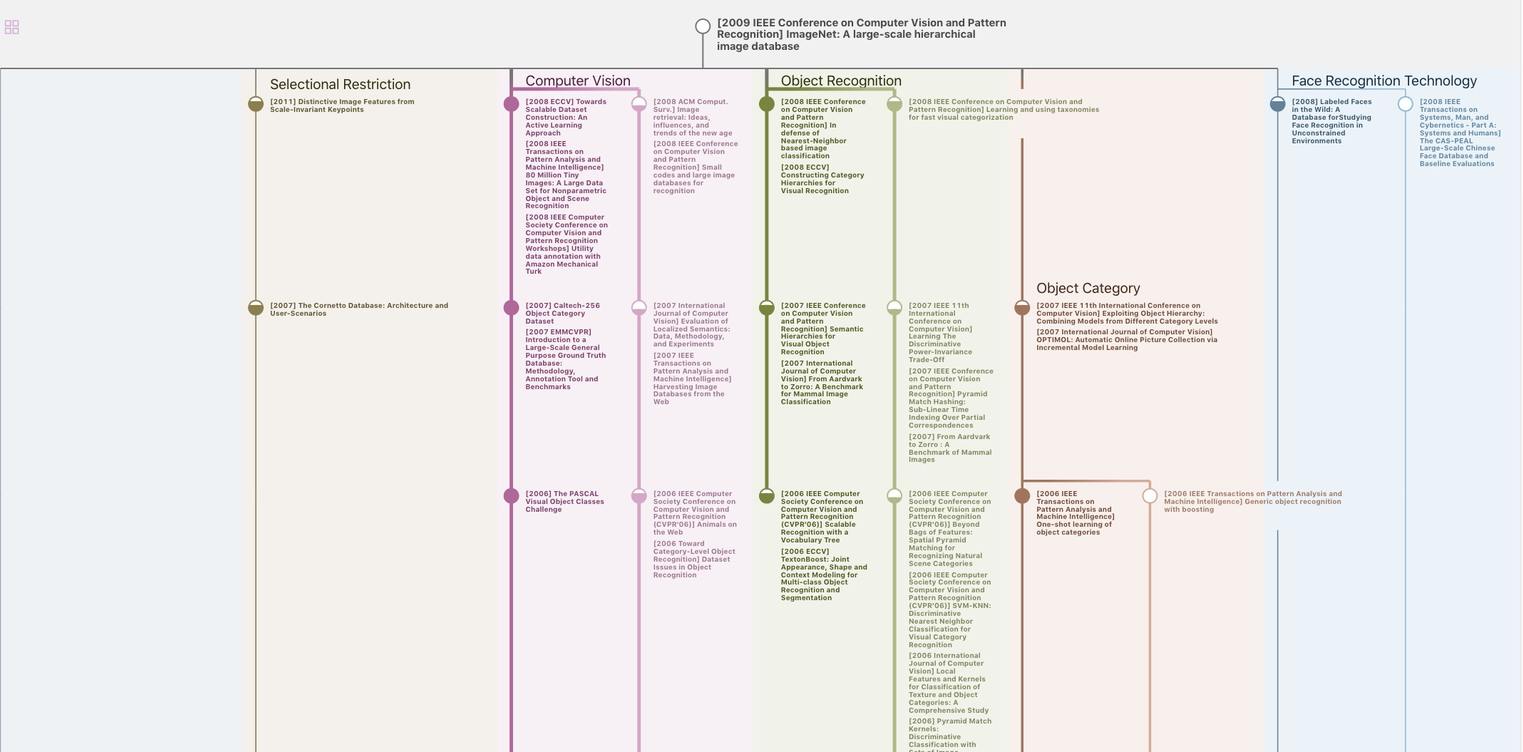
生成溯源树,研究论文发展脉络
Chat Paper
正在生成论文摘要