ColorWater: A Diverse Dataset and Benchmark for Semantic Water Surface Understanding.
ICPR(2022)
摘要
Intelligent water environment monitoring has received global attention. Providing researchers with a water surface dataset with sufficient data and diversity is an important support for training and evaluating the water surface understanding model. However, current water surface datasets lack high-quality and rich annotations of various floating objects on real-world water surfaces, resulting in a partial understanding of the water surface by existing intelligent monitoring methods of the water environment. To address this, we present ColorWater, a diverse semantic segmentation dataset tailored for comprehensively understanding the complex water surface. ColorWater contains 1279 complex water images and pixel-level annotations consisting of 9 categories and 10942 objects and stuff. Our effort exceeds previous datasets in terms of annotation richness and scene complexity. In ColorWater, there exists scale diversity in which the small objects are the majority. Thus, we propose a new global evaluation metric, SWIoU (Scale Weight IoU), to improve the sensitivity of the global metric for the segmentation of different-scale objects and reasonably evaluate the global performance of semantic water surface segmentation models. We further provide an in-depth analysis of annotation inconsistency and dataset's characteristics. Moreover, we also build a benchmark evaluation of semantic segmentation methods in the Color Water. Our dataset is publicly available at https://github.com/L-cuixiao/ColorWater.
更多查看译文
AI 理解论文
溯源树
样例
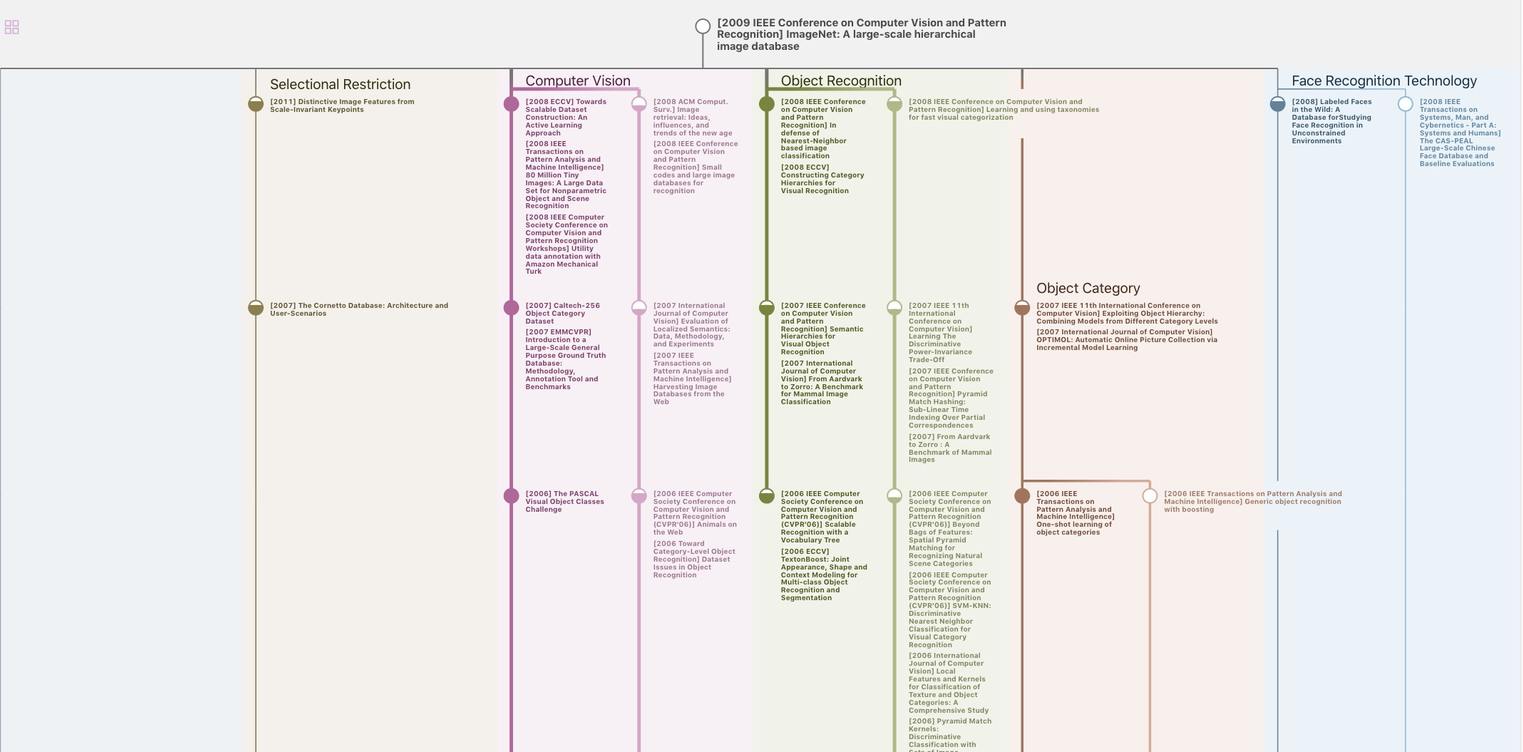
生成溯源树,研究论文发展脉络
Chat Paper
正在生成论文摘要