A Graph Convolutional Network with Early Attention Module for Skeleton-based Action Prediction.
2022 26th International Conference on Pattern Recognition (ICPR)(2022)
摘要
This paper addresses the problem of skeleton-based action prediction, which aims to predict the action label when the skeleton sequence is partially observed. The action prediction task is more challenging compared to the after-the-fact action recognition since it needs to make decisions according to the beginning part of action executions. The existing methods improve the action prediction performance by taking advantage of the global action knowledge in full sequences, and some of them require the correspondence between a partial sequence and its associated full sequence. In this paper, we step towards a new direction by exploiting the discriminative information in early observations of actions as much as possible. We propose a Graph Convolutional Network with Early Attention Module (GCN-EAM), which employs a series of spatial-temporal graph convolution blocks to extract features from skeletons. In order to infer the action category as fast as possible, we introduce an early attention module to adaptively emphasize discriminative observations at the beginning stage of actions. The proposed method is evaluated on the large-scale NTU-RGB+D dataset and achieves excellent performance for action prediction.
更多查看译文
关键词
action category,action executions,action label,action prediction performance,action prediction task,after-the-fact action recognition,early attention module,GCN-EAM,global action knowledge,Graph Convolutional Network,partial sequence,skeleton sequence,skeleton-based action prediction,spatial-temporal graph convolution blocks
AI 理解论文
溯源树
样例
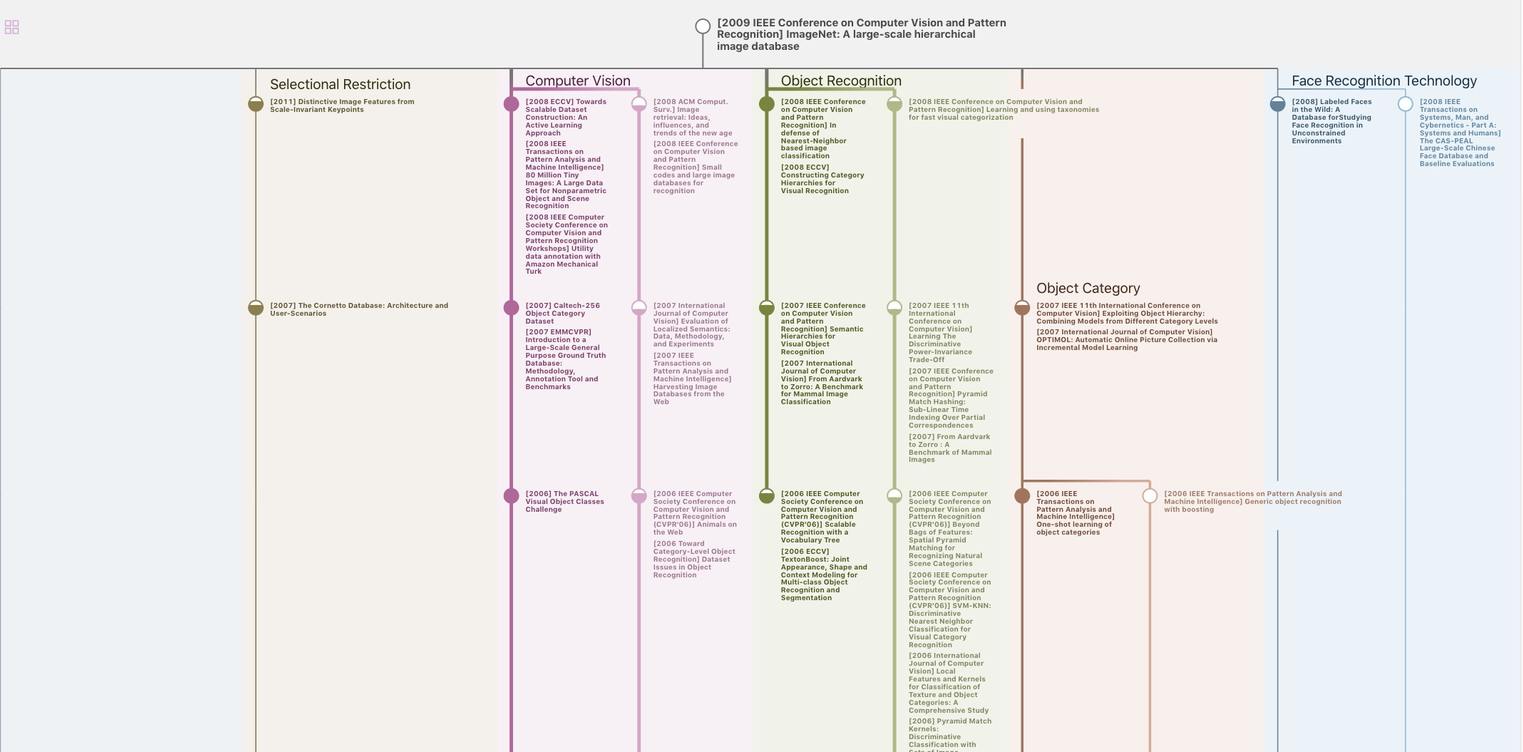
生成溯源树,研究论文发展脉络
Chat Paper
正在生成论文摘要