A General Multi-System Learning Control Framework and its Application to Non-Synchronized Experiments.
CCTA(2022)
摘要
In this paper we present a framework for extending iterative learning control (ILC) to the case of multiple systems learning together. We build on an existing single-system ILC framework that uses a two step approach, wherein first a model is updated from observations, including a correction model explaining deviations compared to the expectations, which is afterwards used to update the control signals to apply. In this paper we will present methods that adapt the first step, so that for updating the models information from multiple systems will be used. This is done by composing the models as a part that is common over all systems, and another delta part that is different for each system and describes the deviation to the common model. The presented methods are positioned in a novel bigger framework, and an extension specifically oriented towards systems not operating at the same time or frequency is proposed. The proposed method has been experimentally validated on 3 similar but non-identical slider-crank setups, wherein the systems perform experiments one after the other in varied sequences. By sharing information, the time needed for each system to find good outcomes is reduced, and especially for systems rarely performing experiments the gain was significant. Over 11 randomly chosen sequences of experiments, we save 37% of iterations on average w.r.t. single-system ILC.
更多查看译文
关键词
bigger framework,common model,control signals,correction model,general multisystem learning control framework,iterative learning control,nonidentical slider-crank setups,nonsynchronized experiments,single-system ILC framework
AI 理解论文
溯源树
样例
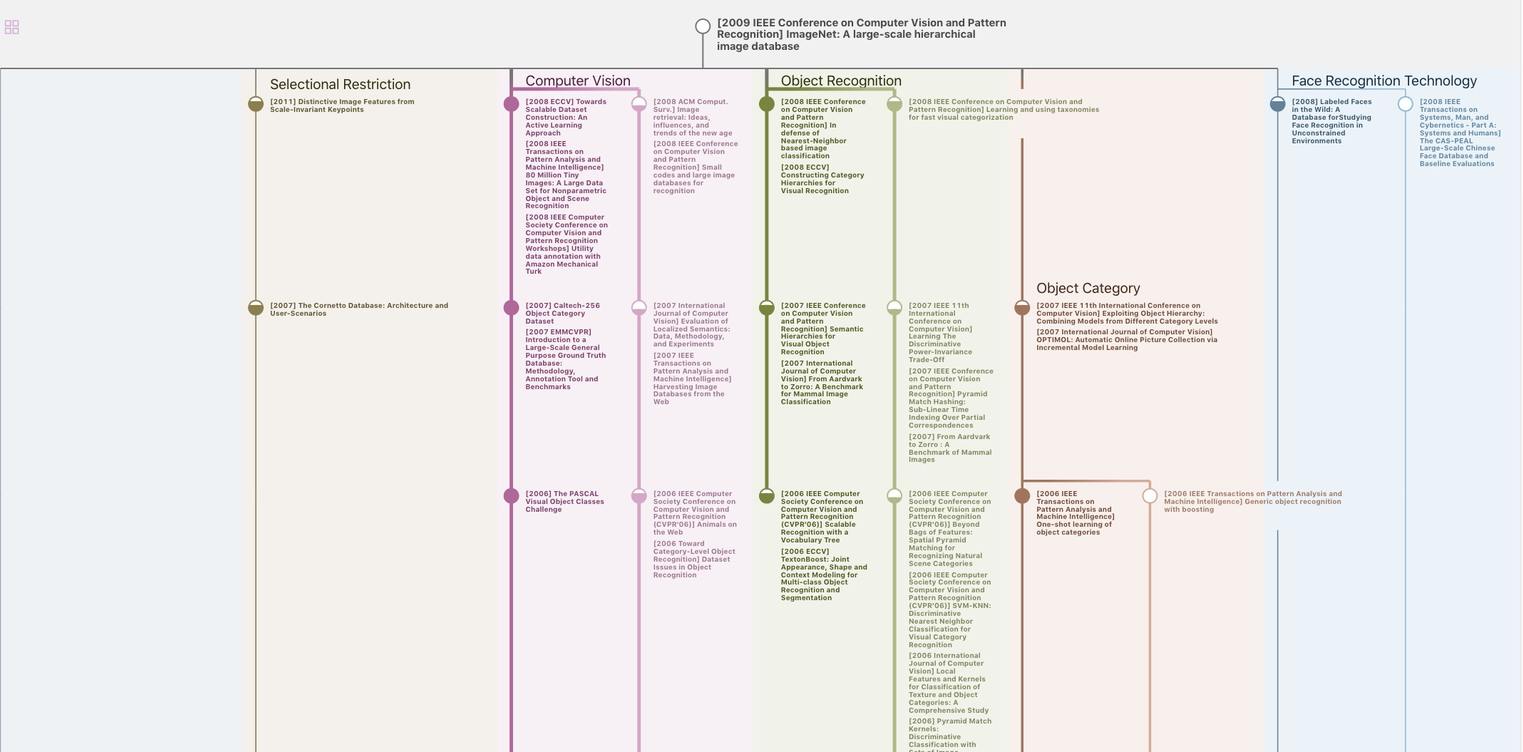
生成溯源树,研究论文发展脉络
Chat Paper
正在生成论文摘要