Unsupervised Discovery of Disentangled Interpretable Directions for Layer-Wise GAN.
Big Data (CCF)(2022)
摘要
Many studies have shown that generative adversarial networks (GANs) can discover semantics at various levels of abstraction, yet GANs do not provide an intuitive way to show how they understand and control semantics. In order to identify interpretable directions in GAN's latent space, both supervised and unsupervised approaches have been proposed. But the supervised methods can only find the directions consistent with the supervised conditions. However, many current unsupervised methods are hampered by varying degrees of semantic property disentanglement. This paper proposes an unsupervised method with a layer-wise design. The model embeds subspace in each generator layer to capture the disentangled interpretable semantics in GAN. And the research also introduces a latent mapping network to map the inputs to an intermediate latent space with rich disentangled semantics. Additionally, the paper applies an Orthogonal Jacobian regularization to the model to impose constraints on the overall input, further enhancing disentanglement. Experiments demonstrate the method's applicability in the human face, anime face, and scene datasets and its efficacy in finding interpretable directions. Compared with existing unsupervised methods in both qualitative and quantitative aspects, this study proposed method achieves excellent improvement in the disentanglement effect.
更多查看译文
关键词
Discovery of interpretable directions, Generative adversarial network, Unsupervised learning, Disentangled semantic
AI 理解论文
溯源树
样例
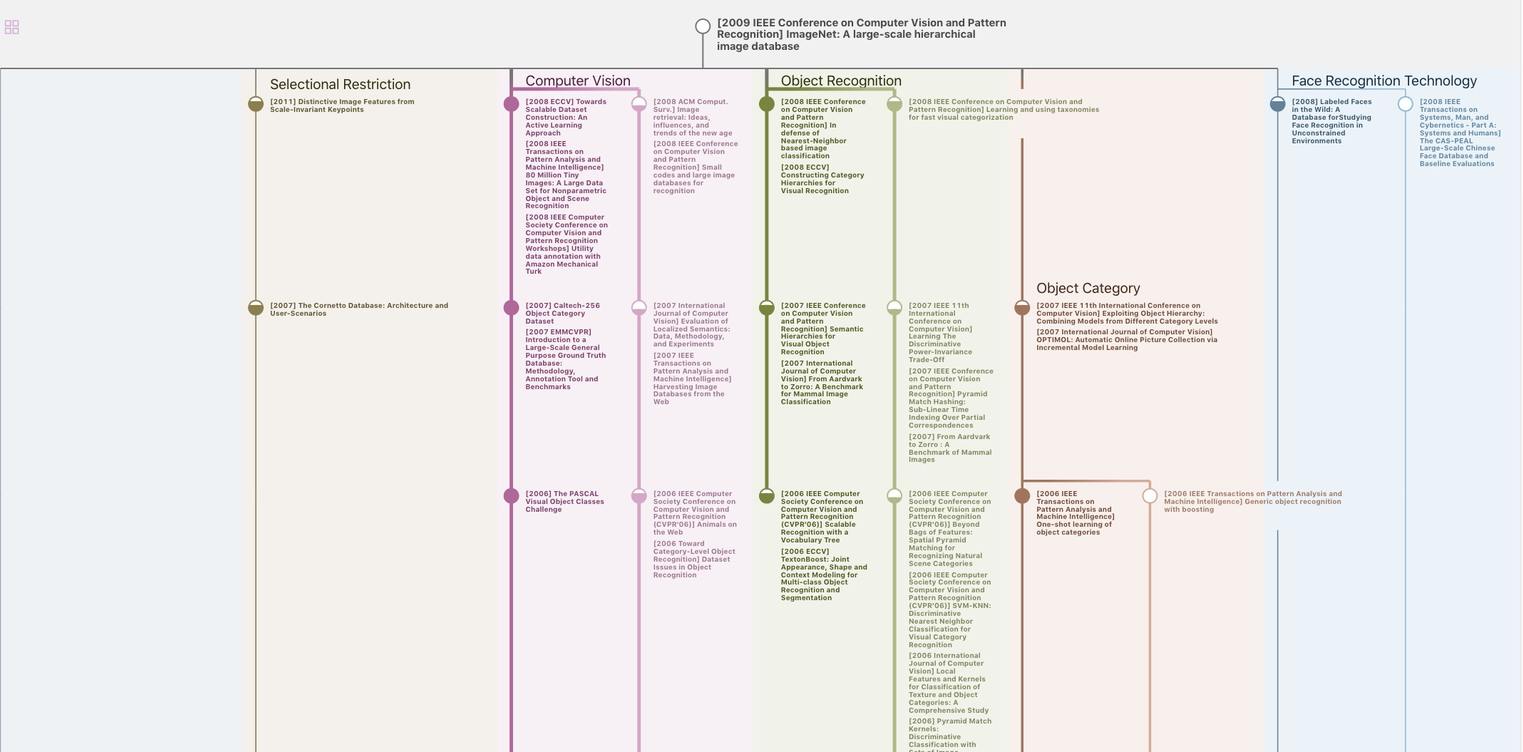
生成溯源树,研究论文发展脉络
Chat Paper
正在生成论文摘要