Effective Imbalance Learning Utilizing Informative Data.
AusDM(2022)
摘要
The class imbalance problem that is caused by unequal data distribution usually results in poor performance, and it has attracted increasing attention in the research community. The challenge of the problem is the difficulty to extract sufficient information from the minority class. As a result, the classifier converges to a sub-optimal state. While methods based on resampling and reweighing the cost for different classes are the common strategies to address the problem, there are still numerous issues with these methods such as under- or over-sampling that may remove necessary information or introduce noise, respectively; and reweighing may result in an inappropriate cost matrix. To address the above shortcomings, in this paper, an enhanced approach based on informative samples is proposed. In our approach, the classifier can indicate which class a sample is closer to by comparing it with boundary samples. The informative samples include the samples from both positive and negative samples located around the boundary. Finally, our experiments show that our proposed method outperforms state-of-the-art algorithms by 18% on F-1 score.
更多查看译文
关键词
Imbalance learning, Informative data
AI 理解论文
溯源树
样例
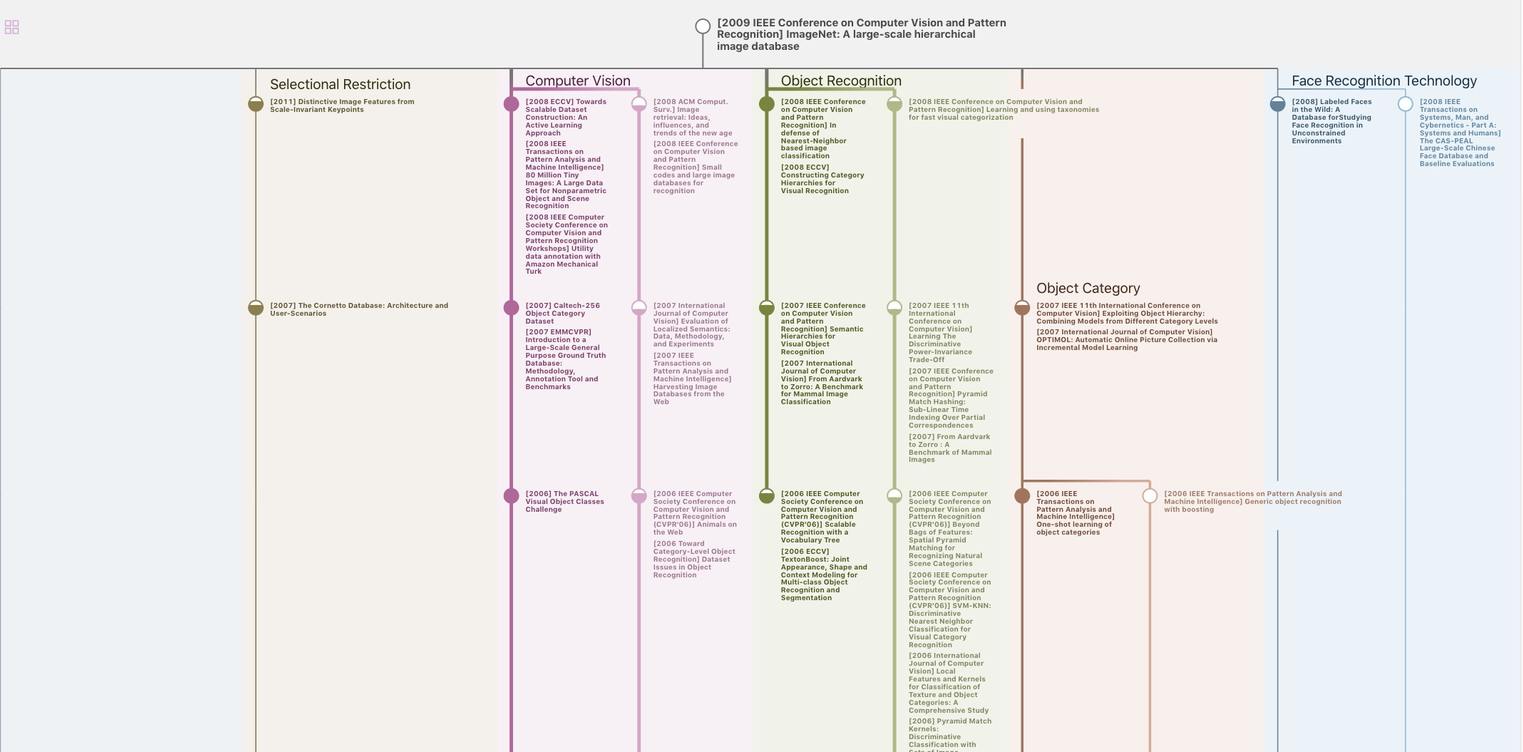
生成溯源树,研究论文发展脉络
Chat Paper
正在生成论文摘要