The Effects of Under and Over Sampling in Exoplanet Transit Identification with Low Signal-to-Noise Ratio Data.
BRACIS (1)(2022)
摘要
This paper presents the results of experiments with undersampling and oversampling applied to machine learning classifiers used in the identification of exoplanet transits with low signal-to-noise ratio (SNR) data. We start by giving an overview of the most popular method for exoplanet detection, followed by an analysis of the Kepler Object of Interest (KOI) data set, along with an overview of the state of the art machine learning models applied to this problem, and how complex it is to correctly identify exoplanets on low SNR data. We then briefly discuss our signal-to noise ratio reduction procedure, used to generate the low SNR data for our experiments. Finally we use our low SNR data set to train and evaluate some models in scenarios with no sampling strategy and with oversampling and undersampling, using repeated holdout validation. Results show that current classifiers can identify transits in low SNR data sets, with accuracy varying between 69% and 81%, and that sampling strategies can affect simpler classifiers, making them less conservative, but do not show significant effects on more complex classifiers.
更多查看译文
关键词
exoplanet transit identification,signal-to-noise
AI 理解论文
溯源树
样例
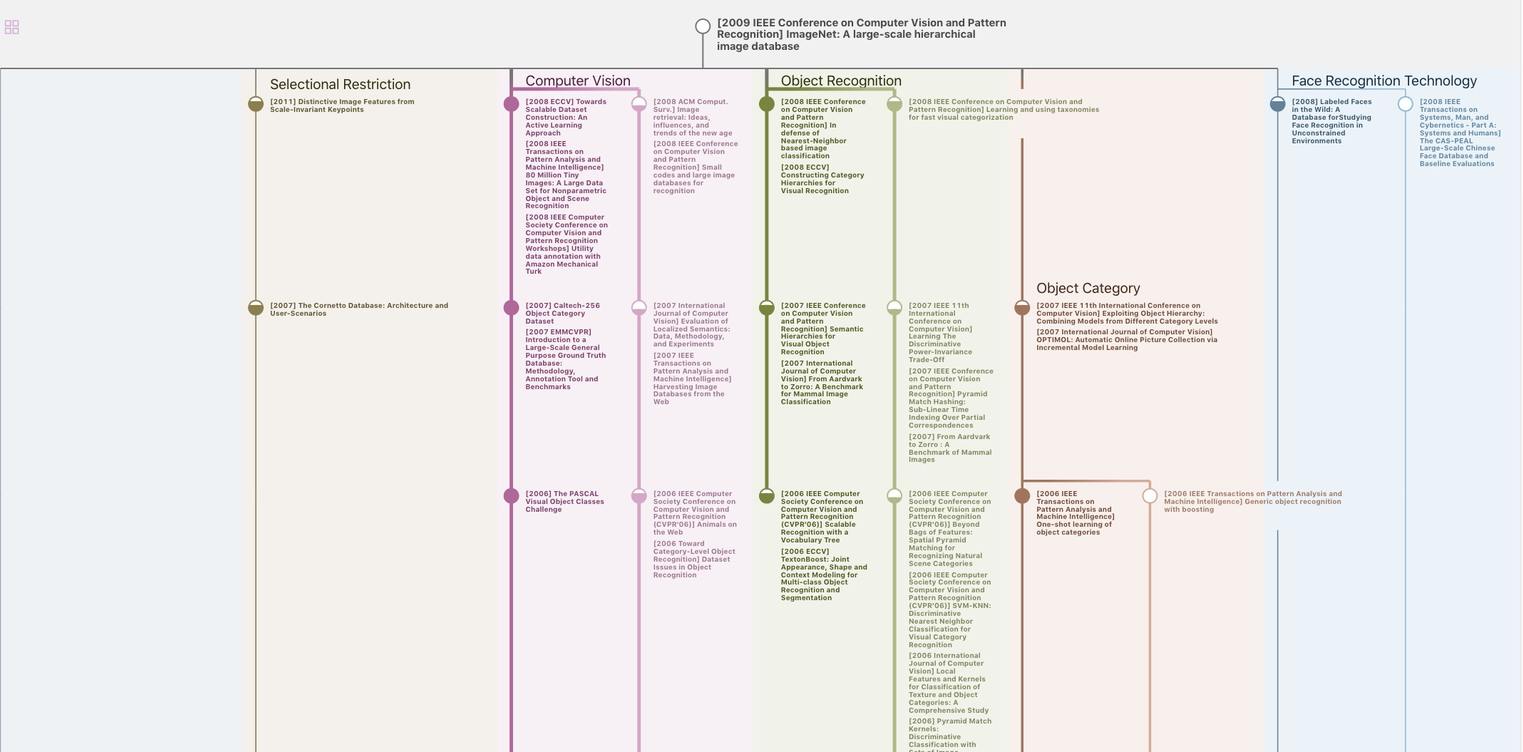
生成溯源树,研究论文发展脉络
Chat Paper
正在生成论文摘要