Dynamic Clustering Federated Learning for Non-IID Data.
CICAI (3)(2022)
摘要
Federated learning (FL) aims to raise a multi-client collaboration approach in the case of local data isolation. In particular, the clients with non-IID data frequently participate in or leave the federated learning training process asynchronously, resulting in dynamic federated learning (DFL) scenario, which attracts more and more attention. Indeed, an effective DFL solution has to address the following two challenges: 1) Statistical Dynamics. The distributions of local data from clients are always non-IID and the global data distribution is dynamic due to the participation or departure of clients. 2) Expiration Dynamics. After clients leave the federated training process, their historical updated models have a certain validity to reuse in subsequent rounds but it is hard to quantify this validity. In this paper, we first consider clustering the clients with similar data distribution to make them much closer to IID and concentrating on the training the models in each cluster. Then we analyze the changing trend of model validity named model quality and define one suitable function to describe expiration dynamics. As a solution, we propose Dynamic Clustering Federated Learning (DCFL) framework to improve federated learning on non-IID data in DFL. Specifically, DCFL follows the client-server architecture as the standard FL. On the client side, the local devices calculate the related information of the local data distribution for client clustering. On the server side, we design two strategies for the challenges above. We propose dynamic clustering aggregation strategy (including a dynamic clustering algorithm and a two-stage aggregation) by dynamically clustering clients and then aggregating the local models to overcome Statistical Dynamics. Besides, we propose expiration memory strategy by reusing the historical models and then adjusting to model quality of historical models as the basis for model aggregation to overcome Expiration Dynamics. Finally, we conduct extensive experiments on public datasets, which demonstrate the effectiveness of the DCFL framework.
更多查看译文
关键词
Federated learning, Dynamics, Clustering, Non-IID data
AI 理解论文
溯源树
样例
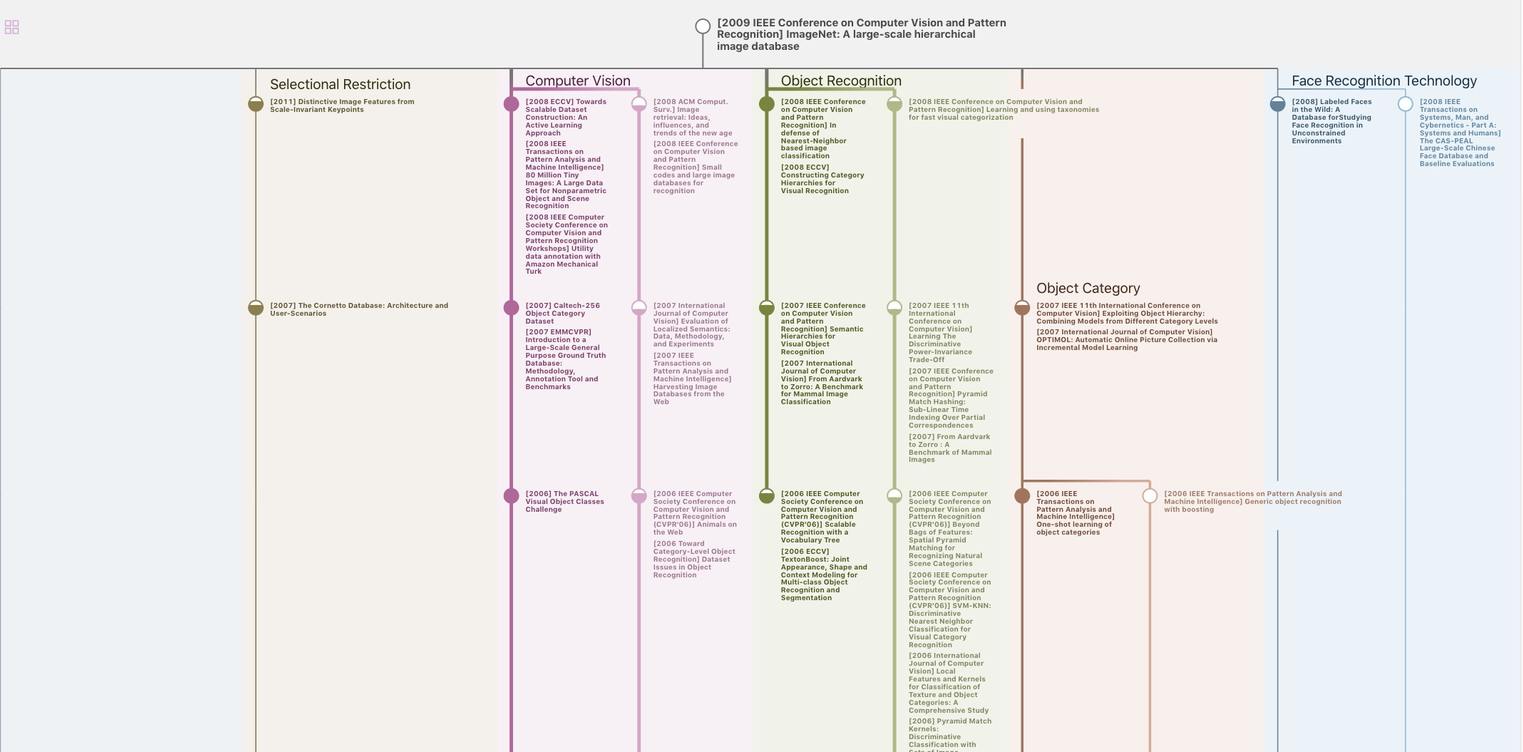
生成溯源树,研究论文发展脉络
Chat Paper
正在生成论文摘要