Chinese Text Classification Using BERT and Flat-Lattice Transformer.
AIMS(2022)
摘要
Recently, large scale pre-trained language models such as BERT and models with lattice structure that consisting of character-level and word-level information have achieved state-of-the-art performance in most downstream natural language processing (NLP) tasks, including named entity recognition (NER), English text classification and sentiment analysis. For Chinese text classification, the existing methods have also tried such kinds of models. However, they cannot obtain the desired results since these pre-trained models are based on characters, which cannot be applied for Chinese language that is based on words. To address this problem, in this paper, we propose BFLAT which a simple but efficient model for Chinese text classification. Specifically, BFLAT utilizes BERT and word2vec to learn character-level and word-level vector representations, and then adopts the flat-lattice transformer to integrate both of the two-level vector representations. Experimental results on two datasets demonstrate that our proposed method outperforms the baseline methods over 1.38–21.82% and 3.42–20.7% in terms of relative F1-measure on two Chinese text classification benchmarks, respectively.
更多查看译文
AI 理解论文
溯源树
样例
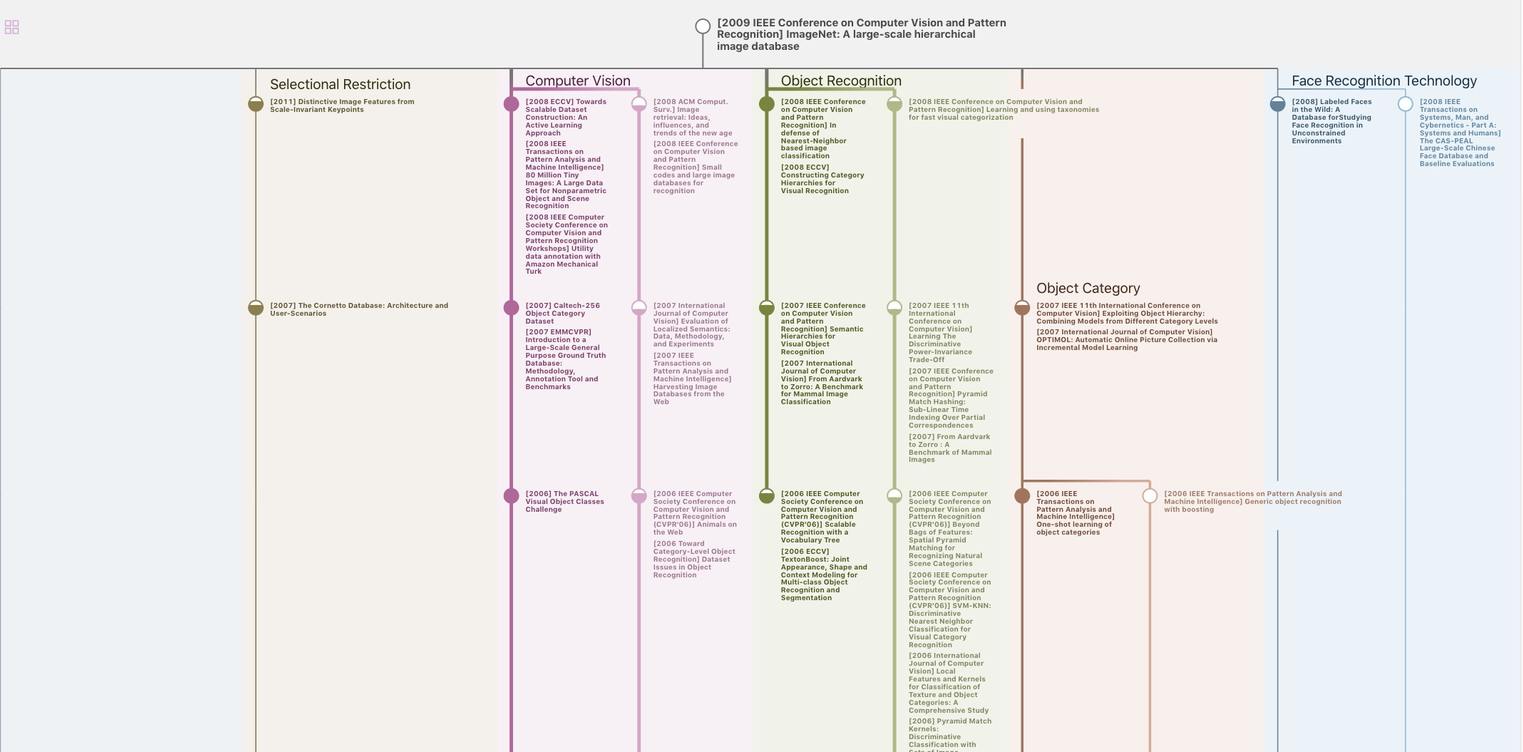
生成溯源树,研究论文发展脉络
Chat Paper
正在生成论文摘要