Exponential Convergence of Infeasibility Proofs for Kinematic Motion Planning.
WAFR(2022)
摘要
Proving motion planning infeasibility is an important part of a complete motion planner. Common approaches for high-dimensional motion planning are only probabilistically complete. Previously, we presented an algorithm to construct infeasibility proofs by applying machine learning to sampled configurations from a bidirectional sampling-based planner. In this work, we prove that the learned manifold converges to an infeasibility proof exponentially. Combining prior approaches for sampling-based planning and our converging infeasibility proofs, we propose the term asymptotic completeness to describe the property of returning a plan or infeasibility proof in the limit. We compare the empirical convergence of different sampling strategies to validate our analysis.
更多查看译文
关键词
kinematic motion planning,motion planning,infeasibility proofs
AI 理解论文
溯源树
样例
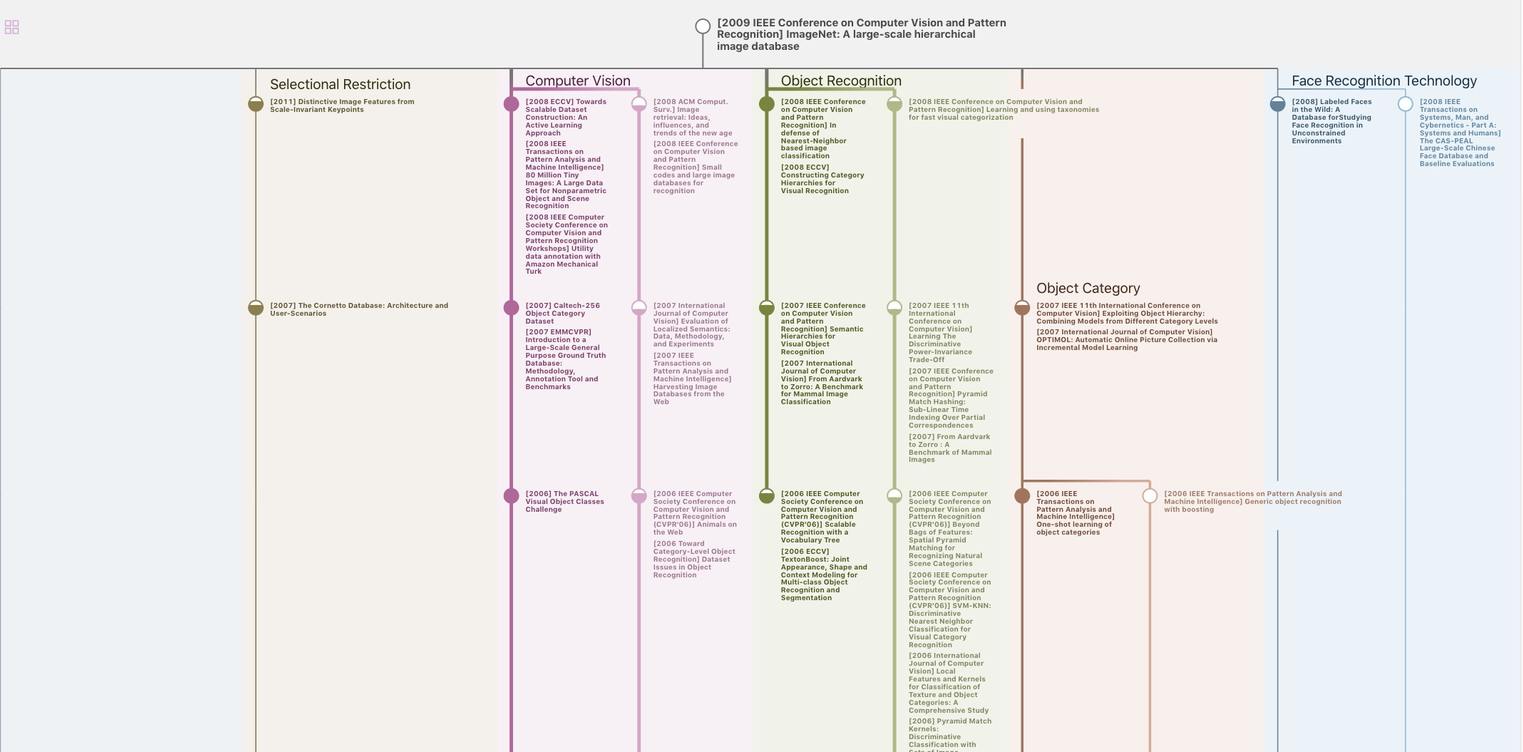
生成溯源树,研究论文发展脉络
Chat Paper
正在生成论文摘要