Audio and Video-based Emotion Recognition using Multimodal Transformers.
ICPR(2022)
摘要
Emotion recognition, an important research problem in human-robot interactions, is primarily achieved by extracting human emotions from audio and visual data. State-of-the-art performance is reported by audio-visual sensor fusion algorithms using deep learning models such as CNN, RNN, and LSTM. However, the RNN and LSTM are shown to be limited in handling the long-term dependencies over the entire input sequence. In this work, we propose to improve the performance of audio-visual emotion recognition using a novel transformer-based model, containing three transformer branches, named multimodal transformers. The three transformer branches, in our work, compute the audio self-attention, the video self-attention, and the audio-video cross attention. The self-attention branches identify the most relevant information in the audio and video input, while the cross-attention branch identifies the most relevant audio-video interactive information. The relevant information from these three branches report the best performance in our ablation study. We also propose a novel temporal embedding scheme, termed block embedding, to add the temporal information to the visual feature, derived from the multiple frames in the video. The proposed architecture is validated using the RAVDESS, CREMA-D, and SAVEE audio-visual public datasets. A detailed ablation study and comparative analysis with baseline models is performed. The results show that the proposed multi-modal transformer framework is better than the baseline methods.
更多查看译文
关键词
emotion recognition,audio,video-based
AI 理解论文
溯源树
样例
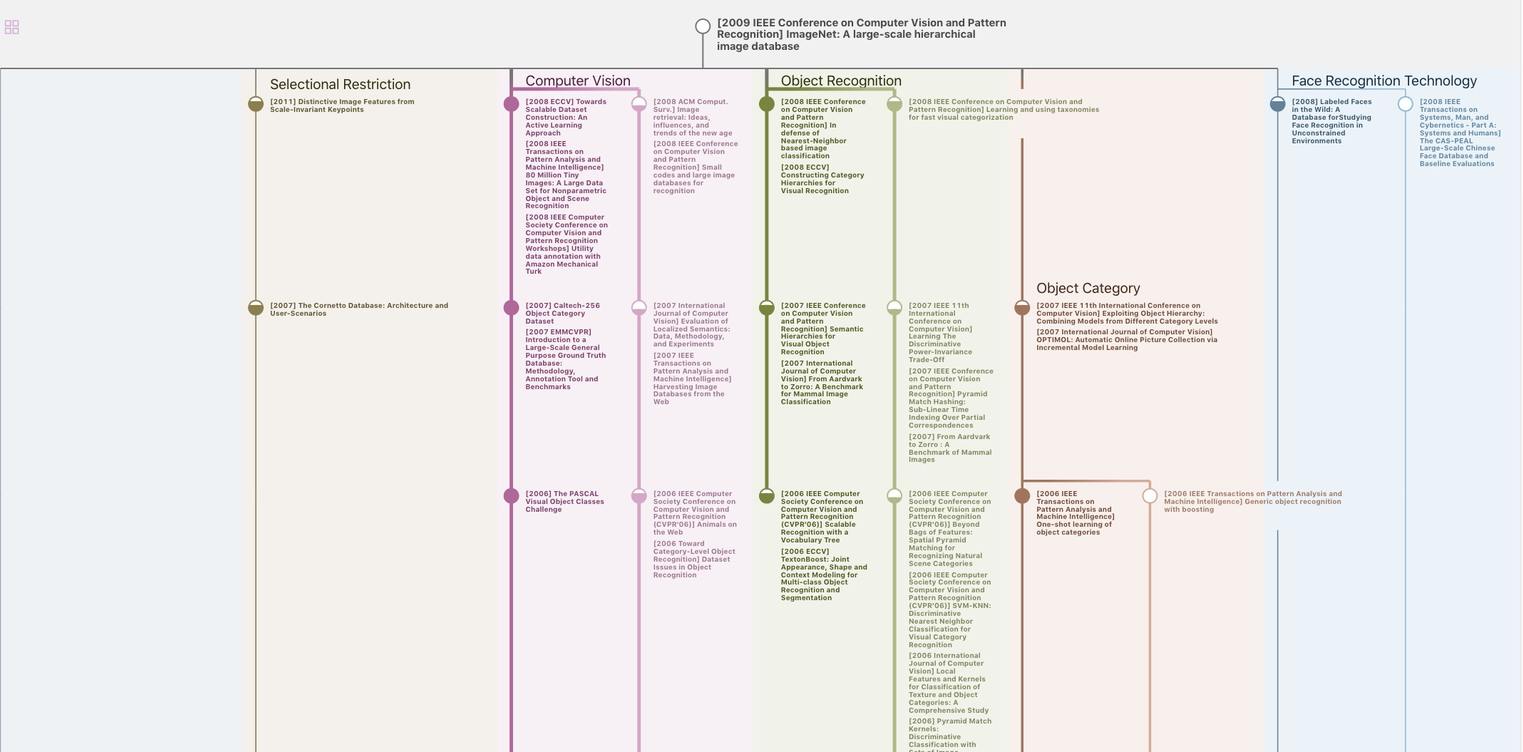
生成溯源树,研究论文发展脉络
Chat Paper
正在生成论文摘要