Impact of Mathematical Norms on Convergence of Gradient Descent Algorithms for Deep Neural Networks Learning.
AI(2022)
摘要
To improve the performance of gradient descent learning algorithms, the impact of different types of norms is studied for deep neural network training. The performance of different norm types used on both finite-time and fixed-time convergence algorithms are compared. The accuracy of the multiclassification task realized by three typical algorithms using different types of norms is given, and the improvement of Jorge's finite time algorithm with momentum or Nesterov accelerated gradient is also studied. Numerical experiments show that the infinity norm can provide better performance in finite time gradient descent algorithms and give strong robustness under different network structures.
更多查看译文
关键词
gradient descent algorithms,deep neural networks,mathematical norms,neural networks,learning
AI 理解论文
溯源树
样例
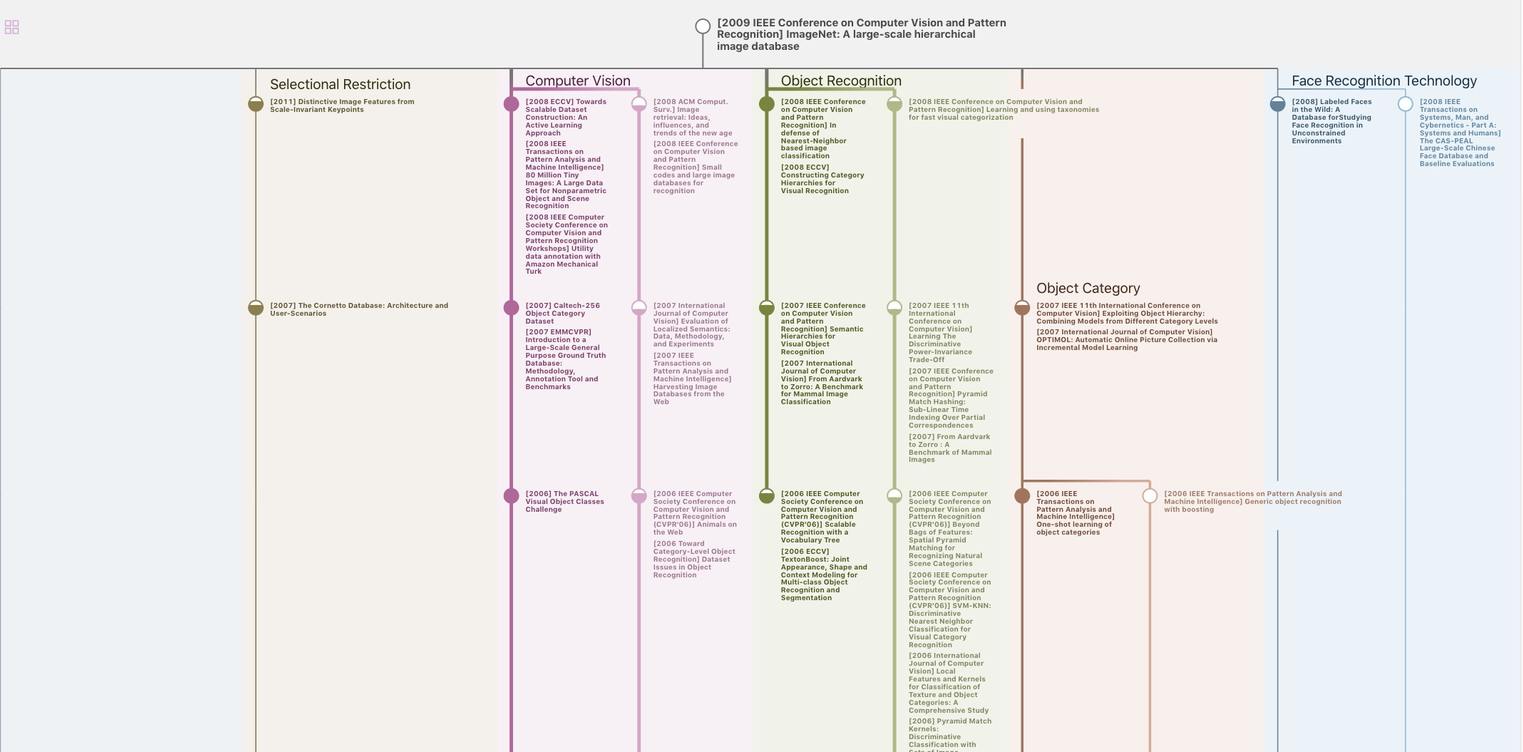
生成溯源树,研究论文发展脉络
Chat Paper
正在生成论文摘要