Automatic generation of areas of interest using multimodal geospatial data from an on-demand food delivery platform (industrial paper)
SIGSPATIAL/GIS(2022)
摘要
On-demand food delivery (ODFD) is booming globally. ODFD services rely heavily on accurate Areas of Interest (AOIs) data to make effective operational decisions, such as service areas of restaurants. Few methods can automatically generate AOIs with contours highly matched to geographic boundaries, so manual labeling and auditing still play a vital role in data production. Most existing studies can merely generate approximate ranges which are not accurate enough for business usage. The others, which can generate AOIs fitting geographic boundaries through map matching methods, are limited by the deficiency of road network data, as geographic boundaries contain rivers, mountains, and so on. To address this issue, we propose a novel AOI generation framework using large-scale geospatial data to generate AOIs which are closer to geographic boundaries. In our framework, we firstly extract multimodal features from satellite images, road networks as well as delivery data (customer addresses, locations, etc.), and then use a semantic segmentation model to infer pixel-level points that possibly lie within the boundaries of AOIs. After that, a contour learning method and simple post-processing are applied to fit the discrete pixel-level points to reconstruct contours of AOIs in arbitrary shapes. Experiments were conducted aiming at comparing the proposed framework with six competing methods qualitatively and quantitatively. As a result, our proposed framework performs better in generating AOIs with accurate and geometry-preserving contours.
更多查看译文
关键词
multimodal geospatial data,areas,automatic generation,industrial paper,on-demand
AI 理解论文
溯源树
样例
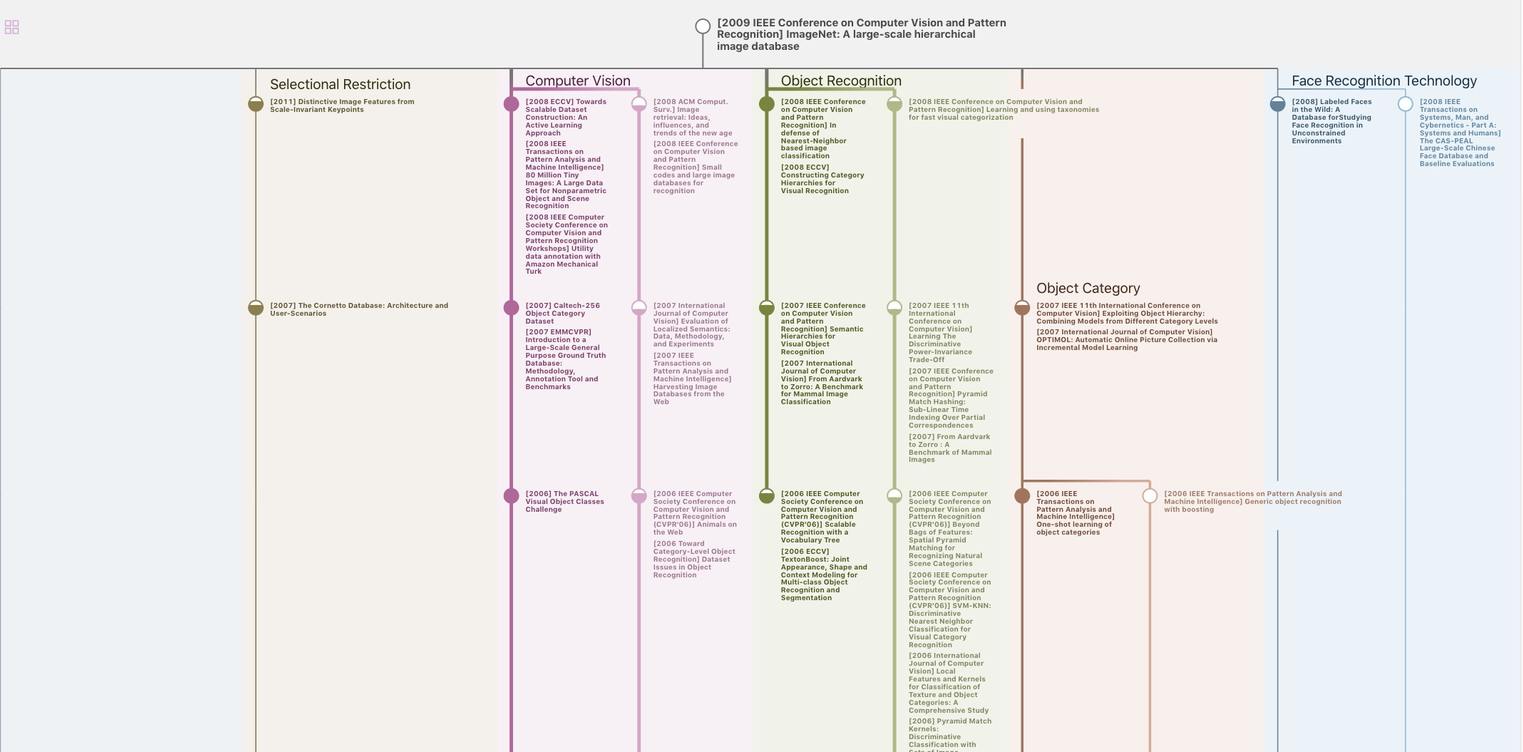
生成溯源树,研究论文发展脉络
Chat Paper
正在生成论文摘要