Time series prediction in IoT: a comparative study of federated versus centralized learning.
BRACIS (2)(2023)
摘要
Temporal data collected by IoT sensors can be used to feed predictive algorithms constantly. This allows the construction of applications that, for example, are used to predict CO2 indices in a given region, whose results can help in the prototyping of solutions aimed at health. In this context, in order to find out which are the best solutions for predicting time series generated from data collected by IoT devices, two machine learning approaches were compared: Federated learning and centralized learning. Federated learning implies training the algorithms in a distributed way across devices, while centralized learning takes data from devices into a server and focuses training on it. We used a Long Short Term Memory (LSTM) to predict the time series. Experiments were performed using LSTM with both federated and centralized strategies. The results show that the Federated Learning model predicts five time-steps of a time series with on average 78% less mean squared error than a Centralized solution.
更多查看译文
关键词
Centralized Learning,Federated Learning,LSTM,Time Series
AI 理解论文
溯源树
样例
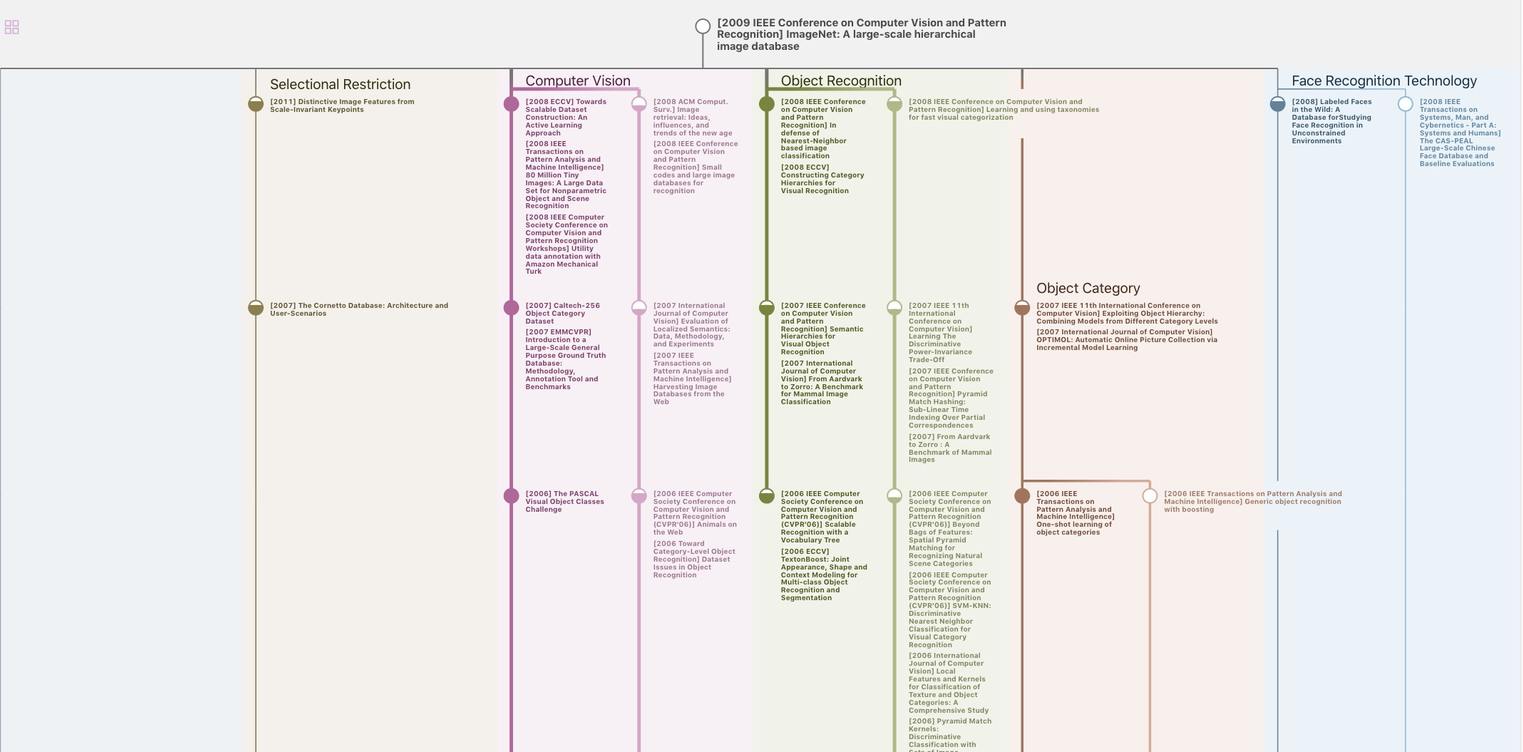
生成溯源树,研究论文发展脉络
Chat Paper
正在生成论文摘要