Boosted Self-evolving Neural Networks for Pattern Recognition.
AI(2022)
摘要
It has been well documented that both boosting and bagging algorithms improve ensemble performance. However, these types of algorithms have only infrequently been applied to ensembles of constructivist learners which are based on neural networks. Although there have been previous attempts at developing similar ensemble learning algorithms for constructivist learners, our proposed approach also addresses the issue of ensuring more diversity of the learners in the ensemble and offers a different approach for handling imbalanced data sets. More specifically, this paper investigates how a modified version of the AdaBoost algorithm can be applied to generate an ensemble of simple incremental learning neural network-based constructivist learners known as the Self-Evolving Connectionist System (SECoS). We develop this boosting algorithm to leverage the accurate learning of the SECoS and to promote diversity in these SECoS learners in order to create an optimal model for classification tasks. Moreover, we adopt a similar minority class sampling method inspired by RUSBoost which addresses the class imbalance problem when learning from data. Our proposed AdaBoostedSECoS (ABSECoS) learning framework is compared with other ensemble-based methods using four benchmark data sets, three of which have class imbalance. The results of these experiments suggest ABSECoS performs comparably well against similar ensemble methods using boosting techniques.
更多查看译文
关键词
Ensemble learning, Adaptive systems, Neural networks
AI 理解论文
溯源树
样例
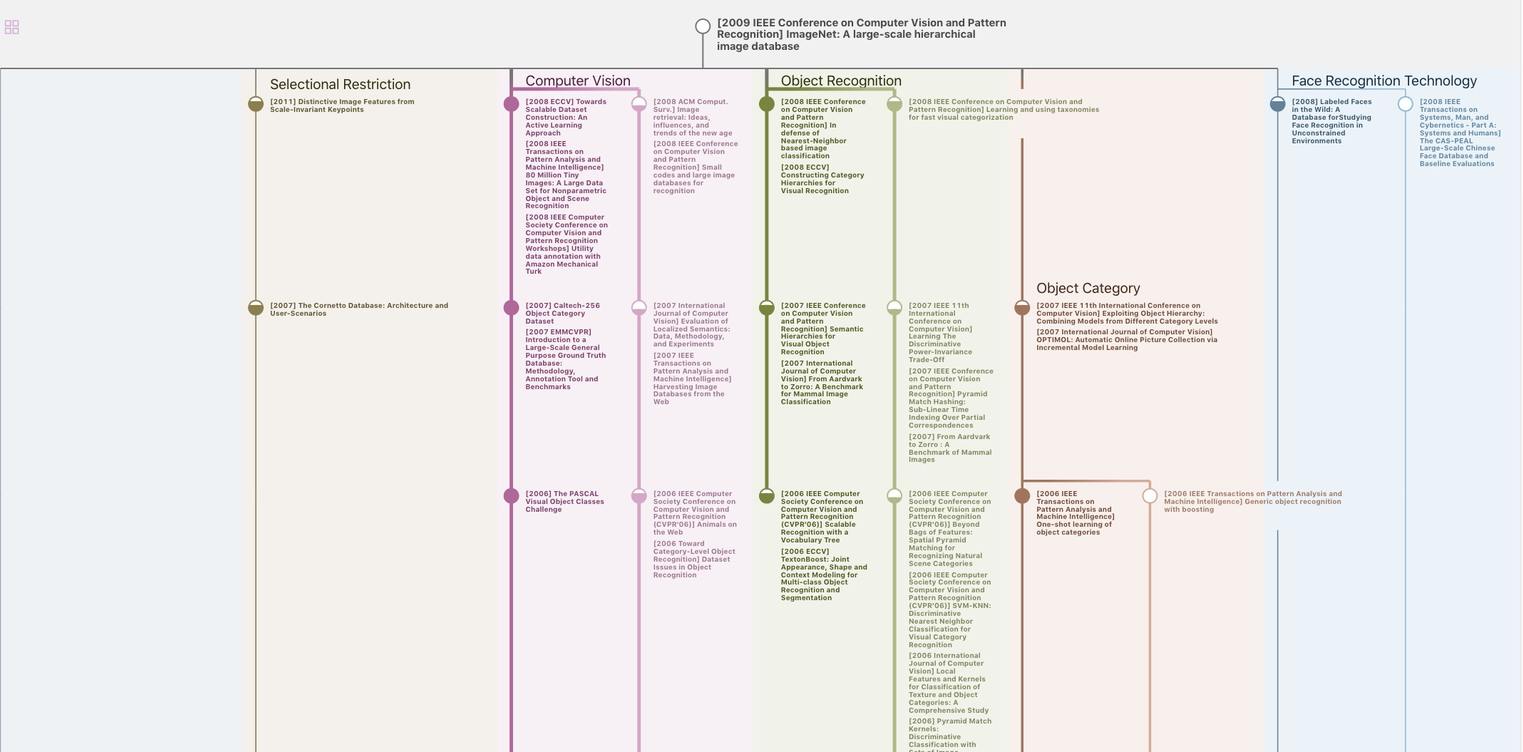
生成溯源树,研究论文发展脉络
Chat Paper
正在生成论文摘要